Predicting CT Images with Attenuation Correction Factor for Using Neighbourhood Approach
Keywords:
PET, CT, MRI, KNN, Attenuation, PCTAbstract
Magnetic Resonance Imaging (MRI) and Computed Tomography (CT) imaging were incorporated and correlated with each other. These two clinical were adjusted with the attenuation factor between them. As both the clinical imaging techniques use the same hardware leads to time consumption with a low rate of prediction in the existing systems. Therefore, in this paper, predicting CT (p-CT) images by accessing MRI data was implemented with nearest neighbor cluster method identification. The proposed model reduces the training cost and enhances the prediction rate by minimizing the time of execution. PET/MRI helps in understanding the regions of soft tissue and functional tissues, which includes the internal body parts. These datasets out to investigate scientific prerequisites that would perchance be acquired from brain PET/MRI imaging mainly primarily based absolutely on caseload. In this paper, the performance metrics compared are Peak Signal Noise Ratio (PSNR), Mean Absolute Error (MAE). The performance of PET/MRI to quit the imaging modality wish to investigate neurologic and oncologic stipulations related to handy tissues is highlighted. Clinical factors of PET/MRI and its software program to scientific conditions are illustrated with examples extracted from the authors' prior experience.
Downloads
References
L. G. Strauss and P. S. Conti, ‘‘The applications of PET in clinical oncology,’’ J. Nucl. Med. 32, 623–648.
Nouby M. Ghazaly, M. M. A. . (2022). A Review on Engine Fault Diagnosis through Vibration Analysis . International Journal on Recent Technologies in Mechanical and Electrical Engineering, 9(2), 01–06. https://doi.org/10.17762/ijrmee.v9i2.364
Brenner DJ, Hall EJ. Computed Tomography — An Increasing Source of Radiation Exposure. New England Journal of Medicine. 2007;357(22):2277–2284.
Zaidi H, Montandon ML, Slosman DO. Magnetic resonance imaging-guided attenuation and scatter corrections in three-dimensional brain positron emission tomography. Medical Physics. 2003;30(5):937–948.
Hsu S-H, et al. Investigation of a method for generating synthetic CT models from MRI scans of the head and neck for radiation therapy. Physics in medicine and biology. 2013;58(23) doi: 10.1088/0031-9155/58/23/8419.
Berker Y, et al. MRI-Based Attenuation Correction for Hybrid PET/MRI Systems: A 4-Class Tissue Segmentation Technique Using a Combined Ultrashort-Echo-Time/Dixon MRI Sequence. Journal of Nuclear Medicine. 2012;53(5):796–804.
Kops ER, Herzog H. Alternative methods for attenuation correction for PET images in MR-PET scanners. Nuclear Science Symposium Conference Record, 2007. NSS ‘07. IEEE; 2007.
Yu Gong1,3, Hongming Shan2, Yueyang Teng∗1, Hairong Zheng 3, Ge Wang2, Shanshan Wang∗3, LOW-DOSE PET IMAGE RESTORATION WITH 2D AND 3D NETWORK PRIOR LEARNING, 978-1-7281-7401-3/20/$31.00 ©2020 IEEE
Lijun Zhao, Zixiao Lu, Jun Jiang, Yujia Zhou, Yi Wu, and Qianjin Feng, “Automatic nasopharyngeal carcinoma segmentation using fully convolutional networks with auxiliary paths on dual-modality pet-ct images,” Journal of digital imaging, vol. 32, no. 3, pp. 462–470, 2019.
Andersen FL, Ladefoged CN, Beyer T, et al. Combined PET/MR imaging in neurology: MR-based attenuation correction implies a strong spatial bias when ignoring bone. Neuroimage. 2014;84: 206–216.
Kadhim, R. R., and M. Y. Kamil. “Evaluation of Machine Learning Models for Breast Cancer Diagnosis Via Histogram of Oriented Gradients Method and Histopathology Images”. International Journal on Recent and Innovation Trends in Computing and Communication, vol. 10, no. 4, Apr. 2022, pp. 36-42, doi:10.17762/ijritcc.v10i4.5532.
Roy S, Carass A, Jog A, et al. MR to CT registration of brains using image synthesis. Proc SPIE. March 21, 2014:9034.
Keller, S.H., Holm, S., Hansen, A.E., Sattler, B., Andersen, F., Klausen, T.L., Højgaard, L., Kjær, A., Beyer, T., 2013. Image artifacts from MR-based attenuation correction in clinical, whole-body PET/MRI. MAGMA 26 (1), 173–181.
