Analytical Progression Scale for Arrhythmia Scope prediction from Electrocardiograms
Keywords:
Analytical Progression scale, electrocardiogram, electrophysiology, cross validation, machine learningAbstract
Machine Learning (ML) techniques have exploded in popularity, especially the use of ML in automated ECG interpretation, which has been widely addressed in the literature. Other applications of machine learning in cardiac electrophysiology as well as arrhythmia are even less well recognised. Yet, the contemporary models are evincing the considerable false alarming in the process of arrhythmia prediction. In order to improve the arrhythmia prediction accuracy, this manuscript portrayed a novel analytical progression scale (APS) that learns from the given input electrocardiograms with appropriate label positive (prone to arrhythmia) or negative (not prone to arrhythmia). The experimental study has carried a 10-fold cross validation strategy on proposed and other contemporary models to scale the performance advantage of the proposed Analytical Progression Scale that compare those statistical values obtained for performance metrics such as precision, sensitivity, specificity, and accuracy. The results obtained from cross validation are evincing that the proposed model APS is outperforming the other contemporary models.
Downloads
References
Goldberger, A. L., G. B. Moody, and M. D. Costa. "Physionet." Nonlinear dynamics, fractals, and chaos theory: implications for neuroanatomic heart rate control in health and disease. http://www. physionet. org/tutorials/ndc (1999).
Qurraie, Safa Sultan, and Rashid Ghorbani Afkhami. "ECG arrhythmia classification using time frequency distribution techniques." Biomedical engineering letters 7.4 (2017): 325-332.
Afkhami, Rashid Ghorbani, Ghanbar Azarnia, and Mohammad Ali Tinati. "Cardiac arrhythmia classification using statistical and mixture modeling features of ECG signals." Pattern Recognition Letters 70 (2016): 45-51.
Krasteva, Vessela, et al. "Superiority of classification tree versus cluster, fuzzy and discriminant models in a heartbeat classification system." PloS one 10.10 (2015): e0140123.
Boyapati, B. ., and J. . Kumar. “Parasitic Element Based Frequency Reconfigurable Antenna With Dual Wideband Characteristics for Wireless Applications”. International Journal on Recent and Innovation Trends in Computing and Communication, vol. 10, no. 6, June 2022, pp. 10-23, doi:10.17762/ijritcc.v10i6.5619.
Elhaj, Fatin A., et al. "Arrhythmia recognition and classification using combined linear and nonlinear features of ECG signals." Computer methods and programs in biomedicine 127 (2016): 52-63.
Martis, Roshan Joy, U. Rajendra Acharya, and Lim Choo Min. "ECG beat classification using PCA, LDA, ICA and discrete wavelet transform." Biomedical Signal Processing and Control 8.5 (2013): 437-448.
Xiao, Xuan, Zhi-Cheng Wu, and Kuo-Chen Chou. "A multi-label classifier for predicting the subcellular localization of gram-negative bacterial proteins with both single and multiple sites." PloS one 6.6 (2011): e20592.
Kiranyaz, Serkan, Turker Ince, and Moncef Gabbouj. "Real-time patient-specific ECG classification by 1-D convolutional neural networks." IEEE Transactions on Biomedical Engineering 63.3 (2015): 664-675.
Zhang, Zhancheng, et al. "Heartbeat classification using disease-specific feature selection." Computers in biology and medicine 46 (2014): 79-89.
Huang, Huifang, et al. "A new hierarchical method for inter-patient heartbeat classification using random projections and RR intervals." Biomedical engineering online 13.1 (2014): 1-26.
Garcia, G., et al. "Inter-patient ECG heartbeat classification with temporal VCG optimized by PSO. Sci. Rep. 7, 10543–10544 (2017)."
Raj, Sandeep, Kailash Chandra Ray, and Om Shankar. "Cardiac arrhythmia beat classification using DOST and PSO tuned SVM." Computer methods and programs in biomedicine 136 (2016): 163-177.
De Lannoy, Gaël, et al. "Weighted conditional random fields for supervised interpatient heartbeat classification." IEEE Transactions on Biomedical Engineering 59.1 (2011): 241-247.
Wu, Zhiyong, Xiangqian Ding, and Guangrui Zhang. "A novel method for classification of ECG arrhythmias using deep belief networks." International Journal of Computational Intelligence and Applications 15.04 (2016): 1650021.
Acharya, U. Rajendra, et al. "A deep convolutional neural network model to classify heartbeats." Computers in biology and medicine 89 (2017): 389-396.
Al Rahhal, Mohamad Mahmoud, et al. "Deep learning approach for active classification of electrocardiogram signals." Information Sciences 345 (2016): 340-354.
Li, Hongqiang, et al. "Genetic algorithm for the optimization of features and neural networks in ECG signals classification." Scientific reports 7.1 (2017): 1-12.
Gunjan, Vinit Kumar, and Madapuri Rudra Kumar. "Predictive Analytics for OSA Detection Using Non-Conventional Metrics." International Journal of Knowledge-Based Organizations (IJKBO) 10.4 (2020): 13-23.
Ye, Can, BVK Vijaya Kumar, and Miguel Tavares Coimbra. "An automatic subject-adaptable heartbeat classifier based on multiview learning." IEEE journal of biomedical and health informatics 20.6 (2015): 1485-1492.
Zidelmal, Zahia, et al. "ECG beat classification using a cost sensitive classifier." Computer methods and programs in biomedicine 111.3 (2013): 570-577.
