Elastic Optical Networks Based Optimization Using Machine Learning: State-Of-Art Review
Keywords:
Elastic optical networks, data transmission line, link monitoring, Machine learning, link provisioningAbstract
To address the issues and difficulties relating to network capacity, elastic optical networks have been developed. Elastic optical networks have been regarded as being primarily enabled by software and hardware resource optimization (EONs). Several Tb/s long-distance data transmission lines can benefit from elastic optical networks' improved spectral efficiency. Elastic optical networks now use machine learning (ML) approaches to facilitate automated processes and unlock more physical layer capacity. One drawback is that training environment—such as traffic pattern or structure of optical network—has a significant impact on how much is learned. Retraining is therefore necessary in cases of network topology or traffic pattern changes, which requires a lot of processing power and time. We examine and debate several ML applications in connection modelling, provisioning, and network control.
Downloads
References
Aibin, M. (2018). Traffic prediction based on machine learning for elastic optical networks. Optical Switching and Networking, 30, 33-39.
Luo, X., Shi, C., Wang, L., Chen, X., Li, Y., & Yang, T. (2019). Leveraging double-agent-based deep reinforcement learning to global optimization of elastic optical networks with enhanced survivability. Optics express, 27(6), 7896-7911.
Salani, M., Rottondi, C., Ceré, L., & Tornatore, M. (2022). Dual-Stage Planning for Elastic Optical Networks Integrating Machine-Learning-Assisted QoT Estimation. IEEE/ACM Transactions on Networking.
Liu, X., Lun, H., Fu, M., Fan, Y., Yi, L., Hu, W., & Zhuge, Q. (2020). AI-based modeling and monitoring techniques for future intelligent elastic optical networks. Applied Sciences, 10(1), 363.
Tian, X., Li, B., Gu, R., & Zhu, Z. (2021). Reconfiguring multicast sessions in elastic optical networks adaptively with graph-aware deep reinforcement learning. Journal of Optical Communications and Networking, 13(11), 253-265.
Tang, B., Huang, Y. C., Xue, Y., & Zhou, W. (2022). Deep Reinforcement Learning-Based RMSA Policy Distillation for Elastic Optical Networks. Mathematics, 10(18), 3293.
Zhang, J., Qian, F., & Yang, J. (2022). Online routing and spectrum allocation in elastic optical networks based on dueling Deep Q-network. Computers & Industrial Engineering, 173, 108663.
Tang, B., Huang, Y. C., Xue, Y., & Zhou, W. (2022). Heuristic reward design for deep reinforcement learning-based routing, modulation and spectrum assignment of elastic optical networks. IEEE Communications Letters, 26(11), 2675-2679.
Pinto-Ríos, J., Calderón, F., Leiva, A., Hermosilla, G., Beghelli, A., Bórquez-Paredes, D., ... & Saavedra, G. (2022). Resource Allocation in Multicore Elastic Optical Networks: A Deep Reinforcement Learning Approach. arXiv preprint arXiv:2207.02074.
Mukherjee, A., & Choudhury, P. D. (2022). Recent Developments on Elastic Optical Networks: A brief Survey. American Journal of Electronics & Communication, 3(2), 16-20.
Trindade, S., Torres, R. D. S., Zhu, Z., & Fonseca, N. L. D. (2021). Cognitive control-loop for elastic optical networks with space-division multiplexing. Sensors, 21(23), 7821.
Agarwal, A., Misra, G., & Agarwal, K. (2022). A Review and Analysis on Elastic Optical Networks (EONs): Concepts, Recent Developments and Research Challenges. Journal of The Institution of Engineers (India): Series B, 1-6.
Zhu, R., Samuel, A., Wang, P., Li, S., Oun, B. K., Li, L., ... & Yu, S. (2021). Protected resource allocation in space division multiplexing-elastic optical networks with fluctuating traffic. Journal of Network and Computer Applications, 174, 102887.
Li, R., Gu, R., Jin, W., & Ji, Y. (2021). Learning-based cognitive hitless spectrum defragmentation for dynamic provisioning in elastic optical networks. IEEE Communications Letters, 25(5), 1600-1604.
Tang, F., Shen, G., & Rouskas, G. N. (2021). Crosstalk-aware shared backup path protection in multi-core fiber elastic optical networks. Journal of Lightwave Technology, 39(10), 3025-3036.
Aibin, M., & Walkowiak, K. (2018, August). Monte Carlo tree search for cross-stratum optimization of survivable inter-data center elastic optical network. In 2018 10th International Workshop on Resilient Networks Design and Modeling (RNDM) (pp. 1-7). IEEE.
Zhu, R., Li, S., Wang, P., & Yuan, J. (2020). Time and spectrum fragmentation-aware virtual optical network embedding in elastic optical networks. Optical Fiber Technology, 54, 102117.
Yao, Q., Yang, H., Bao, B., Yu, A., Zhang, J., & Cheriet, M. (2021). Core and spectrum allocation based on association rules mining in spectrally and spatially elastic optical networks. IEEE Transactions on Communications, 69(8), 5299-5311.
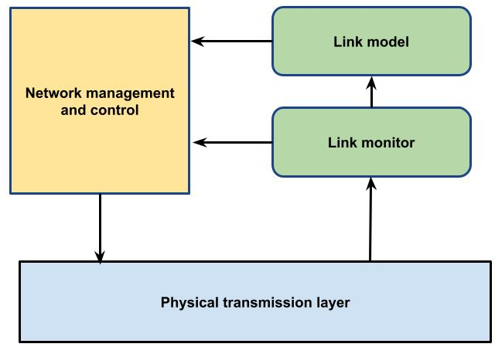
Downloads
Published
How to Cite
Issue
Section
License
Copyright (c) 2023 Ruchi G. Dave, Dolly Thankachan

This work is licensed under a Creative Commons Attribution-ShareAlike 4.0 International License.
All papers should be submitted electronically. All submitted manuscripts must be original work that is not under submission at another journal or under consideration for publication in another form, such as a monograph or chapter of a book. Authors of submitted papers are obligated not to submit their paper for publication elsewhere until an editorial decision is rendered on their submission. Further, authors of accepted papers are prohibited from publishing the results in other publications that appear before the paper is published in the Journal unless they receive approval for doing so from the Editor-In-Chief.
IJISAE open access articles are licensed under a Creative Commons Attribution-ShareAlike 4.0 International License. This license lets the audience to give appropriate credit, provide a link to the license, and indicate if changes were made and if they remix, transform, or build upon the material, they must distribute contributions under the same license as the original.