A. Jog, S. Roy, A. Carass, and J. L. Prince, “Magnetic Resonance Image Synthesis through Patch Regression,” in Proc. of the Int. Symposium on Biomed. Imag. (ISBI 2013), 2013, pp. 350–353.
L. N. Balai, G. K. J. A. K. S. (2022). Investigations on PAPR and SER Performance Analysis of OFDMA and SCFDMA under Different Channels. International Journal on Recent Technologies in Mechanical and Electrical Engineering, 9(5), 28–35. https://doi.org/10.17762/ijrmee.v9i5.371
Li R, et al. Deep Learning Based Imaging Data Completion for Improved Brain Disease Diagnosis. In: Golland P, et al., editors. Medical Image Computing and Computer-Assisted Intervention – MICCAI 2014. Springer International Publishing; 2014. pp. 305–312.
Jung JH, Choi Y, Jung J, Kim S, Lim HK, Im KC, et al. Development of PET/MRI with insertable PET for simultaneous PET and MR imaging of human brain. Med Phys. 2015;42:2354– 63.
Roy S, Carass A, Prince JL. Magnetic Resonance Image Example-Based Contrast Synthesis. Medical Imaging, IEEE Transactions on. 2013;32(12):2348–2363.
C. Rorden, L. Bonilha, J. Fridriksson, B. Bender, and H. Karnath, “Agespecific CT and MRI templates for spatial normalization,” NeuroImage, vol. 61, no. 4, pp. 957–966, 2012.
Ranadev, M. B. ., V. R. . Sheelavant, and R. L. . Chakrasali. “Predetermination of Performance Parameters of 3-Phase Induction Motor Using Numerical Technique Tools”. International Journal on Recent and Innovation Trends in Computing and Communication, vol. 10, no. 6, June 2022, pp. 63-69, doi:10.17762/ijritcc.v10i6.5628.
Ye D, et al. Modality Propagation: Coherent Synthesis of Subject-Specific Scans with Data-Driven Regularization. In: Mori K, et al., editors. Medical Image Computing and Computer-Assisted Intervention – MICCAI 2013. Springer; Berlin Heidelberg: 2013. pp. 606–613.
Kabisha, M. S., Rahim, K. A., Khaliluzzaman, M., & Khan, S. I. (2022). Face and Hand Gesture Recognition Based Person Identification System using Convolutional Neural Network. International Journal of Intelligent Systems and Applications in Engineering, 10(1), 105–115. https://doi.org/10.18201/ijisae.2022.273
Cao, T., Zach, C., Modla, S., Powell, D., Czymmek, K., Niethammer, M.: Registration for Correlative Microscopy Using Image Analogies. In: Dawant, B.M., Christensen, G.E., Fitzpatrick, J.M., Rueckert, D. (eds.) WBIR 2012. LNCS, vol. 7359, pp. 296–306. Springer, Heidelberg (2012)
Chaudhary, D. S. . (2022). Analysis of Concept of Big Data Process, Strategies, Adoption and Implementation. International Journal on Future Revolution in Computer Science &Amp; Communication Engineering, 8(1), 05–08. https://doi.org/10.17762/ijfrcsce.v8i1.2065
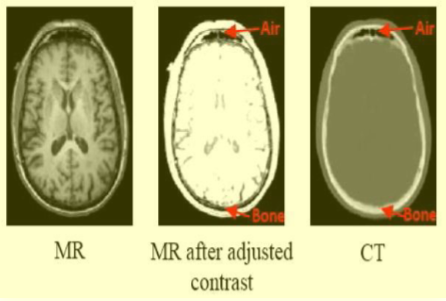
Downloads
Published
How to Cite
Issue
Section
License

This work is licensed under a Creative Commons Attribution-ShareAlike 4.0 International License.
All papers should be submitted electronically. All submitted manuscripts must be original work that is not under submission at another journal or under consideration for publication in another form, such as a monograph or chapter of a book. Authors of submitted papers are obligated not to submit their paper for publication elsewhere until an editorial decision is rendered on their submission. Further, authors of accepted papers are prohibited from publishing the results in other publications that appear before the paper is published in the Journal unless they receive approval for doing so from the Editor-In-Chief.
IJISAE open access articles are licensed under a Creative Commons Attribution-ShareAlike 4.0 International License. This license lets the audience to give appropriate credit, provide a link to the license, and indicate if changes were made and if they remix, transform, or build upon the material, they must distribute contributions under the same license as the original.