Gupta, D. J. . (2022). A Study on Various Cloud Computing Technologies, Implementation Process, Categories and Application Use in Organisation. International Journal on Future Revolution in Computer Science &Amp; Communication Engineering, 8(1), 09–12. https://doi.org/10.17762/ijfrcsce.v8i1.2064
Ye, Can, BVK Vijaya Kumar, and Miguel Tavares Coimbra. "Heartbeat classification using morphological and dynamic features of ECG signals." IEEE Transactions on Biomedical Engineering 59.10 (2012): 2930-2941.
Choi, Edward, et al. "Using recurrent neural network models for early detection of heart failure onset." Journal of the American Medical Informatics Association 24.2 (2017): 361-370.
Choi, Edward, et al. "Doctor ai: Predicting clinical events via recurrent neural networks." Machine learning for healthcare conference. PMLR, 2016, 301-318.
Ghassemi, Marzyeh, et al. "A multivariate timeseries modeling approach to severity of illness assessment and forecasting in ICU with sparse, heterogeneous clinical data." Proceedings of the AAAI conference on artificial intelligence. Vol. 29. No. 1. 2015.
Churpek, Matthew M., Richa Adhikari, and Dana P. Edelson. "The value of vital sign trends for detecting clinical deterioration on the wards." Resuscitation 102 (2016): 1-5.
Pławiak, Paweł, and U. Rajendra Acharya. "Novel deep genetic ensemble of classifiers for arrhythmia detection using ECG signals." Neural Computing and Applications 32.15 (2020): 11137-11161.
Tuncer, Turker, et al. "Automated arrhythmia detection using novel hexadecimal local pattern and multilevel wavelet transform with ECG signals." Knowledge-Based Systems 186 (2019): 104923.
Kandala, Rajesh NVPS, et al. "Towards real-time heartbeat classification: evaluation of nonlinear morphological features and voting method." Sensors 19.23 (2019): 5079.
Pławiak, Paweł, and Moloud Abdar. "Novel methodology for cardiac arrhythmias classification based on long-duration ECG signal fragments analysis." Biomedical signal processing. Springer, Singapore, 2020. 225-272.
Pławiak, Paweł. "Novel genetic ensembles of classifiers applied to myocardium dysfunction recognition based on ECG signals." Swarm and evolutionary computation 39 (2018): 192-208.
Pławiak, Paweł. "Novel methodology of cardiac health recognition based on ECG signals and evolutionary-neural system." Expert Systems with Applications 92 (2018): 334-349.
Fuad Ali Mohammed Al-Yarimi, Nabil Mohammed Ali Munassar, Fahd Awesabi. Electrocardiogram stream level correlated patterns as features to classify heartbeats for arrhythmia prediction. Data Technologies and Applications Emerald Publishing Limited. 2020.
Ortín González, Silvia, et al. “Automated real-time method for ventricular heartbeat classification.” (2019).
Rosemaro, E. . (2022). Understanding the Concept of Entrepreneurship Management and Its Contribution in Organization. International Journal of New Practices in Management and Engineering, 11(01), 24–30. https://doi.org/10.17762/ijnpme.v11i01.159
Madapuri, Rudra Kumar, and P. C. Mahesh. "HBS-CRA: scaling impact of change request towards fault proneness: defining a heuristic and biases scale (HBS) of change request artifacts (CRA)." Cluster Computing 22.5 (2019): 11591-11599.
Rudra Kumar, M., and Vinit Kumar Gunjan. "Machine Learning Based Solutions for Human Resource Systems Management." ICCCE 2021. Springer, Singapore, 2022. 1239-1249
Haynes, Winston. "Wilcoxon rank sum test." Encyclopedia of Systems Biology. Springer New York, 2013. 2354-2355.
Sahoo, Prasanna, and Thomas Riedel. Mean value theorems and functional equations. World Scientific, 1998.
Chalapathi, M. M., et al. "Ensemble Learning by High-Dimensional Acoustic Features for Emotion Recognition from Speech Audio Signal." Security and Communication Networks 2022 (2022).
Kumar, S., Gornale, S. S., Siddalingappa, R., & Mane, A. (2022). Gender Classification Based on Online Signature Features using Machine Learning Techniques. International Journal of Intelligent Systems and Applications in Engineering, 10(2), 260–268. Retrieved from https://ijisae.org/index.php/IJISAE/article/view/2020
Rudra Kumar, M., Rashmi Pathak, and Vinit Kumar Gunjan. "Machine Learning-Based Project Resource Allocation Fitment Analysis System (ML-PRAFS)." Computational Intelligence in Machine Learning. Springer, Singapore, 2022. 1-14
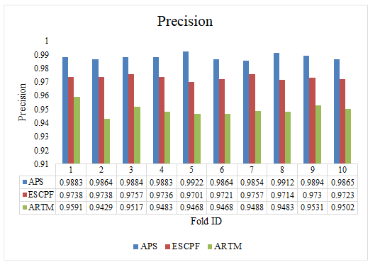
Downloads
Published
How to Cite
Issue
Section
License

This work is licensed under a Creative Commons Attribution-ShareAlike 4.0 International License.
All papers should be submitted electronically. All submitted manuscripts must be original work that is not under submission at another journal or under consideration for publication in another form, such as a monograph or chapter of a book. Authors of submitted papers are obligated not to submit their paper for publication elsewhere until an editorial decision is rendered on their submission. Further, authors of accepted papers are prohibited from publishing the results in other publications that appear before the paper is published in the Journal unless they receive approval for doing so from the Editor-In-Chief.
IJISAE open access articles are licensed under a Creative Commons Attribution-ShareAlike 4.0 International License. This license lets the audience to give appropriate credit, provide a link to the license, and indicate if changes were made and if they remix, transform, or build upon the material, they must distribute contributions under the same license as the original.