An Approach to Prediction of Cardiovascular Diseases using Machine and Deep Learning Models
Keywords:
Healthcare, feature selection, Machine Learning, cardiovascular diseasesAbstract
In this article, we examined approximately 550 patient records in order to determine major risk variables that may be the root cause of cardiac issues. This study attempts to offer a piece of work that may be used as an instant step toward determining a probability assessment for the heart condition. The many risk factors are those that may be the major cause of the emergence of a cardiac condition. In this work, we examined several classification methods to diagnose the heart condition. The data was gathered from five separate Indian cities and also from people of all ages. We used the bidirectional LSTM model (BDLSTM), which was trained with experimental data from the source. The primary goal of this type of activity is to present a clear solution that will allow the patient to know statistically whether a heart problem is likely to occur. This answer is not a substitute for a healthcare professional, but rather a supplement to any doctor's diagnostic procedure. This look after transparency in the treatment of a physician and a patient. The model is evaluated based on both positives and negatives of false generated by model, and the best method for prediction is chosen. The results showed that two of these four algorithms were more accurate than 98.5% of the time.
Downloads
References
Abdalrada, A. S., Abawajy, J., Al-Quraishi, T., & Islam, S. M. S. (2022). Machine learning models for prediction of co-occurrence of diabetes and cardiovascular diseases: a retrospective cohort study. Journal of Diabetes & Metabolic Disorders, 21(1), 251–261. https://doi-org.proxy1.library.eiu.edu/10.1007/s40200-021-00968-z.
Ahishakiye, Emmanuel & Mwangi, Waweru & Muthoni, Petronilla & Nderu, Lawrence & Wario, Ruth. (2021). Comparative Performance of Machine Leaning Algorithms in Prediction of Cervical Cancer.
Cheddad, Abbas. (2020). Machine Learning in Healthcare.
Barbieri, S., Mehta, S., Wu, B., Bharat, C., Poppe, K., Jorm, L., & Jackson, R. (2022). Predicting cardiovascular risk from national administrative databases using a combined survival analysis and deep learning approach. International Journal of Epidemiology, 51(3), 931–944. https://doi-org.proxy1.library.eiu.edu/10.1093/ije/dyab258.3
Dorogush, Anna & Ershov, Vasily & Gulin, Andrey. (2018). CatBoost: gradient boosting with categorical features support. arXiv:1810.11363
Ed-daoudy, A., Maalmi, K. A new Internet of Things architecture for real-time prediction of various diseases using machine learning on big data environment. J Big Data 6, 104 (2019). https://doi.org/10.1186/s40537-019-0271-7.
Graves, Alex & Schmidhuber, Jürgen. (2005). Framewise phoneme classification with bidirectional LSTM and other neural network architectures. Neural networks: the official journal of the International Neural Network Society. 18. 602-10. 10.1016/j.neunet.2005.06.042.
Habehh, Hafsa & Gohel, Suril. (2021). Machine Learning In Healthcare. Current Genomics. 22. 10.2174/1389202922666210705124359.
Keto, Jaana & Ventola, Hanna & Jokelainen, Jari & Linden, Kari & Keinänen-Kiukaanniemi, Sirkka & Timonen, Markku & Ylisaukko-oja, Tero & Auvinen, Juha. (2016). Cardiovascular disease risk factors in relation to smoking behaviour and history: a population-based cohort study. Open Heart. 3. e000358. 10.1136/openhrt-2015-000358.
Olokoba, Abdulfatai & Olusegun, Obateru & Lateefat, Olokoba. (2012). Type 2 Diabetes Mellitus: A Review of Current Trends. Oman medical journal. 27. 269-73. 10.5001/omj.2012.68.
Puaschunder, Julia. (2020). The Potential for Artificial Intelligence in Healthcare. SSRN Electronic Journal. 10.2139/ssrn.3525037.
Li, Bin & Yu, Qingzhao & Peng, Lu. (2019). Ensemble of fast learning stochastic gradient boosting. Communications in Statistics - Simulation and Computation. 1-13. 10.1080/03610918.2019.1645170.
Friedman, Jerome. (2002). Stochastic Gradient Boosting. Computational Statistics & Data Analysis. 38. 367-378. 10.1016/S0167-9473(01)00065-2.
Rustam, F., Ishaq, A., Munir, K., Almutairi, M., Aslam, N., & Ashraf, I. (2022). Incorporating CNN Features for Optimizing Performance of Ensemble Classifier for Cardiovascular Disease Prediction. Diagnostics (2075-4418), 12(6), 1474. https://doi-org.proxy1.library.eiu.edu/10.3390/diagnostics12061474.
Sidey-Gibbons, A. M. Jenni, and C. J. Sidey-Gibbons, “Machine learning in medicine: a practical introduction,” BMC Medical Research Methodology, vol. 19, 2019.
Sung, J. M., Cho, I.-J., Sung, D., Kim, S., Kim, H. C., Chae, M.-H., Kavousi, M., Rueda-Ochoa, O. L., Ikram, M. A., Franco, O. H., & Chang, H.-J. (2019). Development and verification of prediction models for preventing cardiovascular diseases. PLoS ONE, 14(9), 1–12. https://doi-org.proxy1.library.eiu.edu/10.1371/journal.pone.0222809.
Tekkeşin, Ahmet. (2019). Artificial Intelligence in Healthcare: Past, Present and Future. The Anatolian Journal of Cardiology. 22. 10.14744/AnatolJCardiol.2019.28661.
The Top 10 Causes of Death. Available online: https://www.who.int/news-room/fact-sheets/detail/the-top-10-causes-of-death (accessed on 31 May 2021).
Thireou, Trias & Reczko, Martin. (2007). Bidirectional Long Short-Term Memory Networks for Predicting the Subcellular Localization of Eukaryotic Proteins. IEEE/ACM transactions on computational biology and bioinformatics / IEEE, ACM. 4. 441-6. 10.1109/tcbb.2007.1015.
Xie, J., Wu, R., Wang, H., Chen, H., Xu, X., Kong, Y., & Zhang, W. (2021). Prediction of cardiovascular diseases using weight learning based on density information. Neurocomputing, 452, 566–575. https://doi-org.proxy1.library.eiu.edu/10.1016/j.neucom.2020.10.114.
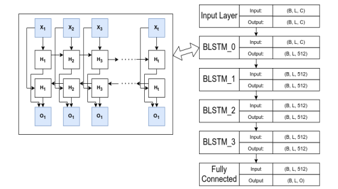
Downloads
Published
How to Cite
Issue
Section
License
Copyright (c) 2022 Bhagyalaxmi Singirikonda, Muktevi Srivenkatesh

This work is licensed under a Creative Commons Attribution-ShareAlike 4.0 International License.
All papers should be submitted electronically. All submitted manuscripts must be original work that is not under submission at another journal or under consideration for publication in another form, such as a monograph or chapter of a book. Authors of submitted papers are obligated not to submit their paper for publication elsewhere until an editorial decision is rendered on their submission. Further, authors of accepted papers are prohibited from publishing the results in other publications that appear before the paper is published in the Journal unless they receive approval for doing so from the Editor-In-Chief.
IJISAE open access articles are licensed under a Creative Commons Attribution-ShareAlike 4.0 International License. This license lets the audience to give appropriate credit, provide a link to the license, and indicate if changes were made and if they remix, transform, or build upon the material, they must distribute contributions under the same license as the original.