A Novel Deep Learning Model to Enhance Network Traffic Monitoring for Cybersecurity
Keywords:
Cyber Security, Deep Learning, Monitoring, Networks, TrafficAbstract
The data amount flowing through computer networks at any specified period is referred to as network traffic. Data packets are broken down into networks traffic and transmitted over a network before being reconstructed by the receiving computers or devices. In the present time, network traffic has become a major problem of rapid increment in network devices which creates complexity in networking data. So, to overcome this problem, the author gives the method which is linked with deep learning methods and will also help to monitor the network traffic for cybersecurity. This paper gives the deep learning method which helps to find the network traffic as well as providing the cybersecurity to that network. The suggested method contains the deep learning method (DLM), convolutional neural network (CNN), recurrent neural network (RNN) and Long Short-Term Memory (LSTM). The method provides better accuracy results for observing the network traffic and also provides cybersecurity by using deep learning methods. This research shows that deep learning techniques can be used to build massive deep learning techniques for networks classification that can be applied in the real world where they can preserve classifying accuracy and increase classification speed considering restricted resource availability.
Downloads
References
G. Nguyen, S. Dlugolinsky, V. Tran, and A. Lopez Garcia, “Deep learning
for proactive network monitoring and security protection,” IEEE Access, vol. 8,
pp. 19696–19716, 2020, doi: 10.1109/ACCESS.2020.2968718.
I. Sohn, “Deep belief network based intrusion detection techniques: A survey,” Expert Systems with Applications. 2021, doi: 10.1016/j.eswa.2020.114170.
M. Abbasi, A. Shahraki, and A. Taherkordi, “Deep Learning for Network Traffic Monitoring and Analysis (NTMA): A Survey,” Computer Communications. 2021, doi: 10.1016/j.comcom.2021.01.021.
Y. Chen et al., “An Optimizing and Differentially Private Clustering Algorithm for Mixed Data in SDN-Based Smart Grid,” IEEE Access, 2018.
I. M. Venter, R. J. Blignaut, K. Renaud, and M. A. Venter, “Cyber security education is as essential as ‘the three R’s,’” Heliyon, 2019, doi: 10.1016/j.heliyon.2019.e02855.
M. S. Abu, S. R. Selamat, A. Ariffin, and R. Yusof, “Cyber threat intelligence – Issue and challenges,” Indones. J. Electr. Eng. Comput. Sci., 2018, doi: 10.11591/ijeecs.v10.i1.pp371-379.
M. Medvedeva, M. Vols, and M. Wieling, “Using machine learning to predict decisions of the European Court of Human Rights,” Artif. Intell. Law, 2020, doi: 10.1007/
s10506-019-09255-y.
N. Miloslavskaya and A. Tolstoy, “New SIEM system for the internet of things,” 2019, doi: 10.1007/978-3-030-16184-2_31.
A. Brogi et al., “Survey High-Performance Modelling and Simulation for Selected Results of the COST Action IC1406 cHiPSet,” Futur. Gener. Comput. Syst., 2018.
M. Amjad, H. Zahid, S. Zafar, and T. Mahmood, “A novel deep learning framework for intrusion detection system,” 2019 Int. Conf. Adv. Emerg. Comput. Technol. AECT 2019, 2020, doi: 10.1109/AECT47998.2020.9194224.
G. Nguyen, S. Dlugolinsky, V. Tran, and A. Lopez Garcia, “Deep learning for proactive network monitoring and security protection,” IEEE Access, 2020, doi: 10.1109/ACCESS.2020.2968718.
H. Dhillon and A. Haque, “Towards network traffic monitoring using deep transfer learning,” Proc. - 2020 IEEE 19th Int. Conf. Trust. Secur. Priv. Comput. Commun. Trust. 2020, pp. 1089–1096, 2020, doi: 10.1109/TrustCom50675.2020.00144.
P. Guo, Z. Liu, H. Lu, and Z. Wang, “Hyperspectral Image Classification Based on Stacked Contractive Autoencoder Combined with Adaptive Spectral-Spatial Information,” IEEE Access, 2021, doi: 10.1109/ACCESS.2021.3095265.
S, R. D., L. . Shyamala, and S. . Saraswathi. “Adaptive Learning Based Whale Optimization and Convolutional Neural Network Algorithm for Distributed Denial of Service Attack Detection in Software Defined Network Environment”. International Journal on Recent and Innovation Trends in Computing and Communication, vol. 10, no. 6, June 2022, pp. 80-93, doi:10.17762/ijritcc.v10i6.5557.
W. Wang, X. Du, D. Shan, R. Qin, and N. Wang, “Cloud Intrusion Detection Method Based on Stacked Contractive Auto-Encoder and Support Vector Machine,” IEEE Trans. Cloud Comput., pp. 1–1, 2020, doi: 10.1109/tcc.2020.3001017.
Rosemaro, E. . (2022). Understanding the Concept of Entrepreneurship Management and Its Contribution in Organization. International Journal of New Practices in Management and Engineering, 11(01), 24–30. https://doi.org/10.17762/ijnpme.v11i01.159
S. Zavrak and M. Iskefiyeli, “Anomaly-Based Intrusion Detection from Network Flow Features Using Variational Autoencoder,” IEEE Access, vol. 8, pp. 108346–108358, 2020, doi: 10.1109/ACCESS.2020.3001350.
