NNLGBM: Medical Image Classification through Secure Collaboration in Pneumonia Detection by Blending NN and LGBM
Keywords:
Segmentation, Deep learning neural network, LGBM, Pneumonia, Watermarking, SecurityAbstract
The amount of digital photos being transmitted over computer networks is increasing at an alarming rate. For a variety of reasons, including confidentiality, authenticity, and reliability, the safeguarding of digital data, particularly medical imaging, becomes increasingly critical. Digital watermarking has swiftly gained popularity as a cutting-edge technology for enhancing the security of digital photographs, and it is becoming increasingly popular. The incorporation of a watermark onto a medical image can serve to authenticate and ensure the integrity of the image. Pneumonia is responsible for the deaths of over 700,000 youngsters per year and affects approximately 7% of the world's population. When it comes to diagnosing this disease, chest X-rays are the primary tool. Examining chest X-rays, on the other hand, is a difficult assignment for even the most experienced radiologist. There is an urgent need to improve the precision with which diagnoses are made. Using computerized chest X-ray images, we present an appropriate method for the clinical condition that can be utilized to guide clinicians through the judgment process. A unique strategy based on a combination of Deep learning neural networks and LGBM in the most efficient manner is presented. A supervised learning strategy is applied in this case, in which the network anticipates the outcome depend on the merits of the data set that was used to train the network. Data augmentation approaches that enhance the training database in a balanced manner are used to increase the training dataset. The suggested classifier outperforms all of the individual models in terms of accuracy. Final assessment is made not just in terms of test accuracy but also in terms of the AUC score, which measures the overall effectiveness of the model. On a chest X-ray dataset, the final proposed weighted classifier model achieves a test accuracy of 99.43 percent and an AUC score of 99.76 percent, demonstrating superior performance. The developed framework can therefore be utilized to make a rapid diagnosis of pneumonia and to assist physicians in their diagnostic procedure.
Downloads
References
UNICEF. Available online:
https://data.unicef.org/topic/child-health/pneumonia/
Tilve A., Nayak S., Vernekar S., Turi D., Shetgaonkar P.R., Aswale S., Pneumonia Detection Using Deep Learning Approaches. In Proceedings of the 2020 International Conference on Emerging Trends in Information Technology and Engineering (ic-ETITE),2020, Vellore, India, 24, p. 1–8.
Garg, D. K. . (2022). Understanding the Purpose of Object Detection, Models to Detect Objects, Application Use and Benefits. International Journal on Future Revolution in Computer Science &Amp; Communication Engineering, 8(2), 01–04. https://doi.org/10.17762/ijfrcsce.v8i2.2066
Periselneris N.J.; Brown, S.J.; José, R.J. (2020): Pneumonia. Availableonline:https://www.medicinejournal.co.uk/article/S1357-3039 (20)30049-9/fulltext.
Sethi R.,Mehrotra M. Sethi D.,Deep Learning based Diagnosis Recommendations for COVID-19 Using Chest X-rays Images. 2020 Second International Conference on Inventive Research in Computing Applications (ICIRCA), 2020, Coimbatore, India, pp.1-4, doi:10.1109/ICIRCA48905.2020.918327.
Swapna G.,Vinayakumar R.,Soman K.P., Diabetes Detection using Deep Learning Algorithms, ICT Express, 2008 4(4), p. 243-246.
Garg, D. K. . (2022). Understanding the Purpose of Object Detection, Models to Detect Objects, Application Use and Benefits. International Journal on Future Revolution in Computer Science &Amp; Communication Engineering, 8(2), 01–04. https://doi.org/10.17762/ijfrcsce.v8i2.2066
Pant A., A. Jain A., Nayak K. C., Gandhi D.,Prasad B. G.,Pneumonia Detection: An Efficient Approach Using Deep Learning, 2020, 11th International Conference on Computing, Communication and Networking Technologies (ICCCNT), pp. 1-6, doi: 10.1109/ICCCNT49239.2020.9225543.
