Patient-Specific Brain Tumor Segmentation using Hybrid Ensemble Classifier to Extract Deep Features
Keywords:
tumor, texture, deep features, classifierAbstract
The abnormal cell development in the Brain is referred to as a tumor. Brain tumors are treated by physicians using radiation and Surgery. The brain tumor is categorized as benign or malignant. The benign tumor can be treated and cured using the appropriate medication suggested. A malignant tumor is an abnormal tissue that affects nearby tissues and can be cured only through proper Surgery by a physician. Manual identification of malignant and benign tumors is a time-consuming and error-prone process. An automatic brain tumor classification technique is proposed to overcome the limitation. An efficient methodology for the detection of brain tumors is done. Initially, the brain MRI image is smoothed and enhanced by a Gaussian filter. Then deep and texture features are extracted. The proposed work uses an ensemble technique using three different classifiers based on Majority Voting Method. The specified method is tested on BRATS 2017 and 2018 datasets. The results are compared with recent methods and prove efficacy.
Downloads
References
Ayşe Demirhan, Mustafa Törü&İnanGüler 2015, ‘Segmentation of tumor and edema along with healthy tissues of the brain using wavelets and neural networks', IEEE Journal of Biomedical and Health Informatics, vol. 19, no. 4, pp. 1451-1458.
Meier, R Stefan Bauer, Johannes Slotboom, Roland Wiest, Mauricio Reyes, et al., 2014, 'Patient-specific semi-supervised learning for postoperative brain tumor segmentation', in Medical Image Computing and Computer Assisted Intervention MICCAI Springer, pp. 714-721.
Rajaguru, H, KarthickGanesan&Vinoth Kumar Bojan 2016, ‘Earlier detection of cancer regions from MR image features and SVM classifiers’, International Journal of Imaging Systems and Technology, vol. 26, no. 3, pp. 196-208.
Viswa Priya, V 2016, ‘Segmentation in MRI’, Indian Journal of Science and Technology, vol. 9, no. 19.
Rajesh Chandra, G, KolasaniRamchand&Rao, H 2016, ‘Tumor detection in brain using genetic algorithm’, Procedia Computer Science, vol. 79, pp. 449-457.
Isselmou, A, Zhang, S &Xu, G 2016, ‘A novel approach for brain tumor detection using MRI images’, Journal of Biomedical Science and Engineering, vol. 9, pp. 44-52.
Ursula Perez, Estanislao Arana & David Moratal 2016, ‘Brain metastases detection algorithms in magnetic resonance imaging’, IEEE Latin America Transactions, vol. 14, no. 3, pp. 1109-1114.
V. Shreyas, and Vinod Pankajakshan,” A Deep Learning Architecture for Brain Tumor Segmentation in MRI Images”, IEEE 2017.
M. Havaei, A. Davy, David Warde-Farley, Antoine Biard, Aaron Courvill, Yoshua Bengio, Chris Pal, Pierre-Marc Jodoin, Hugo Larochelle, “Brain tumor segmentation with deep neural networks,” Medical Image Analysis, vol. 35, pp. 18 – 31, 2017.
S. Pereira, A. Pinto Pereira, Adriano Pinto, Victor Alves, and Carlos A. Silva, "Brain tumor segmentation using convolutional neural networks in MRI images," IEEE Transactions on Medical Imaging, vol. 35, no. 5, pp. 1240–1251, 5 2016.
K. Kamnitsas, W. Bai, E. Ferrante, S. McDonagh, M. Sinclair, N. Pawlowski, M. Rajchl, M. Lee, B. Kainz, D. Rueckert, B. Glocker, "Ensembles of Multiple Models and Architectures for Robust Brain Tumour Segmentation" Brainlesion: Glioma, Multiple Sclerosis, Stroke, and Traumatic Brain Injuries. BrainLes 2017. Lecture Notes in Computer Science, vol 10670. Springer, Cham
S. Bonte, R. Van Holen, I. Goethals; “Brain tumour segmentation on contrast enhanced T1w MRI using local texture and Random Forests”, Gent/BE, European Society of Radiology, 2018, pp. 1-8
Harendra Sharma, H.S. Bhadauria, “An Effective Approach on Brain Tumor Segmentation with Polynomial Hybrid Technique”, IEEE 2017
Mir Khadiza Akter, Shakil Mahmud Khan, Samee Azad and Shaikh Anowarul Fattah, "Automated Brain Tumor Segmentation from MRI Data Based on Exploration of Histogram Characteristics of the Cancerous Hemisphere", 2017 IEEE Region 10 Humanitarian Technology Conference (R10-HTC), pp.815-818
Wei Chen, Xu Qiao, Boqiang Liu, Xianying Qi, Rui Wang, Xiaoya Wang, “Automatic Brain Tumor Segmentation Based on Features of Separated Local Square”, IEEE, 2017, pp. 6489-6493
Najeebullah Shah, Sheikh Ziauddin, Ahmad R. Shahid, “Brain Tumor Segmentation and Classification using Cascaded Random Decision Forests” 2017 14th International Conference on Electrical Engineering/Electronics, Computer, Telecommunications and Information Technology
Rehman, A., Khan, M.A., Saba, T., Mehmood, Z., Tariq, U. and Ayesha, N., 2021. Microscopic brain tumor detection and classification using 3D CNN and feature selection architecture. Microscopy Research and Technique, 84(1), pp.133-149.