D. S. Berman, A. L. Buczak, J. S. Chavis, and C. L. Corbett, “A survey of deep learning methods for cyber security,” Inf., vol. 10, no. 4, 2019, doi: 10.3390/
info10040122.
Chaudhary, D. S. . (2022). Analysis of Concept of Big Data Process, Strategies, Adoption and Implementation. International Journal on Future Revolution in Computer Science &Amp; Communication Engineering, 8(1), 05–08. https://doi.org/10.17762/ijfrcsce.v8i1.2065
M. Särelä, T. Kyöstilä, T. Kiravuo, and J. Manner, “Evaluating intrusion prevention systems with evasions,” Int. J. Commun. Syst., 2017, doi: 10.1002/dac.3339.
M. Hasan, M. M. Islam, M. I. I. Zarif, and M. M. A. Hashem, “Attack and anomaly detection in IoT sensors in IoT sites using machine learning approaches,” Internet of Things (Netherlands), 2019, doi: 10.1016/j.iot.2019.100059.
X. Wang, S. Chen, and J. Su, “Real Network Traffic Collection and Deep Learning for Mobile App Identification,” Wirel. Commun. Mob. Comput., 2020, doi: 10.1155/
/4707909.
H. Li, Z. Zhang, and Z. Liu, “Application of artificial neural networks for catalysis: A review,” Catalysts. 2017, doi: 10.3390/catal7100306.
L. Wang, L. Zhang, and J. Jiang, “Duplicate Question Detection with Deep Learning in Stack Overflow,” IEEE Access, 2020, doi: 10.1109/ACCESS.2020.2968391.
Paithane, P. M., & Kakarwal, D. (2022). Automatic Pancreas Segmentation using A Novel Modified Semantic Deep Learning Bottom-Up Approach. International Journal of Intelligent Systems and Applications in Engineering, 10(1), 98–104. https://doi.org/10.18201/ijisae.2022.272
M. Aazam et al., “Fog computing - Glimps of Upcoming Research Area (#4),” Futur. Gener. Comput. Syst., 2017.
J. Gu et al., “Recent advances in convolutional neural networks,” Pattern Recognit., 2018, doi: 10.1016/j.patcog.2017.10.013.
Sally Fouad Shady. (2021). Approaches to Teaching a Biomaterials Laboratory Course Online. Journal of Online Engineering Education, 12(1), 01–05. Retrieved from http://onlineengineeringeducation.com/index.php/joee/article/view/43
M. Lopez-Martin, B. Carro, A. Sanchez-Esguevillas, and J. Lloret, “Network Traffic Classifier with Convolutional and Recurrent Neural Networks for Internet of Things,” IEEE Access, vol. 5, pp. 18042–18050, 2017, doi: 10.1109/ACCESS.2017.2747560.
Degambur, L.-N., Mungur, A., Armoogum, S., & Pudaruth, S. (2022). Resource Allocation in 4G and 5G Networks: A Review. International Journal of Communication Networks and Information Security (IJCNIS), 13(3). https://doi.org/10.17762/ijcnis.v13i3.5116
L. Aziato and H. O. Antwi, “Facilitators and barriers of herbal medicine use in Accra, Ghana: An inductive exploratory study,” BMC Complement. Altern. Med., 2016, doi: 10.1186/s12906-016-1124-y.
M. Cai and J. Liu, “Maxout neurons for deep convolutional and LSTM neural networks in speech recognition,” Speech Commun., 2016, doi: 10.1016/j.specom.2015.12.003.
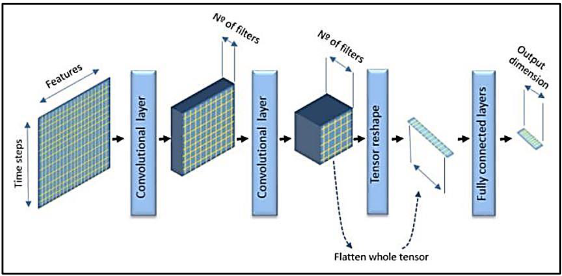
Downloads
Published
How to Cite
Issue
Section
License

This work is licensed under a Creative Commons Attribution-ShareAlike 4.0 International License.
All papers should be submitted electronically. All submitted manuscripts must be original work that is not under submission at another journal or under consideration for publication in another form, such as a monograph or chapter of a book. Authors of submitted papers are obligated not to submit their paper for publication elsewhere until an editorial decision is rendered on their submission. Further, authors of accepted papers are prohibited from publishing the results in other publications that appear before the paper is published in the Journal unless they receive approval for doing so from the Editor-In-Chief.
IJISAE open access articles are licensed under a Creative Commons Attribution-ShareAlike 4.0 International License. This license lets the audience to give appropriate credit, provide a link to the license, and indicate if changes were made and if they remix, transform, or build upon the material, they must distribute contributions under the same license as the original.