Retinal Glaucoma Detection Using Deep Learning Algorithm
DOI:
https://doi.org/10.18201/ijisae.2022.267Keywords:
Curvelet;, Discrete fuzzy Glaucoma, Pre-processing, removal, segmentation, wavelet transformAbstract
Glaucomatic retinopathy is a degenerative eye disease that is assessed as it progresses, making it necessary to examine it more frequently. The lack of professional observers has led to computer-aided monitoring. A novel method of analyzing retinal images by detecting vessels and exudates has been proposed to analyze retinal vascular disorders. A curvelet-based method improves the retinal image to allow for better vessel detection. The discrete curvelet transform (DCT) is applied, and its coefficients are applied to obtain the image ridges using multi-structure elements. With connected component analysis (CCA) and length filtering, false edges are removed. In this work, noise removal is modified by a suitable nonlinear function. The modified function parameters are derived by using fast DCT (FDCT) coefficients, which enhance weak edges while eliminating noise. Mathematical morphogen plane process using Discrete Wavelet Transform, Curvelet Transform, Orthogonal Transform, and Fuzzy Segmentation on a minimum of 10 features such as Mean, Variance, Entropy with the data set trained images on 15 images using NN (neural network) training is implemented and NN classifier based Normal or Abnormal is applied. Simulation using MATLAB Simulink is done and comparisons among Discrete Wavelet, Curvelet, Orthogonal transform, and fuzzy segmentation are executed and the blood vessels segmentation resulted in promising results.
Downloads
References
[2] A. M. Mendonc¸a and A. Campilho, “Segmentation of retinal blood vessels by combining the detection of centrelines and morphological reconstruction,” IEEE Trans. Med. Imag., vol. 25, no. 9, pp. 1200–1213,
[3] M. E. Martinez-Perez, A. D. Hughes, S. A. Thom, and K. H. Parker, “Improvement of a retinal blood vessel segmentation method using the insight segmentation and registration toolkit (ITK),” in Proc. IEEE 29th Annu. Int. Conf. EMBS. Lyon, IA, France, 2007, pp. 892–895.
[4] M. Lalonde, M. Beaulieu, and L. Gagnon, “Fast and robust optic disc detection using pyramidal decomposition and Hausdorff-based template matching,” IEEE Trans. Med. Imag., vol. 20, no. 11, pp. 1193–1200, Nov. 2001Sep. 2008.
[5] Youssif, A.A.-H.A.-R., Ghalwash, A.Z., Ghoneim, A.A.S.A.-R.: Optic disc detection from normalized digital fundus images employing a vessels' direction matched filter. IEEE Transactions on Medical Imaging 27, 11–18 (2008)
[6] T. Kauppi and H. Kälviäinen, “Simple and robust optic disc localization using the decorrelated templates,” in Proc. 10th Int. Conf. Advanced Concepts for Intel. Vision Syst., Berlin, Germany: Springer-Verlag, 2008, pp. 719–729.
[7] Banerjee, B., Bhattacharjee, T., Chowdhury, N.: Colour Image Segmentation Technique Using Natural Grouping of Pixels. International Journal of Image Processing (IJIP) 4(4), 320–328 (2010)
[8] Mohamed Saleem TS, Jain A, Tarani P, Ravi V, Gauthaman K. "Aliskiren: A Novel, Orally Active Renin Inhibitor." Systematic Reviews in Pharmacy 1.1 (2010), 93-98. Print. doi:10.4103/0975-8453.59518
[9] Rajendra Acharya, et al, (2011). Automated Diagnosis of Glaucoma Using Texture and Higher-Order Spectral Features. IEEE Transactions on information technology in biomedicine. 15(3):449-455.
[10] Jyotiprava Dash and Nilamani Bhoi. A thresholding-based technique to extract retinal blood vessels from fundus images. Future Computing and Informatics Journal, 2(2):103–109, 2017.
[11] Huazhu Fu, Jun Cheng and Yanwu Xu. Disc-aware Ensemble Network for Glaucoma Screening from Fundus Image. In 2017 an IEEE transaction Journal on medical imaging, 2017.
[12] Alreja, G., Lotfi, A. Eustachian valve endocarditis: Rare case reports and review of the literature (2011) Journal of Cardiovascular Disease Research, 2 (3), pp. 181-185. DOI: 10.4103/0975-3583.85266
[13] Ganesh E., Member, IEEE, Shanker N.R., and Priya.M. Non-Invasive measurement of glaucoma disease at an earlier stage through GMR sensor AH bio-magnetic signal from the eye and RAWDT algorithm. In 2018, an IEEE journal, 2018.
[14]. S. S. Kanse and D. M. Yadav. Retinal fundus image for glaucoma detection: A review and study. Journal of Intelligent Systems, 28(1):43– 56, 2017.
[15] Bhupendra Singh Kirar, Dheeraj Kumar Agrawal. Computer-aided diagnosis of glaucoma using discrete and empirical wavelet transform from fundus images. In 2018 an IET image process journal at Maulana Azad National Institute of Technology, Bhopal, India, 2018.
[16] Diaz-Pinto, A. Morales, S., Naranjo, V. et al. CNNs for automatic glaucoma assessment using fundus images: an extensive validation. BioMed Eng Online 18, 29 (2019)
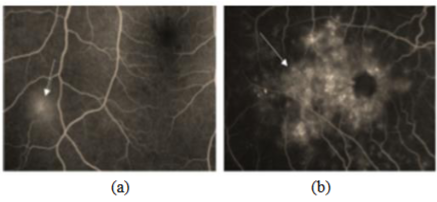
Downloads
Published
How to Cite
Issue
Section
License
Copyright (c) 2022 Tanya Maurya, Lalitha Kala, Kaveti Manasa, Kanimozhi Gunasekaran, Umayal C

This work is licensed under a Creative Commons Attribution-ShareAlike 4.0 International License.
All papers should be submitted electronically. All submitted manuscripts must be original work that is not under submission at another journal or under consideration for publication in another form, such as a monograph or chapter of a book. Authors of submitted papers are obligated not to submit their paper for publication elsewhere until an editorial decision is rendered on their submission. Further, authors of accepted papers are prohibited from publishing the results in other publications that appear before the paper is published in the Journal unless they receive approval for doing so from the Editor-In-Chief.
IJISAE open access articles are licensed under a Creative Commons Attribution-ShareAlike 4.0 International License. This license lets the audience to give appropriate credit, provide a link to the license, and indicate if changes were made and if they remix, transform, or build upon the material, they must distribute contributions under the same license as the original.