A Framework for Extracted Data from Social Networking Sites in Addressing the Cross-Site Cold-Start Product Recommendation
Keywords:
Social Networks, Deep Learning, Product Recommendation, Cross-Site Cold-Start, FeaturesAbstract
With rise of online social networks, social network-based proposal approach is prevalently utilized. Significant advantage of this method is capacity of managing the issues with cold-start clients. Notwithstanding social networks, client trust data likewise assumes a significant part to acquire dependable proposals. Deep learning (DL) has drawn in expanding consideration by virtue of its huge handling power in errands, like discourse, picture, or text handling. This research propose novel technique in social networking site data based product recommendation for cross-site cold-startusing deep learning techniques. Here the input data has been collected as social networking data with addressing of cross-site cold-startproducts. The input data has been processed for noise removal, smoothening and normalization. The processed data features has been extracted using deep convolutional capsulenet neural network. The experimental analysis has been carried out in terms of accuracy, precision, recall, F-1 score, MAP, RMSE. We perform broad trials on certifiable informal community information to exhibit the precision and viability of our proposed approach in correlation with other cutting edge strategies .The proposed technique attained accuracy of 93%, precision of 91%, recall of 85%, F-1 score of 80%, MAP of 52%, RMSE of 55%.
Downloads
References
Gong, Q., Chen, Y., He, X., Xiao, Y., Hui, P., Wang, X., & Fu, X. (2021). Cross-site prediction on social influence for cold-start users in online social networks. ACM Transactions on the Web (TWEB), 15(2), 1-23.
Abdullah, N. A., Rasheed, R. A., Nasir, M. H. N. M., &Rahman, M. M. (2021). Eliciting auxiliary information for cold start user recommendation: A survey. Applied Sciences, 11(20), 9608.
Amara, A., Taieb, M. A. H., &Aouicha, M. B. (2022). Cross-social networks analysis: building me-edge centered BUNet dataset based on implicit bridge users. Online Information Review, (ahead-of-print).
Shao, Y., & Liu, C. (2021). H2Rec: Homogeneous and Heterogeneous Network Embedding Fusion for Social Recommendation. International Journal of Computational Intelligence Systems, 14(1), 1303-1314.
Ji, Z., Wu, M., Yang, H., &Íñigo, J. E. A. (2021). Temporal sensitive heterogeneous graph neural network for news recommendation. Future Generation Computer Systems, 125, 324-333.
Zang, T., Zhu, Y., Liu, H., Zhang, R., & Yu, J. (2021). A survey on cross-domain recommendation: taxonomies, methods, and future directions. arXiv preprint arXiv:2108.03357.
Wang, W. (2022). Application of E-Commerce Recommendation Algorithm in Consumer Preference Prediction. Journal of Cases on Information Technology (JCIT), 24(5), 1-28.
Liu, J., Pan, B., Zhang, X., & Li, D. (2021). Mobile E-commerce information system based on industry cluster under edge computing. Mobile Information Systems, 2021.
Ayala, C., Jiménez, K., Loza-Aguirre, E., & Andrade, R. O. (2021). A Hybrid Recommender for Cybersecurity Based on Rating Approach. In Advances in Cybersecurity Management (pp. 445-462). Springer, Cham.
Gupta, C., Jain, A., Castillo, O., & Joshi, N. (2021). A Scientometric Analysis of Transient Patterns in Recommender Systems with Soft Computing Techniques. Computación y Sistemas, 25(1), 193-221.
Ovaisi, Z., Heinecke, S., Li, J., Zhang, Y., Zheleva, E., &Xiong, C. (2022, February). RGRecSys: A Toolkit for Robustness Evaluation of Recommender Systems. In Proceedings of the Fifteenth ACM International Conference on Web Search and Data Mining (pp. 1597-1600).
Himeur, Y., Sohail, S. S., Bensaali, F., Amira, A., &Alazab, M. (2022). Latest Trends of Security and Privacy in Recommender Systems: A Comprehensive Review and Future Perspectives. Computers & Security, 102746.
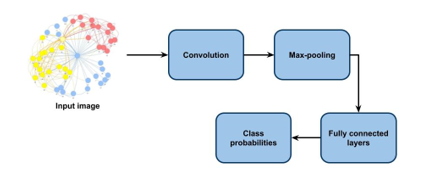
Downloads
Published
How to Cite
Issue
Section
License
Copyright (c) 2022 Alka Kumari, KDV Prasad, C. Sushama, Archana P. Kale, Revati M. Wahul, Shrddha Sagar

This work is licensed under a Creative Commons Attribution-ShareAlike 4.0 International License.
All papers should be submitted electronically. All submitted manuscripts must be original work that is not under submission at another journal or under consideration for publication in another form, such as a monograph or chapter of a book. Authors of submitted papers are obligated not to submit their paper for publication elsewhere until an editorial decision is rendered on their submission. Further, authors of accepted papers are prohibited from publishing the results in other publications that appear before the paper is published in the Journal unless they receive approval for doing so from the Editor-In-Chief.
IJISAE open access articles are licensed under a Creative Commons Attribution-ShareAlike 4.0 International License. This license lets the audience to give appropriate credit, provide a link to the license, and indicate if changes were made and if they remix, transform, or build upon the material, they must distribute contributions under the same license as the original.