An Optimal Pruning Fuzzy Learning Model for Analysing Risk Factors of Tuberculosis
Keywords:
Computational intelligence, Fuzzy, Pruning Fuzzy, Fuzzy Neural Network, TuberculosisAbstract
The most prevalent pathogens disease that causes death is tuberculosis (TB). Approximately 1,6 million per year or 4,384 people die every day of Tuberculosis globally, surpasses death number of HIV and Malaria combined according to WHO, 2018. Computational intelligence has the ability to tackle complex, broad, and ambiguous problems. However, existing computational techniques such as Fuzzy logic and Neural Network suffer from performance degradation when fitting the model to a guidance data set. We use computational techniques to analyse the risk factors of Tuberculos is on patient dataset publicly available in WHO portal. We apply pruning fuzzy model to reduce the network size while maintaining the fitting accuracy. To categorise input data, fuzzy C-means classifier and fuzzy inference system are utilised. To improve prediction accuracy, we use an Adaptive Neuro-fuzzy Inference System to generate fuzzy rules. The findings indicate that the suggested technique has greater precision, which meets the needs of the physicians. As a result, the created system will benefit both regular people and medical professionals.
Downloads
References
N. Awoke, B. Dulo and F. Wudneh, "Total Delay in Treatment of Tuberculosis and Associated Factors among New Pulmonary TB Patients in Selected Health Facilities of Gedeo Zone, Southern Ethiopia, 2017/18", Interdisciplinary Perspectives on Infectious Diseases, vol. 2019, pp. 1-14, 2019. Available: 10.1155/2019/2154240.
B. Patterson and R. Wood, "Is cough really necessary for TB transmission?", Tuberculosis, vol. 117, pp. 31-35, 2019. Available: 10.1016/j.tube.2019.05.003.
A. Kizny Gordon, B. Marais, T. M. Walker, and V. Sintchenko, “Clinical and public health utility of mycobacterium tuberculosis whole genome sequencing,” International Journal of Infectious Diseases, vol. 113, pp. S40-S42, 2021.
M.-R. Lee, H.-L. Huang, L.-C. Chen, H.-C. Yang, J.-C. Ko, M.-H. Cheng, I.-W. Chong, L.-N. Lee, J.-Y. Wang, and G. Dimopoulos, “Seroprevalence of Aspergillus IGG and disease prevalence of chronic pulmonary aspergillosis in a country with intermediate burden of tuberculosis: A prospective observational study,” Clinical Microbiology and Infection, vol. 26, no. 8, pp.1091-e1, 2020.
R. Perrigot and K. ElomGbetchi, “Social franchise chains operating in African countries: Are their social goals aligned with the 2030 United Nations Sustainable Development Goals?,” International Journal of Emerging Markets, 2022.
P. J. Dodd, C. M. Yuen, S. M. Jayasooriya, M. M. van der Zalm, and J. A. Seddon, “Quantifying the global number of tuberculosis survivors: A modelling study,” The Lancet Infectious Diseases, vol. 21, no. 7, pp. 984–992, 2021.
A. Sharma, A. Sharma, R. Malhotra, P. Singh, R. K. Chakrabortty, S. Mahajan, and A. K. Pandit, “An accurate artificial intelligence system for the detection of pulmonary and extra pulmonary tuberculosis,” Tuberculosis, vol. 131, p. 102143, 2021.
K. D. Dale, M. Karmakar, K. J. Snow, D. Menzies, J. M. Trauer, and J. T. Denholm, “Quantifying the rates of late reactivation tuberculosis: A systematic review,” The Lancet Infectious Diseases, vol. 21, no. 10, pp. e303-e317, 2021.
Dale, K.D., Karmakar, M., Snow, K.J., Menzies, D., Trauer, J.M. and Denholm, J.T., 2021. Quantifying the rates of late reactivation tuberculosis: a systematic review. The Lancet Infectious Diseases, 21(10),.
