Building a Novel Ensemble Learning – Based Prediction Framework for Diagnosis of Coronary Heart Disease
Keywords:
Heart disease prediction, Deep Residual Network (DRN), Ensemble classifiers, coronary artery diseaseAbstract
The newer technologies such as data mining, machine learning, artificial intelligence and data analytics have revolutionized medical sector in terms of using the existing big data to predict the various patterns emerging from the datasets available in the healthcare repositories. The predictions based on the existing datasets in the healthcare sector have rendered several benefits such as helping clinicians to make accurate and informed decisions while managing the patients’ health leading to better management of patients’ wellbeing and health-care coordination. The millions of people have been affected by the coronary artery disease (CAD). There are several machine learning including ensemble learning approach and deep neural networks-based algorithms have shown promising outcomes in improving prediction accuracy for early diagnosis of CAD. This paper analyses the deep neural network variant DRN, Rider Optimization Algorithm-Neural network (RideNN) and Deep Neural Network-Fuzzy Neural Network (DNFN) with application of ensemble learning method for improvement in the prediction accuracy of CAD. The experimental outcomes showed the proposed ensemble classifier achieved the highest accuracy compared to the other machine learning models.
Downloads
References
C. Beulah Christalin Latha, S. and Carolin Jeeva, “Improving the accuracy of prediction of heart disease risk based on ensemble classification techniques”, Informatics in Medicine Unlocked, vol.16, pp.100203, January 2019.
K. Subhadra, and Vikas B, “Neural network based intelligent system for predicting heart disease”, International Journal of Innovative Technology and Exploring Engineering, vol.8, no.5, pp.484-7, March 2019.
K.Srinivas, Dr.G.Raghavendra Rao, and Dr. A.Govardhan, “Analysis of Coronary Heart Disease and Prediction of Heart Attack in Coal Mining Regions Using Data Mining Techniques”, The 5th International Conference on Computer Science & Education, pp.1344-1349, August 2010.
L. N. Balai, G. K. J. A. K. S. (2022). Investigations on PAPR and SER Performance Analysis of OFDMA and SCFDMA under Different Channels. International Journal on Recent Technologies in Mechanical and Electrical Engineering, 9(5), 28–35. https://doi.org/10.17762/ijrmee.v9i5.371
Stephen F. Weng, Jenna Reps, Joe Kai, Jonathan M. Garibaldi, and Nadeem Qureshi, “Can machine-learning improve cardiovascular risk prediction using routine clinical data?”, vol.12, no.4, pp.e0174944, April 2017.
Resul Das, Ibrahim Turkoglu, and Abdulkadir Sengur, “Effective diagnosis of heart disease through neural networks ensembles”, Expert System with applications, vol.36, no. 4, pp.7675–7680, 2009.
Garg, K. . (2022). Compactness in General Category Theory. International Journal on Recent Trends in Life Science and Mathematics, 9(1), 19–27. https://doi.org/10.17762/ijlsm.v9i1.138
Afef Mdhaffar, Ismael Bouassida Rodriguez, Khalil Charfi, Leila Abid, and Bernd Freisleben, “CEP4HFP: Complex event processing for heart failure prediction”, IEEE transactions on nanobioscience, vol.16, no.8, pp.708-17, November 2017.
Rahul Kumar Sevakula, and Nishchal Kumar Verma,“Assessing Generalization Ability of Majority Vote Point Classifiers”, IEEE Transactions on Neural Networks and Learning Systems, vol.28, no. 12, pp.2985–2997, December 2017.
Sehirli, E., & Alesmaeil, A. (2022). Detecting Face-Touch Hand Moves Using Smartwatch Inertial Sensors and Convolutional Neural Networks. International Journal of Intelligent Systems and Applications in Engineering, 10(1), 122–128. https://doi.org/10.18201/ijisae.2022.275
Hui Li, Yanying Cui, Wenze Li, Yue Shi, Chao Fing, Hongzhi Li, Ting Gao, Lihong Hu, and Yinghua Lu, “Ensemble learning for overall power conversion efficiency of the all-organic dye-sensitized solar cells”, IEEE Access, vol.6, pp.34118-26, June 2018.
