Residential Property Value Modeling using the Group Methods of Data Handling-Neural Network and Regression Analysis: A Case Study of GHMC, India
Keywords:
Residential Property Value, Significant Property facilities, Group Methods of Data Handling- Neural Network (GMDH-NN), Linear Regression AnalysisAbstract
location's demand and the purchasers' affordability determine the property's optimal value. To meet this optimal price the facilities in the properties are arranged through house sorting. Before sorting, it is important to find the significant facilities that contribute to the property value. The previous works mostly through qualitative assessment have identified the important factors influencing residential property value. The current article aims to determine the significant property facilities that are contributing to its value using statistical techniques. The facilities of a residential property considered in this study are Total Area, Age, Maintenance Cost, Number of bedrooms, Number of Toilet, Number of Balconies, facing of the property, Furnishing, Availability of Power Backup, Security, Elevator, Parking facilities. Data needed for the study were collected through a ground survey and interviews with the owners and real estate agents in the Greater Hyderabad Municipal Corporation (GHMC) Region. A total of 202 residential apartments that are yet to be sold were considered for the model development and 32 sold-out properties were obtained to validate the models. The significant facilities are identified using the p-test and the Group Methods of Data Handling- Neural Network (GMDH-NN) and linear regression techniques are adopted to develop the models for all possible combinations of the most significant factors. The accuracy of the developed models is assessed using R2 and Root Mean Square Error (RMSE) and Mean Average Percentage Error (MAPE). The study's findings indicate that the total area of the Residential apartment and the maintenance cost are the most significant factors. Both GMDH-NN and linear regression models developed using these factors show that the consideration of the Total area alone as a variable for prediction of property value has R2 > 0.748 and the RMSE and MAPE being less than 30%. The accuracy of prediction for the validation datasets using the Total area of the property as an input variable is more than 70% for both models. Identifying the significant factors using statistical techniques and then developing the models using these factors is the novelty of the research. the proposed models in the article can be used directly to understand the preferred facilities in Residential properties in the GHMC region and the adopted framework can be used for property valuation in general for any region
Downloads
References
Brandt, S., & Maennig, W. (2011). Road noise exposure and residential property prices: Evidence from Hamburg. Transportation Research Part D: Transport and Environment, 16(1), 23–30.
Burinskienė, M., Rudzkiene, V., & Venckauskaitė, J. (2011). MODELS OF FACTORS INFLUENCING THE REAL ESTATE PRICE.
J, M. S., S. K. Dr.N.C., M. Dr. P., T. N, and J. P. S. “IEEHR: Improved Energy Efficient Honeycomb Based Routing in MANET for Improving Network Performance and Longevity”. International Journal on Recent and Innovation Trends in Computing and Communication, vol. 10, no. 7, July 2022, pp. 85-93, doi:10.17762/ijritcc.v10i7.5575.
Chau, K. W., & Chin, T. L. (2003). A critical review of literature on the hedonic price model. International Journal for Housing Science and Its Applications, 27(2), 145–165.
Chau, K. W., Wong, S. K., & Yiu, C. Y. (2004). The value of the provision of a balcony in apartments in Hong Kong. Property Management, 22(3), 250–264.
Choy, L. H. T., Mak, S. W. K., & Ho, W. K. O. (2007). Modeling Hong Kong real estate prices. Journal of Housing and the Built Environment, 22(4), 359–368.
Ferlan, N., Bastic, M., & Psunder, I. (2017). Influential factors on the market value of residential properties. Engineering Economics, 28(2), 135–144. https://doi.org/10.5755/j01.ee.28.2.13777
Fisher, J. D., & Martin, R. S. (1994). Income property valuation. Kaplan Publishing.
Fletcher, M., Gallimore, P., & Mangan, J. (2000). Heteroscedasticity in hedonic house price models. Journal of Property Research, 17(2), 93–108.
Grebler, L., Blank, D. M., & Winnick, L. (1956). Capital formation in residential real estate. Princeton University Press Princeton.
Baes, A. M. M. ., Adoptante, A. J. M. ., Catilo, J. C. A. ., Lucero, P. K. L. ., Peralta, J. F. P., & de Ocampo, A. L. P. (2022). A Novel Screening Tool System for Depressive Disorders using Social Media and Artificial Neural Network. International Journal of Intelligent Systems and Applications in Engineering, 10(1), 116–121. https://doi.org/10.18201/ijisae.2022.274
Janmaat, J. A. (2005). Factors affecting Residential Property Values in a Small Historic Canadian University Town In Nova Scotia. May.