B. Long, S. Y. Liang, A. Koyfman, and M. Gottlieb, “Tuberculosis: A focused review for the Emergency Medicine Clinician,” The American Journal of Emergency Medicine, vol. 38, no. 5, pp. 1014–1022, 2020.
L. Chelkeba, G. Fekadu, G. Tesfaye, F. Belayneh, T. Melaku, and Z. Mekonnen, “Effects of time of initiation of antiretroviral therapy in the treatment of patients with HIV/TB co-infection: A systemic review and meta-analysis,” Annals of Medicine and Surgery, vol. 55, pp. 148–158, 2020.
M. I. Fale and Y. G. Abdulsalam, “Dr. Flynxz – a first aid Mamdani-SUGENO-type fuzzy expert system for differential symptoms-based diagnosis,” Journal of King Saud University - Computer and Information Sciences, vol. 34, no. 4, pp. 1138–1149, 2022.
S. Varela-Santos and P. Melin, “A new modular neural network approach with fuzzy response integration for lung disease classification based on multiple objective feature optimization in chest X-ray images,” Expert Systems with Applications, vol. 168, p. 114361, 2021.
B. M. Yahya, F. S. Yahya, and R. G. Thannoun, “Covid-19 prediction analysis using artificial intelligence procedures and GIS Spatial Analyst: A case study for Iraq,” Applied Geomatics, vol. 13, no. 3, pp. 481–491, 2021.
Z. Khalid, G. Khan, and M. A. Arbab, “Extrinsically evolved system for breast cancer detection,” Evolutionary Intelligence, 2022.
L. J. Muhammad and E. A. Algehyne, “Fuzzy based expert system for diagnosis of coronary artery disease in Nigeria,” Health and Technology, vol. 11, no. 2, pp. 319–329, 2021.
R. Kunhimangalam, S. Ovallath, and P. K. Joseph, “A novel fuzzy expert system for the identification of severity of carpal tunnel syndrome,” BioMed Research International, vol. 2013, pp. 1–12, 2013.
D. Rasi and S. N. Deepa, “Energy optimization of internet of things in wireless sensor network models using type‐2 Fuzzy Neural Systems,” International Journal of Communication Systems, vol. 34, no. 17, 2021.
Hernández-Julio, Y.F., Hernández, H.M., Guzmán, J.D.C., Nieto-Bernal, W., Díaz, R.R.G. and Ferraz, P.P., 2019, February. Fuzzy knowledge discovery and decision-making through clustering and dynamic tables: Application in medicine. In International Conference on Information Technology & Systems (pp. 122-130). Springer, Cham.
C. Alcalde and A. Burusco, "Reduction of the size of L-fuzzy contexts. A tool for differential diagnoses of diseases", International Journal of General Systems, vol. 48, no. 7, pp. 692-712, 2019. Available: 10.1080/03081079.2019.1620740.
B. Rathore and R. Gupta, "A fuzzy based hybrid decision-making framework to examine the safety risk factors of healthcare workers during COVID-19 outbreak", Journal of Decision Systems, vol. 31, no. 1-2, pp. 68-101, 2020. Available: 10.1080/12460125.2020.1862988.
Akila, D. and Balaganesh, D., 2021, April. Fuzzy Based Treatment Model for Critical Healthcare System. In 2021 2nd International Conference on Intelligent Engineering and Management (ICIEM) (pp. 193-198). IEEE.
Y. Zhang, W. Wang, X. Zhang and S. Wang, "Secondary Pulmonary Tuberculosis Recognition by 4-Direction Varying-Distance GLCM and Fuzzy SVM", Mobile Networks and Applications, 2022. Available: 10.1007/s11036-021-01901-7.
H. Thakkar, V. Shah, H. Yagnik and M. Shah, "Comparative anatomization of data mining and fuzzy logic techniques used in diabetes prognosis", Clinical eHealth, vol. 4, pp. 12-23, 2021. Available: 10.1016/j.ceh.2020.11.001.