Ibomoiye Domor Mienye, Yanxia Sun, and Zenghui Wang, “An improved ensemble learning approach for the prediction of heart disease risk”, Informatics in Medicine Unlocked, vol.20, pp.100402, January 2020.
Deekshith Shetty, Harshavardhan C A, M Jayanth Varma, Shrishail Navi, Mohammed Riyaz Ahmed, “Diving Deep into Deep Learning: History, Evolution, Types and Applications”.
ZRui Zhao, Ruqiang Yan, Zhenghua Chen, Kezhi Mao, Peng Wang, and Robert X. Gao,“Deep learning and its applications to machine health monitoring”, Mechanical Systems and Signal Processing, vol.15, pp.213-37, January 2019.
U Rajendra Acharya, Oliver Faust, Vinitha Sree, Swapna G, Roshan Joy Martis,
Nahrizul Adib Kadri, and Jasjit S Suri, “Linear and nonlinear analysis of normal and CAD-affected heart rate signals”, Computer methods and programs in biomedicine, vol.113, no.1, pp.55-68, January 2014.
Ahmad M. Karim, Mehmet S. Guzel, Mehmet R. Tolun, Hilal Kaya, and Fatih V. Çelebi, “A new framework using deep auto-encoder and energy spectral density for medical waveform data classification and processing”, Biocybernetics and Biomedical Engineering, vol.39, no.1, pp.148-159, 2019.
Rajamhoana SP, Devi CA, Umamaheswari K, Kiruba R, Karunya K, and Deepika R., "Analysis of neural networks based heart disease prediction system", In 2018 11th International Conference on Human System Interaction (HSI), pp.233-239, July 2018.
Chandrika, L., and K. Madhavi. "A Hybrid Framework for Heart Disease Prediction Using Machine Learning Algorithms." In E3S Web of Conferences, vol. 309. EDP Sciences, 2021.
Sally Fouad Shady. (2021). Approaches to Teaching a Biomaterials Laboratory Course Online. Journal of Online Engineering Education, 12(1), 01–05. Retrieved from http://onlineengineeringeducation.com/index.php/joee/article/view/43
Ghosh, Pronab, Sami Azam, Mirjam Jonkman, Asif Karim, FM Javed Mehedi Shamrat, Eva Ignatious, Shahana Shultana, Abhijith Reddy Beeravolu, and Friso De Boer. "Efficient prediction of cardiovascular disease using machine learning algorithms with relief and LASSO feature selection techniques." IEEE Access 9,19304-19326, 2021.
Reddy, N. Satish Chandra, Song Shue Nee, Lim Zhi Min, and Chew Xin Ying. "Classification and feature selection approaches by machine learning techniques: Heart disease prediction." International Journal of Innovative Computing, vol. 9, no. 1, 2019.
Mustafa Jan, Akber A Awan, Muhammad S Khalid, and Salman Nisar, “Ensemble approach for developing a smart heart disease prediction system using classification algorithms”, Research Reports in Clinical Cardiology, vol.n9, no.33, 2018.
Farman Ali, Shaker El-Sappagh, S.M. Riazul Islam, Daehan Kwak, Amjad Ali, Muhammad Imran, and Kyung-Sup Kwak, “A smart healthcare monitoring system for heart disease prediction based on ensemble deep learning and feature fusion”, Information Fusion, vol.63, pp.208-22, November 2020.
Qi Zhenya, and Zuoru Zhang, “A hybrid cost-sensitive ensemble for heart disease prediction”, 2021.
Xiao-Yan Gao, Abdelmegeid Amin Ali, Hassan Shaban Hassan, and Eman M. Anwar, “Improving the Accuracy for Analyzing Heart Diseases Prediction Based on the Ensemble Method”, Complexity, February 2021.
Bayu Adhi Tama, Sun Im, and Seungchul Lee, “Improving an intelligent detection system for coronary heart disease using a two-tier classifier ensemble”, BioMed Research International, vol.2020, April 2020.
