Hybrid Auto Encoder for Dimensionality Reduction in Ct Scan Images of Heart
Keywords:
De-noising Encoder, Convolution Neural Network, Sensors, Decoder, Identity FunctionAbstract
Modern style of food habit system and stress life has completely impacted the human life. Sudden deaths irrespective of age have become most common now days. In order to save human life, predict heart attack system many of the existing systems are working on the textual attributes but in the proposed model, the major focus of the heart attack prediction is based on the CT SCAN, continuous monitoring of which helps the doctors to diagnosis the patients effectively. The proposed model along with the identification of the heart attack, it also analyzes the heart beat rate time to time based on the sensor. This analysis helps the doctor to recognize the part that is blocked and that makes the patient to suffer from cardiac arrest or survival problems in future. Traditional approaches use either principal component analysis or neural networks for identifying those parts but the proposed model extends the de-noising auto encoder that has the capability to prevent similar identity learning features. The model has achieved +3.1% accuracy improvements when compared to the traditional CNN.
Downloads
References
Diniz, J. O. B., Ferreira, J. L., Cortes, O. A. C., Silva, A. C., & de Paiva, A. C. (2022). An automatic approach for heart segmentation in CT scans through image processing techniques and Concat-U-Net. In Expert Systems with Applications (Vol. 196, p. 116632). Elsevier BV. https://doi.org/10.1016/j.eswa.2022.116632
Sharobeem, S., Le Breton, H., Lalys, F. et al. Validation of a Whole Heart Segmentation from Computed Tomography Imaging Using a Deep-Learning Approach. J. of Cardiovasc. Trans. Res. 15, 427–437 (2022). https://doi.org/10.1007/s12265-021-10166-0
Li, F., Sun, L., Lam, K.-Y., Zhang, S., Sun, Z., Peng, B., Xu, H., & Zhang, L. (2022). Segmentation of human aorta using 3D nnU-net-oriented deep learning. In Review of Scientific Instruments (Vol. 93, Issue 11, p. 114103). AIP Publishing. https://doi.org/10.1063/5.0084433
Nainamalai, V., Lippert, M., Brun, H., Elle, O. J., & Kumar, R. P. (2022). Local integration of deep learning for advanced visualization in congenital heart disease surgical planning. In Intelligence-Based Medicine (Vol. 6, p. 100055). Elsevier BV.
Pieszko, K., Shanbhag, A., Killekar, A., Miller, R. J. H., Lemley, M., Otaki, Y., Singh, A., Kwiecinski, J., Gransar, H., Van Kriekinge, S. D., Kavanagh, P. B., Miller, E. J., Bateman, T., Liang, J. X., Berman, D. S., Dey, D., & Slomka, P. J. (2022). Deep Learning of Coronary Calcium Scores From PET/CT Attenuation Maps Accurately Predicts Adverse Cardiovascular Events. In JACC: Cardiovascular Imaging. Elsevier BV.
Reddy, A. M., Reddy, K. S., Jayaram, M., Venkata Maha Lakshmi, N., Aluvalu, R., Mahesh, T. R., Kumar, V. V., & Stalin Alex, D. (2022). An Efficient Multilevel Thresholding Scheme for Heart Image Segmentation Using a Hybrid Generalized Adversarial Network. In S. Bhattacharya (Ed.), Journal of Sensors (Vol. 2022, pp. 1–11). Hindawi Limited.
Sri Silpa Padmanabhuni and Pradeepini Gera, “Synthetic Data Augmentation of Tomato Plant Leaf using Meta Intelligent Generative Adversarial Network: Milgan” International Journal of Advanced Computer Science and Applications(IJACSA), 13(6), 2022.
SRI SILPAPADMANABHUNI SRINIVASA REDDY K, P. VENKATESWARA RAO, A.MALLIKARJUNA REDDY, K SUDHEER REDDY, DR. J. LAKSHMI NARAYANA "NEURAL NETWORK AIDED OPTIMIZED AUTO ENCODER AND DECODER FOR DETECTION OF COVID-19 AND PNEUMONIA USING CT-SCAN" Journal of Theoretical and Applied Information Technology,15th November 2022. Vol.100. No 21,pp.no.6346-6360, 2022.
Al-Absi, Hamada R. H., Mohammad Tariqul Islam, Mahmoud Ahmed Refaee, Muhammad E. H. Chowdhury, and Tanvir Alam. 2022. "Cardiovascular Disease Diagnosis from DXA Scan and Retinal Images Using Deep Learning" Sensors 22, no. 12: 4310.
Song, Y., Ren, S., Lu, Y., Fu, X., & Wong, K. K. (2022). Deep learning-based automatic segmentation of images in cardiac radiography: A promising challenge. Computer Methods and Programs in Biomedicine, 220, 106821.
Sajja, T.K., Kalluri, H.K. (2020). A deep learning method for prediction of cardiovascular disease using convolutional neural network. Revue d'Intelligence Artificielle, Vol. 34, No. 5, pp. 601-606.
Chen, Zhennong. "Analysis of Cardiovascular Disease using Cardiac Computed Tomography and Deep Learning." Ph.D. diss., UC San Diego, 2022.
Papandrianos, Nikolaos, and Elpiniki Papageorgiou. 2021. "Automatic Diagnosis of Coronary Artery Disease in SPECT Myocardial Perfusion Imaging Employing Deep Learning" Applied Sciences 11, no. 14: 6362. https://doi.org/10.3390/app11146362
Maragatham, G., & Devi, S. (2019). LSTM Model for Prediction of Heart Failure in Big Data. Journal of Medical Systems, 43(5).
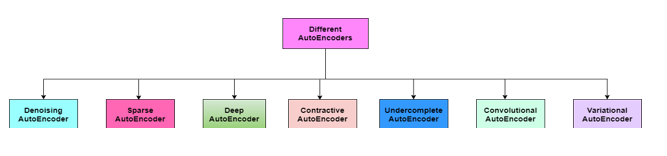
Downloads
Published
How to Cite
Issue
Section
License

This work is licensed under a Creative Commons Attribution-ShareAlike 4.0 International License.
All papers should be submitted electronically. All submitted manuscripts must be original work that is not under submission at another journal or under consideration for publication in another form, such as a monograph or chapter of a book. Authors of submitted papers are obligated not to submit their paper for publication elsewhere until an editorial decision is rendered on their submission. Further, authors of accepted papers are prohibited from publishing the results in other publications that appear before the paper is published in the Journal unless they receive approval for doing so from the Editor-In-Chief.
IJISAE open access articles are licensed under a Creative Commons Attribution-ShareAlike 4.0 International License. This license lets the audience to give appropriate credit, provide a link to the license, and indicate if changes were made and if they remix, transform, or build upon the material, they must distribute contributions under the same license as the original.