Khan, M. A., Ashraf, I., Alhaisoni, M., Damaševicˇius, R., Scherer, R., Rehman, A., & Bukhari, S. A. C. (2020). Multimodal brain tumor classification using deep learning and robust feature selection: A machine learning application for radiologists. Diagnostics, 10, 565.
Sharif, M. I., Li, J. P., Khan, M. A., & Saleem, M. A. (2020). Active deep neural network features selection for segmentation and recognition of brain tumors using MRI images. Pattern Recognition Letters, 129, 181–189
Saba, T., Mohamed, A.S., El-Affendi, M., Amin, J. and Sharif, M., 2020. Brain tumor detection using fusion of hand crafted and deep learning features. Cognitive Systems Research, 59, pp.221-230.
Amin, J., Sharif, M., Yasmin, M. and Fernandes, S.L., 2020. A distinctive approach in brain tumor detection and classification using MRI. Pattern Recognition Letters, 139, pp.118-127.
Aboussaleh, I., Riffi, J., Mahraz, A.M. and Tairi, H., 2021. Brain Tumor Segmentation Based on Deep Learning’s Feature Representation. Journal of Imaging, 7(12), p.269.
Ranjbarzadeh, R., Kasgari, A.B., Ghoushchi, S.J., Anari, S., Naseri, M. and Bendechache, M., 2021. Brain tumor segmentation based on deep learning and an attention mechanism using MRI multi-modalities brain images. Scientific Reports, 11(1), pp.1-17.
Liu, P., Dou, Q., Wang, Q. and Heng, P.A., 2020. An encoder-decoder neural network with 3D squeeze-and-excitation and deep supervision for brain tumor segmentation. IEEE Access, 8, pp.34029-34037.
Wang, G.; Li, W.; Ourselin, S.; Vercauteren, T. Automatic brain tumor segmentation using cascaded anisotropic convolutional neural networks. In International MICCAI Brainlesion Workshop; Springer: Cham, Switzerland, 2017; pp. 178–190.
Myronenko, A. 3D MRI brain tumor segmentation using autoencoder regularization. In International MICCAI Brainlesion Workshop; Springer: Cham, Switzerland, 2018; pp. 311–320. 27. Nema, S.; Dudhane, A.; Murala, S.; Naidu, S. RescueNet: An unpaired GAN for brain tumor segmentation. Biomed. Signal Process. Control 2020, 55, 101641
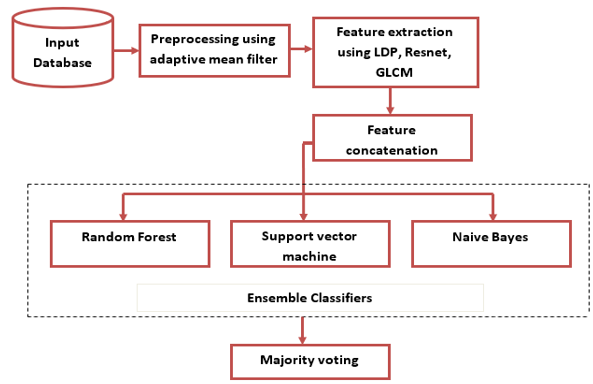
Downloads
Published
How to Cite
Issue
Section
License

This work is licensed under a Creative Commons Attribution-ShareAlike 4.0 International License.
All papers should be submitted electronically. All submitted manuscripts must be original work that is not under submission at another journal or under consideration for publication in another form, such as a monograph or chapter of a book. Authors of submitted papers are obligated not to submit their paper for publication elsewhere until an editorial decision is rendered on their submission. Further, authors of accepted papers are prohibited from publishing the results in other publications that appear before the paper is published in the Journal unless they receive approval for doing so from the Editor-In-Chief.
IJISAE open access articles are licensed under a Creative Commons Attribution-ShareAlike 4.0 International License. This license lets the audience to give appropriate credit, provide a link to the license, and indicate if changes were made and if they remix, transform, or build upon the material, they must distribute contributions under the same license as the original.