N. Auwal, I. Goni, D. Ali, U. Ngene and I. Manga, "Image Processing Approach to Determine the Severity Level of Tuberculosis", Current Journal of Applied Science and Technology, vol. 37, no. 3, pp. 1-8, 2019.
A. Kukker and R. Sharma, "Modified Fuzzy Q Learning Based Classifier for Pneumonia and Tuberculosis", IRBM, vol. 42, no. 5, pp. 369-377, 2021. Available: 10.1016/j.irbm.2020.10.005.
E. Dogu, Y. Albayrak and E. Tuncay, "Multidrug-resistant tuberculosis risk factors assessment with intuitionistic fuzzy cognitive maps", Journal of Intelligent & Fuzzy Systems, vol. 38, no. 1, pp. 1083-1095, 2020. Available: 10.3233/jifs-179470.
G. Reddy, M. Reddy, K. Lakshmanna, D. Rajput, R. Kaluri and G. Srivastava, "Hybrid genetic algorithm and a fuzzy logic classifier for heart disease diagnosis", Evolutionary Intelligence, vol. 13, no. 2, pp. 185-196, 2019. Available: 10.1007/s12065-019-00327-1.
S. Das and M. Sanyal, "Machine intelligent diagnostic system (MIDs): an instance of medical diagnosis of tuberculosis", Neural Computing and Applications, vol. 32, no. 19, pp. 15585-15595, 2020. Available: 10.1007/s00521-020-04894-8.
E. Ontiveros-Robles and P. Melin, "Toward a development of general type-2 fuzzy classifiers applied in diagnosis problems through embedded type-1 fuzzy classifiers", Soft Computing, vol. 24, no. 1, pp. 83-99, 2019. Available: 10.1007/s00500-019-04157-2.
M. Fale and Y. Abdulsalam, "Dr. Flynxz – A First Aid Mamdani-Sugeno-type fuzzy expert system for differential symptoms-based diagnosis", Journal of King Saud University - Computer and Information Sciences, vol. 34, no. 4, pp. 1138-1149, 2022. Available: 10.1016/j.jksuci.2020.04.016.
M. Omisore, O. Samuel and E. Atajeromavwo, "A Genetic-Neuro-Fuzzy inferential model for diagnosis of tuberculosis", Applied Computing and Informatics, vol. 13, no. 1, pp. 27-37, 2017. Available: 10.1016/j.aci.2015.06.001.
T. Oladele, R. Ogundokun, J. Awotunde, M. Adebiyi and J. Adeniyi, "Diagmal: A Malaria Coactive Neuro-Fuzzy Expert System", Computational Science and Its Applications – ICCSA 2020, pp. 428-441, 2020. Available: 10.1007/978-3-030-58817-5_32 [Accessed 20 September 2022].
J. Du and M. J. Er, "A fast pruning algorithm for an Efficient Adaptive Fuzzy Neural Network," IEEE ICCA 2010, 2010, pp. 1030-1035, doi: 10.1109/ICCA.2010.5524417.
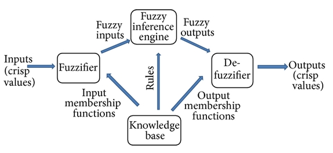
Downloads
Published
How to Cite
Issue
Section
License

This work is licensed under a Creative Commons Attribution-ShareAlike 4.0 International License.
All papers should be submitted electronically. All submitted manuscripts must be original work that is not under submission at another journal or under consideration for publication in another form, such as a monograph or chapter of a book. Authors of submitted papers are obligated not to submit their paper for publication elsewhere until an editorial decision is rendered on their submission. Further, authors of accepted papers are prohibited from publishing the results in other publications that appear before the paper is published in the Journal unless they receive approval for doing so from the Editor-In-Chief.
IJISAE open access articles are licensed under a Creative Commons Attribution-ShareAlike 4.0 International License. This license lets the audience to give appropriate credit, provide a link to the license, and indicate if changes were made and if they remix, transform, or build upon the material, they must distribute contributions under the same license as the original.