Xia T.,Fu Y.Q., Jin N.,Chazot P.,Angeloy P.,Jiang R.,AI-enabled Microscopic Blood Analysis for Microfluidic COVID19Hematology, 2020, 5th International Conference on Computational Intelligence and Applications (ICCIA),Beijing, China, p.98-102, doi:10.1109 /ICCIA49625. 2020.00026.
Garg, D. K. . (2022). Understanding the Purpose of Object Detection, Models to Detect Objects, Application Use and Benefits. International Journal on Future Revolution in Computer Science &Amp; Communication Engineering, 8(2), 01–04. https://doi.org/10.17762/ijfrcsce.v8i2.2066
Ramwala O.A., MulchandaniH., Dala P., Paunwala M.C., Pinwale C.N.,COVID-19 Diagnosis from Chest Radiography ImagesUsing Deep Residual Network, 2020, 11th International Conference on Computing, Communication and Networking Technologies (ICCCNT), Kharagpur, India.
Garg, D. K. . (2022). Understanding the Purpose of Object Detection, Models to Detect Objects, Application Use and Benefits. International Journal on Future Revolution in Computer Science &Amp; Communication Engineering, 8(2), 01–04. https://doi.org/10.17762/ijfrcsce.v8i2.2066
Xu L., MaoX.,Sun M., Liu W., Wang Y.,Tang Y.,Lung lesions detection from CT images based on the modified Faster R-CNN, 2020, International Conference on Computer, Information and Telecommunication Systems (CITS), Hangzhou, China, p.1-5,doi: 10.1109/CITS49457.2020.9232611.
Garg, D. K. . (2022). Understanding the Purpose of Object Detection, Models to Detect Objects, Application Use and Benefits. International Journal on Future Revolution in Computer Science &Amp; Communication Engineering, 8(2), 01–04. https://doi.org/10.17762/ijfrcsce.v8i2.2066
Ravita, R., & Rathi, S. (2022). Inductive Learning Approach in Job Recommendation. International Journal of Intelligent Systems and Applications in Engineering, 10(2), 242–251. Retrieved from https://ijisae.org/index.php/IJISAE/article/view/1829
Müftüoğlu Z., Kizrak M.A., Yildlnm T., Differential privacy practice on diagnosis of covid-19 radiology imaging using efficientnet, 2020 August, In 2020 International Conference on INnovations in Intelligent SysTems and Applications (INISTA) (pp. 1-6). IEEE.
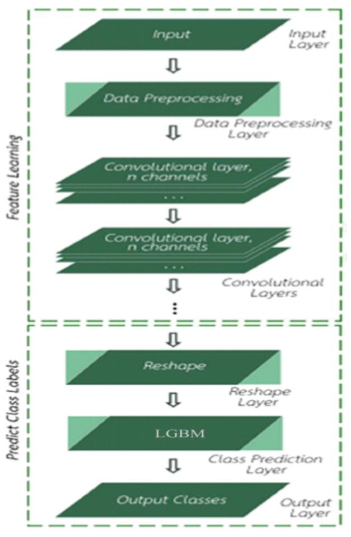
Downloads
Published
How to Cite
Issue
Section
License

This work is licensed under a Creative Commons Attribution-ShareAlike 4.0 International License.
All papers should be submitted electronically. All submitted manuscripts must be original work that is not under submission at another journal or under consideration for publication in another form, such as a monograph or chapter of a book. Authors of submitted papers are obligated not to submit their paper for publication elsewhere until an editorial decision is rendered on their submission. Further, authors of accepted papers are prohibited from publishing the results in other publications that appear before the paper is published in the Journal unless they receive approval for doing so from the Editor-In-Chief.
IJISAE open access articles are licensed under a Creative Commons Attribution-ShareAlike 4.0 International License. This license lets the audience to give appropriate credit, provide a link to the license, and indicate if changes were made and if they remix, transform, or build upon the material, they must distribute contributions under the same license as the original.