Exploring Vegetative Indices for Yield Prediction using Sentinel 2 Data – A Study in a Select Region of Karnataka
Keywords:
Crop Yield, NDVI, NDWI, SAVI, Correlation and Regression AnalysisAbstract
India is an agrarian economy and largest share of population depend on agriculture. Though there are mechanisms to approximately estimate crop yield by means of controlled experiments or past data, the reliability is limited. As a matter of fact, the crop yield is based on estimates that may suffer from multiple bias. In recent years, remote sensing images augmented with machine learning and deep learning techniques help us get the efficient crop yield statistics based on the crop on the field which help policymakers in devising better policies and governance. Remote sensing images of crops under study when subjected to machine learning techniques, one can classify the images into homogenous crop classes and record crop health / growth indicators such as Normalized Difference Vegetation Index (NDVI), Normalized Difference Water Index (NDWI) and Soil Adjusted Vegetative Index (SAVI) based on the training dataset and image quality, which further leads to crop yield estimates with desired level of accuracy. The present study investigates the relationship between yield influencing parameters such as physical variables, soil, weather characteristics and vegetation indices. Using correlation and multiple regression analysis, most efficient parameters that best estimate the crop yield is determined using the satellite data obtained for a study region in central part of the state of Karnataka. It was found that one of the vegetation indices, Soil Adjusted Vegetative Index values can predict near to accurate crop yield values by the end of 81 days after transplantation of paddy where as NDVI and NDVI can give yield estimated only after 116 days of transplantation. Thus crop yield estimates using SAVI works better in terms of predicting paddy yield at least one month before (after 81 days of transplantation) actual harvesting i.e., after 120 days as practiced by farmers in the study area.
Downloads
References
Babar, M. A., van Ginkel, M., Klatt, A. R., Prasad, B., & Reynolds, M. P. The potential of using spectral reflectance indices to estimate yield in wheat grown under reduced irrigation.Euphytica, 2006; 150(1–2), 155–172. https://doi.org/10.1007/s10681-006-9104-9
Battude,M.,AlBitar,A.,Morin,D.,Cros,J., Huc, M., Marais Sicre,C.,Le Dantec,V.,& Demarez,V. Estimating maize biomass and yield over large areas using high spatial and temporal resolution Sentinel-2 like remote sensing data. Remote Sensing Of Environment,184 ,668–681. https://doi.org/10.1016/j.rse.2016.07.030
Bognár,P.,Kern,A.,Pásztor,S.,Lichtenberger,J., Koronczay,D.,& Ferencz,C. Yield estimation and forecasting for winter wheat in Hungary using time series of MODIS data. International Journal of Remote Sensing,2017; 38(11), 3394–3414. https://doi.org/10.1080/01431161.2017.1295482
Campos,I.,González Gómez,L., Villodre,J., Calera,M., Campoy,J.,Jiménez, N.,Plaza,C.,Sánchez- Prieto,S. & Calera A, Mapping within-field variability in wheat yield and biomassusing remote sensing vegetation indices Precision Agriculture, 2019; 20(2), 214–236. https://doi.org/10.1007/ s11119-018-9596-z
Clevers,J.,Kooistra,L.,& vanden Brande, M Using Sentinel-2 data for retrieving LAI and leaf and canopy chlorophyll content of a potato crop, Remote Sensing, 2017.;9(5), 405. https://doi.org/10.3390/ rs9050405
Geisler, G. Pflanzenbau.Paul Parey. Evans, L.T., & Fischer,R.A.Yield potential: Its definition, measurement,and significance. Crop Science, 1999;39(6),1544–1551. https://doi.org/10.2135/cropsci1999.3961544x
Guo, C., Zhang, L., Zhou, X., Zhu, Y., Cao, W., Qiu, X., Cheng, T., &Tian, Y. Integrating remote sensing information with crop model to monitor wheat growth and yield based on simulation zone partitioning. Precision Agriculture, 2018; 19(1), 55–78. https://doi.org/10.1007/s11119-017-9498-5
Hack, H., Bleiholder, H., Buhr, L., Meier,U., Schnock-Fricke,U.Weber,E.,& Witzenberger, A. Auniform code for phenological growth stages of mono-and dicotyledonous plants—Extended BBCHscale,general-.Nachrichtenblatt Deutscher P flanzen schutzdienst,1992; 44(12),265–270.
