An uncertain Liver Segmentation in Computed Tomography Images with Enhanced generative Mask Region on CNN
Keywords:
liver segmentation, CNN, uncertain dataset, CT scanAbstract
The ability to measure liver volume with a single device has emerged in medical practice. Images from CT & MRI are used to estimate the liver's total volume. After the liver and its peripherals are scanned using abdominal computed tomography, the PC-aided conclusion and remedial intervention may begin. This research presents a self-loader division technique based on the specific multivariable appropriation of Tissues in the Liver & sub-divisions of the graph cut. While it isn't wholly mechanized, the process negligibly includes human communications. Unequivocally, it comprises three primary stages. A subject-explicit probabilistic model, first and foremost, was worked from an inside fix encompassing a seed point determined by the client. Furthermore, a repeated task of pixel marks has been implemented to refresh the probabilistic guide of the tissues in light of spatial-logical data. The chart slice model was later upgraded to extricate the 3-dimensional Liver from the picture. In the post-handling, excessively fragmented nodal areas because of fluffy tissue division were eliminated, keeping up with their suitable life structures utilizing hearty bottleneck location with adjoining form imperatives. The proposed framework was carried out and approved on the MICCAI SLIVER07 dataset. Results were compared to cutting-edge techniques in light of critical clinical metrics. The visual and quantitative evaluations thus suggest that the proposed framework might function on the accuracy and dependability of asymptomatic liver division. Increasingly, the liver image division is being used for essential clinical objectives, such as liver evaluation, disease diagnosis, and therapy. Using GANs and veils local convolutional brain organizations, this study provides a liver picture division method (Mask R-CNN). We first looked at the combination of Mask R-CNN and GANs to improve pixel-wise characterization since that's how most incoming images contain uproarious parts. A GAN Mask R-CNN calculation was finally presented, and it outperformed the standard Mask R-CNN and Mask CNN calculations in terms of exhibition analysis. This includes measures for the Dice similitude coefficient (DSC) and MICCAI measurements. The proposed calculation additionally accomplished better execution analysis than ten advanced calculations concerning six Boolean markers.
Downloads
References
Girshick, R. (2015). Fast r-cnn. In Proceedings of the IEEE international conference on computer vision (pp. 1440-1448).
J. Jin, S., Su, Y., Gao, S., Wu, F., Hu, T., Liu, J., ... & Guo, Q. (2018). Deep learning: individual maize segmentation from terrestrial lidar data using faster R-CNN and regional growth algorithms. Frontiers in plant science, 9, 866..
Bürgel, U., Mädler, B., Honey, C. R., Thron, A., Gilsbach, J., & Coenen, V. A. (2009). Fiber tracking with distinct software tools results in a clear diversity in anatomical fiber tract portrayal. Central European Neurosurgery-Zentralblatt für Neurochirurgie, 70(01), 27-35.
Virzì, A., Muller, C. O., Marret, J. B., Mille, E., Berteloot, L., Grévent, D., ... & Bloch, I. (2020). Comprehensive review of 3D segmentation software tools for MRI usable for pelvic surgery planning. Journal of digital imaging, 33(1), 99-110.
Ananth, C., & Jain, R. (2020). Enhancing Segmentation Approaches from Oaam to Fuzzy KC-Means. The Journal of Research on the Lepidoptera, 51(2), 1086-1108.
Sanai, F. M., & Bzeizi, K. I. (2005). Systematic review: tuberculous peritonitis–presenting features, diagnostic strategies and treatment. Alimentary pharmacology & therapeutics, 22(8), 685-700.
Wang, K., Lu, X., Zhou, H., Gao, Y., Zheng, J., Tong, M., ... & Zheng, R. (2019). Deep learning Radiomics of shear wave elastography significantly improved diagnostic performance for assessing liver fibrosis in chronic hepatitis B: a prospective multicentre study. Gut, 68(4), 729-741.
Loffroy, R., Favelier, S., Chevallier, O., Estivalet, L., Genson, P. Y., Pottecher, P., ... & Cercueil, J. P. (2015). Preoperative portal vein embolization in liver cancer: indications, techniques and outcomes. Quantitative imaging in medicine and surgery, 5(5), 730. [9] L. Lenchik, L., Heacock, L., Weaver, A. A., Boutin, R. D., Cook, T. S., Itri, J., ... & Narayana, P. A. (2019). Automated segmentation of tissues using CT and MRI: a systematic review. Academic radiology, 26(12), 1695-1706.
Guo, X., Schwartz, L. H., & Zhao, B. (2019). Automatic liver segmentation by integrating fully convolutional networks into active contour models. Medical physics, 46(10), 4455-4469.
