Nutrition-rich Food Suggestion for Cancer Patient using CapsNet
Keywords:
CapsNet, Deep Neural Network, Food Capsule layer, Primary Capsule layer, Routing AgreementAbstract
Food is important for human survival and has been the focus of several medical conventions. Novel dietary assessment and nutrition investigation technologies now provide more options to assist people in understanding their regular eating habits, researching nutrition trends, and maintaining a balanced diet. People with cancer are now advised to eat a nutritious, balanced diet in order to maintain their quality of life and achieve optimal health results. It is necessary to maintain a healthy body weight and to consume nutritional foods. The major purpose of this work is to provide healthy and nutritious meal suggestions for cancer patients. This research proposes a CapsNet model, a kind of deep neural network, for food recommendation. Preprocessing, feature extraction, and classification are the three key procedures in the proposed study. Reshaping, squash function and routing agreement are the major operations of the proposed work. There are six layers are used to build the CapsNet model which also has the two main layers called Primary Capsule layer and Food Capsule layer. After preprocessing of the food image, the features are extracted. As a final point, depending on the identification outcomes, the system will evaluate the nutritional substances and afford a dietary assessment report by estimating the capacity of fat, calories and carbohydrate. For analysis Food-101 dataset and cancer patient dataset are used. The performance of the proposed methodology is analyzed through different evaluation metrics and achieving an accuracy of 95% and the loss value also decreased. To prove the effectiveness of the proposed methods, this study presents a comparison with the advanced techniques.
Downloads
References
Suma, S., Bharathi, M., Harikumar, G., & Deepak, B. (2020). Food Recognition and Calorie Estimation Using Image Processing. International Journal of Advance Research and Innovative Ideas in Education (IJARIIE), 6(1).
Bulla, P. . “Traffic Sign Detection and Recognition Based on Convolutional Neural Network”. International Journal on Recent and Innovation Trends in Computing and Communication, vol. 10, no. 4, Apr. 2022, pp. 43-53, doi:10.17762/ijritcc.v10i4.5533.
Gao, X., Feng, F., He, X., Huang, H., Guan, X., Feng, C., Ming, Z., & Chua, T. (2020). Hierarchical attention network for visually-aware food recommendation. IEEE Transactions on Multimedia, 22(6), 1647-1659.
Rostami, M., Oussalah, M., & Farrahi, V. (2022). A novel time-aware food recommender-system based on deep learning and graph clustering. IEEE Access, 10, 52508-52524.
Agarwal, D. A. . (2022). Advancing Privacy and Security of Internet of Things to Find Integrated Solutions. International Journal on Future Revolution in Computer Science &Amp; Communication Engineering, 8(2), 05–08. https://doi.org/10.17762/ijfrcsce.v8i2.2067
Kalyani, G., Janakiramaiah, B., Karuna, A., & Prasad, L. V. (2021). Diabetic retinopathy detection and classification using capsule networks. Complex & Intelligent Systems.
Naik, P. A. (2020). Intelligent food recommendation system using machine learning. International Journal of Innovative Science and Research Technology, 5(8), 616-619.
Satish, A. B. ., & Rechanna, D. (2022). An Empirical Study on the Impact of HRD Practices on NPOs in Utilizing Foreign Contributions Received. International Journal of New Practices in Management and Engineering, 11(01), 01–14. https://doi.org/10.17762/ijnpme.v11i01.143
Food 101. (n.d.). Retrieved from https://www.kaggle.com/datasets/dansbecker/food-101?resource=downloadhttps://www.kaggle.com/datasets/dansbecker/food-101?resource=download
Yu, Y., Guan, H., Li, D., Gu, T., Wang, L., Ma, L., & Li, J. (2020). A hybrid capsule network for land cover classification using Multispectral LiDAR data. IEEE Geoscience and Remote Sensing Letters, 17(7), 1263-1267.
Sudhakar, C. V., & Reddy, G. U. . (2022). Land use Land cover change Assessment at Cement Industrial area using Landsat data-hybrid classification in part of YSR Kadapa District, Andhra Pradesh, India. International Journal of Intelligent Systems and Applications in Engineering, 10(1), 75–86. https://doi.org/10.18201/ijisae.2022.270
Ma, X., Zhong, H., Li, Y., Ma, J., Cui, Z., & Wang, Y. (2021). Forecasting transportation network speed using deep capsule networks with nested LSTM models. IEEE Transactions on Intelligent Transportation Systems, 22(8), 4813-4824.
Wang, C., Wu, Y., Wang, Y., Chen, Y., & Gao, Y. (2021). Multilevel capsule weighted aggregation network based on a decoupled dynamic filter for remote sensing scene classification. IEEE Access, 9, 125309-125319.
Ahmed Cherif Megri, Sameer Hamoush, Ismail Zayd Megri, Yao Yu. (2021). Advanced Manufacturing Online STEM Education Pipeline for Early-College and High School Students. Journal of Online Engineering Education, 12(2), 01–06. Retrieved from http://onlineengineeringeducation.com/index.php/joee/article/view/47
W. R. Emmanuel, S. J. Minija, “Fuzzy clustering and whale-based neural network to food recognition and calorie estimation for daily dietary assessment,” Sādhanā, vol. 43, no. 5, 2018.
Shanthi, S., & Rajkumar, N. (2020). Lung cancer prediction using stochastic diffusion search (SDS) based feature selection and machine learning methods. Neural Processing Letters, 53(4), 2617-2630.
EL-YAHYAOUI, A., & OMARY, F. (2022). A Like ELGAMAL Cryptosystem But Resistant To Post-Quantum Attacks. International Journal of Communication Networks and Information Security (IJCNIS), 14(1). https://doi.org/10.17762/ijcnis.v14i1.5180
Shanthi, S., & Rajkumar, N. (2019). Non-small-cell lung cancer prediction using radiomic features and machine learning methods. International Journal of Computers and Applications, 1-9.
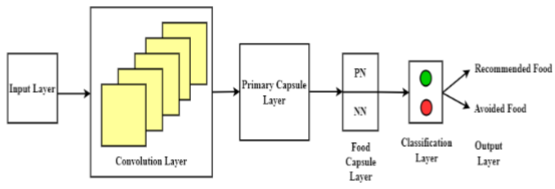
Downloads
Published
How to Cite
Issue
Section
License

This work is licensed under a Creative Commons Attribution-ShareAlike 4.0 International License.
All papers should be submitted electronically. All submitted manuscripts must be original work that is not under submission at another journal or under consideration for publication in another form, such as a monograph or chapter of a book. Authors of submitted papers are obligated not to submit their paper for publication elsewhere until an editorial decision is rendered on their submission. Further, authors of accepted papers are prohibited from publishing the results in other publications that appear before the paper is published in the Journal unless they receive approval for doing so from the Editor-In-Chief.
IJISAE open access articles are licensed under a Creative Commons Attribution-ShareAlike 4.0 International License. This license lets the audience to give appropriate credit, provide a link to the license, and indicate if changes were made and if they remix, transform, or build upon the material, they must distribute contributions under the same license as the original.