Hunt,M.L.,Blackburn,G.A.,Carrasco,L.,Redhead,J. W.,& Rowland,C.SHigh resolution wheat yield mapping using Sentinel-2. Remote Sensing of Environment, 2019; 233, 111410. https://doi. org/10.1016/J.RSE.2019.111410
Journal, Knoblauch, C., Watson, C., Berendonk, C., Becker, R., Wrage-Mönnig, N., &Wichern, F. Relation- ship between remote sensing data, plant biomass and soil nitrogen dynamics in intensively managed grasslands under controlled conditions. Sensors,2017;17(7),1483. https://doi.org/10.3390/s17071483
Marti,J., Bort,J., Slafer,G.A.,& Araus,J. L. Can wheat yield be assessed by early measure- ments of Normalized Difference Vegetation Index. Annals of Applied Biology, 2007;150(2),253–257. https://doi.org/10.1111/j.1744-7348.2007.00126.x
Pasqualotto,N.,Delegido,J.,VanWittenberghe,S.,Rinaldi,M.,&Moreno,J. Multi-cropgreen LAI estimation with a new simple Sentinel-2 LAI Index (SeLI). Sensors.(2019).https://doi.org/10.3390/ s19040904.
Punalekar,S.M.,Verhoef,A.,Quaife,T.L.,Humphries,D.,Bermingham,L.,&Reynolds,C.K.Application of Sentinel-2 A data for pasture biomass monitoring using a physically based radioactive transfer model. Remote Sensing of Environment,2018;218,207–220.https://doi.org/10.1016/J.RSE.2018.09.028
Serrano,L.,Filella,I.,&Pen,J. Remote sensing of biomass and yield of winter wheat under different nitrogen supplies. Crop Science, 2000; 40, 723–731.
Barnes,E.M.,Clarke,T.R.,Richards,S.E.,Colaizzi,P.D.,Haberland,J,Kostrzewski,M.,Waller,P., Choi,C. Riley, E.,& Thompson,T. Coincident detection of crop water stress, nitrogen status and canopy density using ground-based multispectral data. In Proceedings of the 5th International Conference on Precision Agriculture and Other Resource Management. Bloomington, Madison, USA. 2000.
Baruth, B., Royer, A., Klisch, A., & Genovese, G. The use of remote sensing within the MARS crop yield monitoring system of the European Commission. Proceedings of ISPRS,2008; 27, 935–940
Arumugam, P.; Chemura, A.; Schauberger, B.; Gornott, C. Remote Sensing Based Yield Estimation of Rice (Oryza Sativa L.) Using Gradient Boosted Regression in India. Remote Sensing, MDPI. 2021, 13, 2379. https://doi.org/10.3390/rs13122379
Bose, P., Kasabov, N.K., “Spiking Neural Networks for Crop Yield Estimation Based on Spatiotemporal Analysis of Image Time Series”, IEEE transactions on geo-science and remote sensing, vol. 54, no. 11, pp. 6563-6573, 2016.
Gandhi, N., Armstrong, L.J., Petkar, O., “Proposed Decision Support System (DSS) for Indian Rice Crop Yield Prediction”, IEEE International Conference on Technological Innovations in ICT For Agriculture and Rural Development, pp. 13-18, 2016.
X.Zhou, H.B.Zheng et. al. Predicting grain yield in rice using multi-temporal vegetation indices from UAV-based multispectral and digital imagery, Volume 130, pp 246-255, 2017.
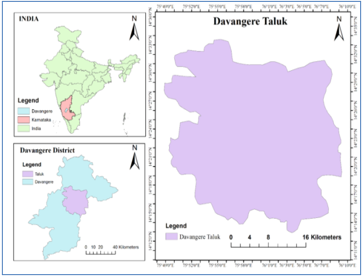
Downloads
Published
How to Cite
Issue
Section
License

This work is licensed under a Creative Commons Attribution-ShareAlike 4.0 International License.
All papers should be submitted electronically. All submitted manuscripts must be original work that is not under submission at another journal or under consideration for publication in another form, such as a monograph or chapter of a book. Authors of submitted papers are obligated not to submit their paper for publication elsewhere until an editorial decision is rendered on their submission. Further, authors of accepted papers are prohibited from publishing the results in other publications that appear before the paper is published in the Journal unless they receive approval for doing so from the Editor-In-Chief.
IJISAE open access articles are licensed under a Creative Commons Attribution-ShareAlike 4.0 International License. This license lets the audience to give appropriate credit, provide a link to the license, and indicate if changes were made and if they remix, transform, or build upon the material, they must distribute contributions under the same license as the original.