Lu, F., Wu, F., Hu, P., Peng, Z., & Kong, D. (2017). Automatic 3D liver location and segmentation via convolutional neural network and graph cut. International journal of computer assisted radiology and surgery, 12(2), 171-182.
Saikumar, K., Rajesh, V., Babu, B.S. (2022). Heart disease detection based on feature fusion technique with augmented classification using deep learning technology. Traitement du Signal, Vol. 39, No. 1, pp. 31-42. https://doi.org/10.18280/ts.390104 .
Kailasam, S., Achanta, S.D.M., Rama Koteswara Rao, P., Vatambeti, R., Kayam, S. (2022). An IoT-based agriculture maintenance using pervasive computing with machine learning technique. International Journal of Intelligent Computing and Cybernetics, 15(2), pp. 184–197.
Saikumar, K. (2020). RajeshV. Coronary blockage of artery for Heart diagnosis with DT Artificial Intelligence Algorithm. Int J Res Pharma Sci, 11(1), 471-479.
Saikumar, K., Rajesh, V. (2020). A novel implementation heart diagnosis system based on random forest machine learning technique International Journal of Pharmaceutical Research 12, pp. 3904-3916.
Raju K., Chinna Rao B., Saikumar K., Lakshman Pratap N. (2022) An Optimal Hybrid Solution to Local and Global Facial Recognition Through Machine Learning. In: Kumar P., Obaid A.J., Cengiz K., Khanna A., Balas V.E. (eds) A Fusion of Artificial Intelligence and Internet of Things for Emerging Cyber Systems. Intelligent Systems Reference Library, vol 210. Springer, Cham. https://doi.org/10.1007/978-3-030-76653-5_11
Sankara Babu B., Nalajala S., Sarada K., Muniraju Naidu V., Yamsani N., Saikumar K. (2022) Machine Learning Based Online Handwritten Telugu Letters Recognition for Different Domains. In: Kumar P., Obaid A.J., Cengiz K., Khanna A., Balas V.E. (eds) A Fusion of Artificial Intelligence and Internet of Things for Emerging Cyber Systems. Intelligent Systems Reference Library, vol 210. Springer, Cham. https://doi.org/10.1007/978-3-030-76653-5_12.
Kiran Kumar M., Kranthi Kumar S., Kalpana E., Srikanth D., Saikumar K. (2022) A Novel Implementation of Linux Based Android Platform for Client and Server. In: Kumar P., Obaid A.J., Cengiz K., Khanna A., Balas V.E. (eds) A Fusion of Artificial Intelligence and Internet of Things for Emerging Cyber Systems. Intelligent Systems Reference Library, vol 210. Springer, Cham. https://doi.org/10.1007/978-3-030-76653-5_8.
Shravani, C., Krishna, G. R., Bollam, H. L., Vatambeti, R., & Saikumar, K. (2022, January). A Novel Approach for Implementing Conventional LBIST by High Execution Microprocessors. In 2022 4th International Conference on Smart Systems and Inventive Technology (ICSSIT) (pp. 804-809). IEEE.
Rao, K. S., Reddy, B. V., Sarada, K., & Saikumar, K. (2021). A Sequential Data Mining Technique for Identification of Fault Zone Using FACTS-Based Transmission. In Handbook of Research on Innovations and Applications of AI, IoT, and Cognitive Technologies (pp. 408-419). IGI Global..
Raju, K., Pilli, S. K., Kumar, G. S. S., Saikumar, K., & Jagan, B. O. L. (2019). Implementation of natural random forest machine learning methods on multi spectral image compression. Journal of Critical Reviews, 6(5), 265-273.
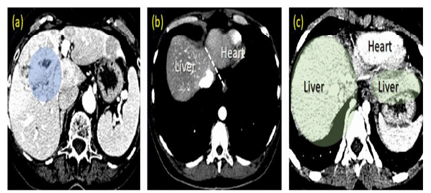
Downloads
Published
How to Cite
Issue
Section
License

This work is licensed under a Creative Commons Attribution-ShareAlike 4.0 International License.
All papers should be submitted electronically. All submitted manuscripts must be original work that is not under submission at another journal or under consideration for publication in another form, such as a monograph or chapter of a book. Authors of submitted papers are obligated not to submit their paper for publication elsewhere until an editorial decision is rendered on their submission. Further, authors of accepted papers are prohibited from publishing the results in other publications that appear before the paper is published in the Journal unless they receive approval for doing so from the Editor-In-Chief.
IJISAE open access articles are licensed under a Creative Commons Attribution-ShareAlike 4.0 International License. This license lets the audience to give appropriate credit, provide a link to the license, and indicate if changes were made and if they remix, transform, or build upon the material, they must distribute contributions under the same license as the original.