Joslin, A. (2005). An investigation into the expression of uncertainty in property valuations. Journal of Property Investment & Finance.
Kordík, P., Náplava, P., Snorek, M., & Genyk-Berezovskyj, M. (2003). Modified GMDH method and models quality evaluation by visualization. Control Systems and Computers, 2, 68–75.
Lenk, M. M., Worzala, E. M., & Shiva, A. (1997). High‐tech valuation: should artificial neural networks bypass the human valuer? Journal of Property Valuation and Investment.
McCluskey, W., & Anand, S. (1999). The application of intelligent hybrid techniques for the mass appraisal of residential properties. Journal of Property Investment & Finance.
M. J. Traum, J. Fiorentine. (2021). Rapid Evaluation On-Line Assessment of Student Learning Gains for Just-In-Time Course Modification. Journal of Online Engineering Education, 12(1), 06–13. Retrieved from http://onlineengineeringeducation.com/index.php/joee/article/view/45
McCluskey, W. J., Deddis, W. G., Lamont, I. G., & Borst, R. A. (2000). The application of surface generated interpolation models for the prediction of residential property values. Journal of Property Investment & Finance.
McGreal, S., Adair, A., McBurney, D., & Patterson, D. (1998). Neural networks: the prediction of residential values. Journal of Property Valuation and Investment.
Pandya, A. S., Kondo, T., Shah, T. U., & Gandhi, V. R. (1999). Prediction of stock market characteristics using neural networks. Applications and Science of Computational Intelligence II, 3722, 189–197.
Rodgers, T. (1994). Property-to-property comparison. The Appraisal Journal, 62(1), 64.
Rossini, P. (1997). Artificial neural networks versus multiple regression in the valuation of residential property. Australian Land Economics ReviewRossini, P. (1997). Artificial Neural Networks versus Multiple Regression in the Valuation of Residential Property. Australian Land Economics Review, 3(1), 1–12., 3(1), 1–12.
Selim, H. (2009). Determinants of house prices in Turkey: Hedonic regression versus artificial neural network. Expert Systems with Applications, 36(2), 2843–2852.
Sirmans, G. S., MacDonald, L., Macpherson, D. A., & Zietz, E. N. (2006). The value of housing characteristics: a meta-analysis. The Journal of Real Estate Finance and Economics, 33(3), 215–240.
Ustaoğlu, E. (2003). Hedonic price analysis of office rents: A case study of the office market in Ankara. Middle East Technical University.
Yayar, R., & Deniz, G. Ü. L. (2014). Mersin kent merkezinde konut piyasası fiyatlarının hedonik tahmini. Anadolu Üniversitesi Sosyal Bilimler Dergisi, 14(3), 87–100.
Yilmazel, S., Afsar, A., & Yilmazel, Ö. (2017). Analysis of apartments for sale in turkey based on city and region by using big data technologies. Sakarya İktisat Dergisi, 6(3), 1–21.
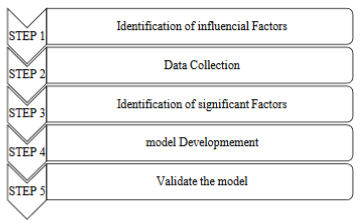
Downloads
Published
How to Cite
Issue
Section
License

This work is licensed under a Creative Commons Attribution-ShareAlike 4.0 International License.
All papers should be submitted electronically. All submitted manuscripts must be original work that is not under submission at another journal or under consideration for publication in another form, such as a monograph or chapter of a book. Authors of submitted papers are obligated not to submit their paper for publication elsewhere until an editorial decision is rendered on their submission. Further, authors of accepted papers are prohibited from publishing the results in other publications that appear before the paper is published in the Journal unless they receive approval for doing so from the Editor-In-Chief.
IJISAE open access articles are licensed under a Creative Commons Attribution-ShareAlike 4.0 International License. This license lets the audience to give appropriate credit, provide a link to the license, and indicate if changes were made and if they remix, transform, or build upon the material, they must distribute contributions under the same license as the original.