Performance Analysis of Poly Cystic Ovary Syndrome (PCOS) using Broyden’s Kernel Import Point (BKIP) Classifier
Keywords:
Broyden, Import Vector Machine, Support Vector Machine, Machine LearningAbstract
Machine Learning (ML) is a progressive and immensely approached technological application which became an enormous trend within the industry. ML is widely utilized in various applications and can be utilized by healthcare companies to acquire valuable data that can be used to diagnose diseases in the earlier stage. In this paper, a new classification approach Broyden’s Kernel Import Point (BKIP) classifier is proposed and summarizes the use and its application in healthcare domain. BKIP classifier is a new approach used for classification which is built on Kernel Logistic Regression and Import Vector Machine (IVM). Data for the current study project was gathered from the ESIC Hospital in Bengaluru, India, and it was found that the BKIP algorithm's computational cost was significantly lower than that of the Support Vector Machine (SVM) and the IVM. The paper provides the implementation of the BKIP classification algorithm and it is noted that when applied to Polycystic Ovary Syndrome (PCOS) data, the model produced an accurate result of 89.1 %.
Downloads
References
Zhu, T. Hastie, Kernel logistic regression and the import vector machine, Comput.Graph. Stat. 14 (2005) 185–205.278R. Roscher et al. / Image and Vision Computing 30 (2012) 263–278.
Y. Tarabalka, M. Fauvel, J. Chanussot, and J. Benediktsson, “SVMand MRF-Based Method for Accurate Classification of Hyperspectral Images,” IEEE Geosci. Remote Sens. Lett., vol. 7, pp. 736–740, 2010.
M. Pal and P. Mather, “Some Issues in the Classification of DAIS Hyperspectral Data,” Int. J. Remote Sens., vol. 27, no. 14, pp. 2895–2916, 2006.
Ehrmann DA: Polycyctic ovary syndrome. N. Engl. J. Med. 352, 1223–1236 (2005).
Professor Cindy Farquhar , Associate Professor Neil Johnson, Dept of Obstetrics and Gynaecology, University of Auckland “Understanding polycystic ovary syndrome"Issue 12 April 2008, ISSN 1177-5645 (Print) ISSN 2253-1947 (Online).
B. Waske, J. Benediktsson, K. Arnason, and J. Sveinsson, “Mapping of Hyperspectral AVIRIS Data using Machine-Learning Algorithms,” Can. J. Remote Sensing, vol. 35, pp. 106–116, 2009.
J. Platt, Advances in Kernel Methods - Support Vector Learning. MIT Press, 1999, ch. Fast Training of Support Vector Machines using Sequential Minimal Optimization, pp. 185–208.
] B. Waske, J. Benediktsson, K. Arnason, and J. Sveinsson, “Mapping of Hyperspectral AVIRIS Data using Machine-Learning Algorithms,” Can. J. Remote Sensing, vol. 35, pp. 106–116, 2009.
Ahmed Cherif Megri, Sameer Hamoush, Ismail Zayd Megri, Yao Yu. (2021). Advanced Manufacturing Online STEM Education Pipeline for Early-College and High School Students. Journal of Online Engineering Education, 12(2), 01–06. Retrieved from http://onlineengineeringeducation.com/index.php/joee/article/view/47
Kelley, C. T., and E. W. Sachs. “Broyden’s Method for Approximate Solution of Nonlinear Integral Equations.” Journal of Integral Equations, vol. 9, no. 1, 1985, pp. 25–43, http://www.jstor.org/stable/26164266. Accessed 19 Apr. 2022.
Yang, J.-P. . “A Novel Storage Virtualization Scheme for Network Storage Systems”. International Journal on Recent and Innovation Trends in Computing and Communication, vol. 10, no. 1, Jan. 2022, pp. 08-13, doi:10.17762/ijritcc.v10i1.5514.
G. Camps-Valls, N. Shervashidze, and K. M. Borgwardt, “Spatio- Spectral Remote Sensing Image Classification with Graph Kernels,” IEEE Geosci. Remote Sens. Lett., vol. 7, no. 4, pp. 741–745, 2010.
J. Mu˜noz-Mar´ı, F. Bovolo, L. G´omez-Chova, L. Bruzzone, and G. Camp- Valls, “Semisupervised One-Class Support Vector Machines for Classification of Remote Sensing Data,” IEEE Trans. Geosci. Remote Sens., vol. 48, no. 8, pp. 3188–3197, 2010.