Garg, D. K. . (2022). Understanding the Purpose of Object Detection, Models to Detect Objects, Application Use and Benefits. International Journal on Future Revolution in Computer Science &Amp; Communication Engineering, 8(2), 01–04. https://doi.org/10.17762/ijfrcsce.v8i2.2066
Yaxing Zhao, Limsoon Wong, and Wilson Wen Bin Goh, "How to do quantile normalization correctly for gene expression data analyses", Scientific reports, vol.10, no.1, pp.1-11, 2020.
Patil, P. ., D. D. . Waghole, D. V. . Deshpande, and D. M. . Karykarte. “Sectoring Method for Improving Various QoS Parameters of Wireless Sensor Networks to Improve Lifespan of the Network”. International Journal on Recent and Innovation Trends in Computing and Communication, vol. 10, no. 6, June 2022, pp. 37-43, doi:10.17762/ijritcc.v10i6.5622.
Safdar, Saima, Saad Zafar, Nadeem Zafar, and Naurin Farooq Khan. "Machine learning based decision support systems (DSS) for heart disease diagnosis: a review." Artificial Intelligence Review 50, no. 4 (2018): 597-623.
Zhicong Chen, Yixiang Chen, Lijun Wu, Shuying Cheng, and Peijie Lin, “Deep residual network based fault detection and diagnosis of photovoltaic arrays using current-voltage curves and ambient conditions”, Energy Conversion and Management, vol.198, pp.111793, October 2019.
D. Binu and B. S Kariyappa, “RideNN: A new rider optimization algorithm-based neural network for fault diagnosis in analog circuits”, IEEE Transactions on Instrumentation and Measurement, vol.68, no.1, pp.2-6, May 2018.
Sakeena Javaid, Muhammad Abdullah, Nadeem Javaid, Tanzeela Sultana, Jawad Ahmed, and Norin Abdul Sattar, “Towards Buildings Energy Management: Using Seasonal Schedules Under Time of Use Pricing Tariff via Deep Neuro-Fuzzy Optimizer”, In2019 15th International Wireless Communications & Mobile Computing Conference (IWCMC), pp.1594-1599, June 2019.
K. Divya Vani, "Heart Disease Prediction using Long Short-Term Memory (LSTM) Deep Learning Methodology", vol.2, no.8, pp.1076-1081, 2021.
Muhammad, Yar, Muhammad Tahir, Maqsood Hayat, and Kil To Chong. "Early and accurate detection and diagnosis of heart disease using intelligent computational model." Scientific reports, vol. 10, no. 1, 1-17, 2020.
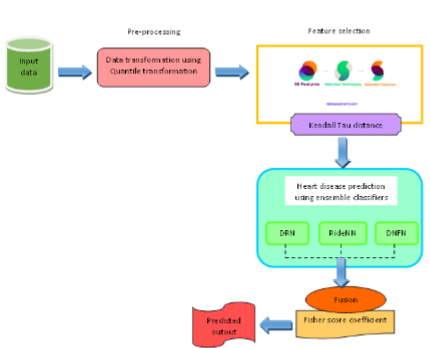
Downloads
Published
How to Cite
Issue
Section
License

This work is licensed under a Creative Commons Attribution-ShareAlike 4.0 International License.
All papers should be submitted electronically. All submitted manuscripts must be original work that is not under submission at another journal or under consideration for publication in another form, such as a monograph or chapter of a book. Authors of submitted papers are obligated not to submit their paper for publication elsewhere until an editorial decision is rendered on their submission. Further, authors of accepted papers are prohibited from publishing the results in other publications that appear before the paper is published in the Journal unless they receive approval for doing so from the Editor-In-Chief.
IJISAE open access articles are licensed under a Creative Commons Attribution-ShareAlike 4.0 International License. This license lets the audience to give appropriate credit, provide a link to the license, and indicate if changes were made and if they remix, transform, or build upon the material, they must distribute contributions under the same license as the original.