Research on the Philosophy of Science Oriented to Deep Learning under the Ethical Dilemma
Keywords:
Deep learning, Ethical dilemma, Philosophy of science, Random forest algorithmAbstract
The rapid growth in technology has resulted in the technical up-gradation of works in every field. In this study, we are going to research the philosophy of science-oriented to deep learning under the ethical dilemma The philosophy of science is concerned with the value and use of scientific knowledge and its underlying assumptions, techniques, and implications. An ethical dilemma arises when a person is forced to choose between two courses of action, neither of which is morally permissible. Random Forest Algorithm is used in this research to perform regression and classification tasks. It is found that the Random Forest Algorithm outperforms other algorithms in classification problems.
Downloads
References
A. Daly et al., “Artificial intelligence, governance and ethics: global perspectives”, SSRN Electron J., 2019.
P. De Sutter, Automated decision-making processes: ensuring consumer protection, and free movement of goods and services, 2020.
J. Cowls, “Deciding how to decide: six key questions for reducing AI’s democratic deficit,” in The 2019 Yearbook of the Digital Ethics Lab, C. Burr, S. Milano, eds. Springer International Publishing, Cham, pp. 101–116, 2020.
T. Dragičević, P. Wheeler, and F. Blaabjerg, “Artificial intelligence aided automated design for reliability of power electronic systems,” IEEE Trans Power Electron, vol. 34, pp. 7161–7171, 2019.
D. Greene, A. L. Hoffmann, L. Stark, “Better, nicer, clearer, fairer: a critical assessment of the movement for ethical artificial intelligence and machine learning.” in Proceedings of the 52nd Hawaii International Conference on System Sciences, 2019.
A. Jobi, M. Ienca, E. Vayena, “Artificial intelligence: The global landscape of ethics guidelines,” Nat. Mach. Intell., vol. 1, pp. 389–399, 2019.
B. Mittelstadt, “Principles alone cannot guarantee ethical AI,” Nat. Mach. Intell., vol. 1, pp. 501–507, 2019.
M. Brundage, S. Avin, J. Wang, H. Belfield, G. Krueger, G. Hadfield, H. Khlaaf et al., Toward trustworthy AI development: mechanisms for supporting verifiable claims. ArXiv:2004.07213 [Cs], 2020.
J. Cowls, A. Tsamados, M. Taddeo, L. Floridi, “A definition, benchmark and database of AI for social good initiatives,” Nat. Mach. Intell., 2021.
G. Ras, M. van Gerven, P. Haselager, Explanation methods in deep learning: users, values, concerns and challenges. ArXiv:1803.07517, 2018. http://arxiv.org/abs/1803.07517. Accessed 24 Aug 2020.
I. Rahwan, “Society-in-the-loop: programming the algorithmic social contract,” Ethics Inf. Technol., vol. 20, no. 1, pp. 5–14, 2018.
H. Roberts, J. Cowls, J. Morley, M. Taddeo, V. Wang, and L. Floridi, “The Chinese approach to artificial intelligence: An analysis of policy, ethics, and regulation,” AI Soc., vol. 36, pp. 59–77, 2020.
A. Erasmus, T. D. Brunet, and E. Fisher, “What is interpretability?” Philos. Technol., vol. 34, pp. 833–862, 2020.
L. H. Gilpin, D. Bau, B. Z. Yuan, A. Bajwa, M. Specter, and L. Kagal, “Explaining explanations: An overview of interpretability of machine learning.” in IEEE 5th international conference on data science and advanced analytics (DSAA), pp. 80–89, 2018. arXiv:1806.00069.
I. Goodfellow, Y. Bengio, and A. Courville, Deep learning: MIT Press, 2016.
J. Jebeile, V. Lam, and T. Räz, “Understanding climate change with statistical downscaling and machine learning,” Synthese, vol. 199, pp. 1877–1897, 2021.
A. Schubbach, “Judging machines: Philosophical aspects of deep learning,” Synthese, vol. 198, pp. 1807–1827, 2019.
R. Shwartz-Ziv and N. Tishby, Opening the black box of deep neural networks via information, 2017. ArXiv:1703.00810.
M. Reyes, R. Meier, S. Pereira, C. A. Silva, F. Dahlweid, H. von Tengg-Kobligk, R. M. Summers, and R. Wiest, “On the Interpretability of Artificial Intelligence in Radiology: Challenges and Opportunities,” Radiol. Artif. Intell., vol. 2, no. 3, 2020.
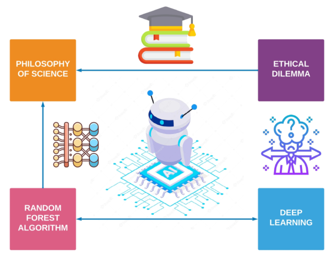
Downloads
Published
How to Cite
Issue
Section
License
Copyright (c) 2022 Jie Zhou, Ratna Roshida Ab. Razak

This work is licensed under a Creative Commons Attribution-ShareAlike 4.0 International License.
All papers should be submitted electronically. All submitted manuscripts must be original work that is not under submission at another journal or under consideration for publication in another form, such as a monograph or chapter of a book. Authors of submitted papers are obligated not to submit their paper for publication elsewhere until an editorial decision is rendered on their submission. Further, authors of accepted papers are prohibited from publishing the results in other publications that appear before the paper is published in the Journal unless they receive approval for doing so from the Editor-In-Chief.
IJISAE open access articles are licensed under a Creative Commons Attribution-ShareAlike 4.0 International License. This license lets the audience to give appropriate credit, provide a link to the license, and indicate if changes were made and if they remix, transform, or build upon the material, they must distribute contributions under the same license as the original.