Cristianini, N., & Shawe-Taylor, J. (2000). Support Vector Machines. In An Introduction to Support Vector Machines and Other Kernel-based Learning Methods (pp. 93-124). Cambridge: Cambridge University Press. doi:10.1017/CBO9780511801389.008.
A. Mathur and G. Foody, “Multiclass and binary SVM classification: implications for training and classification users,” IEEE Geosci. Remote Sens. Lett., vol. 5, no. 2, pp. 241–245, 2008.
G. Foody, “RVM-Based Multi-Class Classification of Remotely Sensed. Data,” Int. J. Remote Sens., vol. 29, no. 6, pp. 1817–1823, 2008.
J. Platt, N. Cristianini, and J. Shawe-Taylor, “Large Margin DAGs for Multiclass Classification,” Adv. Neural Inf. Process. Syst., vol. 12, no. 3, pp. 547–553, 2000.
Ghazaly, N. M. . (2022). Data Catalogue Approaches, Implementation and Adoption: A Study of Purpose of Data Catalogue. International Journal on Future Revolution in Computer Science &Amp; Communication Engineering, 8(1), 01–04. https://doi.org/10.17762/ijfrcsce.v8i1.2063
G. Foody, “RVM-Based Multi-Class Classification of Remotely Sensed Data,” Int. J. Remote Sens., vol. 29, no. 6, pp. 1817–1823, 2008.
M. Tipping, “Sparse Bayesian Learning and the Relevance Vector Machine,” J. of Mach. Learn. Research, vol. 1, pp. 211–244, 2001.
Chawla, A. (2022). Phishing website analysis and detection using Machine Learning. International Journal of Intelligent Systems and Applications in Engineering, 10(1), 10–16. https://doi.org/10.18201/ijisae.2022.262
F. Giacco, C. Thiel, L. Pugliese, S. Scarpetta, and M. Marinaro, “Uncertainty Analysis for the Classification of Multispectral Satellite Images Using SVMs and SOMs,” IEEE Trans. Geosci. Remote Sens., no. 99, pp. 1–11, 2010.
Vapnik, V.(1995).:”Support-vector networks".Machine Learning. 20 (3): 273–297. doi:10.1007/BF00994018.
Christopher Houck, Jeffery Joines, Michael Kay, A Genetic Algorithm for Function Optimization: A Matlab Implementation, NCSU-IE Technical Report 95-09, 1996.
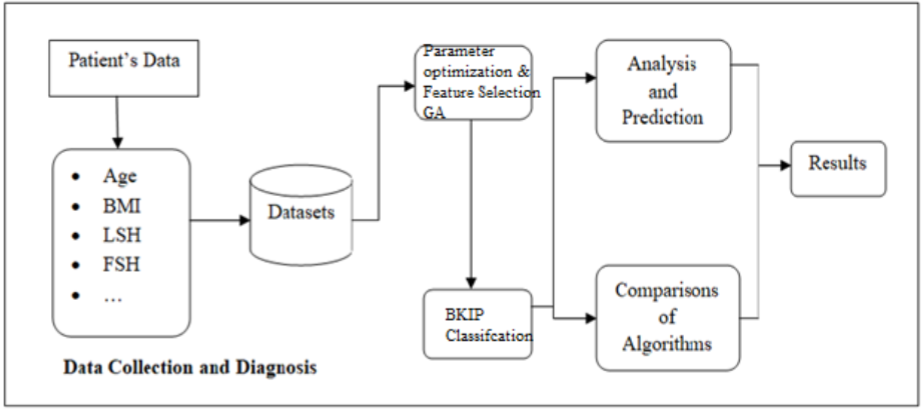
Downloads
Published
How to Cite
Issue
Section
License

This work is licensed under a Creative Commons Attribution-ShareAlike 4.0 International License.
All papers should be submitted electronically. All submitted manuscripts must be original work that is not under submission at another journal or under consideration for publication in another form, such as a monograph or chapter of a book. Authors of submitted papers are obligated not to submit their paper for publication elsewhere until an editorial decision is rendered on their submission. Further, authors of accepted papers are prohibited from publishing the results in other publications that appear before the paper is published in the Journal unless they receive approval for doing so from the Editor-In-Chief.
IJISAE open access articles are licensed under a Creative Commons Attribution-ShareAlike 4.0 International License. This license lets the audience to give appropriate credit, provide a link to the license, and indicate if changes were made and if they remix, transform, or build upon the material, they must distribute contributions under the same license as the original.