Machine Learning Based Prediction of Obsolescence Risk
Keywords:
Stages of the life cycle, machine learning, obsolescence, maintenance for computer equipmentAbstract
Rapid technological ups and downs have led to an increasingly quick jump in product overviews. Fast ups and downs enable useful life for long-life schemes, but they also provide significant issues for managing obsolescence when combined. Numerous techniques for anticipating obsolescence risk and product life cycle have been developed over time. However, gathering the data necessary for prediction is frequently difficult and independent, leading to disparities in forecasts. The goal of this paper is to develop a ML based system capable of accurately forecasting obsolescence risk and product life cycle while minimizing maintenance and upkeep of the predicting scheme in order to report these issues. Specifically, this innovative approach enables prediction of the obsolescence risk level as well as the timeframe during which a part becomes obsolete. A case study of the computer sale is presented to demonstrate the value and potency of the unique approach.
Downloads
References
D. Singh, “CONCEPTS OF INVENTORY AND RELATED TECHNICAL TERMINOLOGIES : A CONCEPTS OF INVENTORY AND RELATED TECHNICAL TERMINOLOGIES : A LITERATURE REVIEW,” no. August, 2022.
D. Kumar, P. K. Sarangi, and R. Verma, “A systematic review of stock market prediction using machine learning and statistical techniques,” Mater. Today Proc., vol. 49, no. January, pp. 3187–3191, 2020, doi: 10.1016/j.matpr.2020.11.399.
Colbert Edd, “THE ROLE OF DEMONSTRATION FARMS IN AGROECOLOGICAL TRANSITIONS-NEXUS FOR SCALING UP AND SCALING OUT AGROECOLOGY Edd Colbert MSc Agroecology Double Degree,” pp. 1–90, 2020.
C. Ramsey, “Forecasting risk. Risk management will become increasingly critical in accountable care models.,” Health Manag. Technol., vol. 32, no. 5, p. 22, 2011.
P. A. Sandborn, F. Mauro, and R. Knox, “A data mining based approach to electronic part obsolescence forecasting,” IEEE Trans. Components Packag. Technol., vol. 30, no. 3, pp. 397–401, 2007, doi: 10.1109/TCAPT.2007.900058.
P. Sandborn, V. Prabhakar, and O. Ahmad, “Forecasting electronic part procurement lifetimes to enable the management of DMSMS obsolescence,” Microelectron. Reliab., vol. 51, no. 2, pp. 392–399, 2011, doi: 10.1016/j.microrel.2010.08.005.
I. Trabelsi, M. Zolghadri, B. Zeddini, M. Barkallah, and M. Haddar, “Prediction of obsolescence degree as a function of time: A mathematical formulation,” Comput. Ind., vol. 129, 2021, doi: 10.1016/j.compind.2021.103470.
C. Jennings, D. Wu, and J. Terpenny, “Forecasting obsolescence risk and product life cycle with machine learning,” IEEE Trans. Components, Packag. Manuf. Technol., vol. 6, no. 9, pp. 1428–1439, 2016, doi: 10.1109/TCPMT.2016.2589206.
K. S. Moon, H. W. Lee, H. J. Kim, H. Kim, J. Kang, and W. C. Paik, “Forecasting Obsolescence of Components by Using a Clustering-Based Hybrid Machine-Learning Algorithm,” Sensors, vol. 22, no. 9, pp. 1–21, 2022, doi: 10.3390/s22093244.
Y. Grichi, T. M. Dao, and Y. Beauregard, “A new approach for optimal obsolescence forecasting based on the random forest (RF) technique and meta-heuristic particle swarm optimization (PSO),” Proc. Int. Conf. Ind. Eng. Oper. Manag., vol. 2018, no. JUL, pp. 1680–1688, 2018.
M. I. Jordan and T. M. Mitchell, “Machine learning: Trends, perspectives, and prospects,” Science (80-. )., vol. 349, no. 6245, pp. 255–260, 2015, doi: 10.1126/science.aaa8415.
O. B. Sezer, M. U. Gudelek, and A. M. Ozbayoglu, “Financial time series forecasting with deep learning: A systematic literature review: 2005–2019,” Appl. Soft Comput. J., vol. 90, pp. 2005–2019, 2020, doi: 10.1016/j.asoc.2020.106181.
K. S. Moon, H. W. Lee, and H. Kim, “Adaptive Data Selection-Based Machine Learning Algorithm for Prediction of Component Obsolescence,” Sensors, vol. 22, no. 20, pp. 1–21, 2022, doi: 10.3390/s22207982.
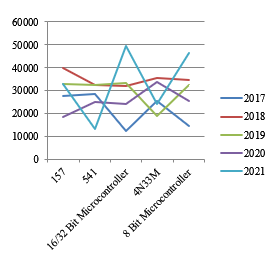
Downloads
Published
How to Cite
Issue
Section
License
Copyright (c) 2023 Ajay K. Dass , S. D. Lokhande

This work is licensed under a Creative Commons Attribution-ShareAlike 4.0 International License.
All papers should be submitted electronically. All submitted manuscripts must be original work that is not under submission at another journal or under consideration for publication in another form, such as a monograph or chapter of a book. Authors of submitted papers are obligated not to submit their paper for publication elsewhere until an editorial decision is rendered on their submission. Further, authors of accepted papers are prohibited from publishing the results in other publications that appear before the paper is published in the Journal unless they receive approval for doing so from the Editor-In-Chief.
IJISAE open access articles are licensed under a Creative Commons Attribution-ShareAlike 4.0 International License. This license lets the audience to give appropriate credit, provide a link to the license, and indicate if changes were made and if they remix, transform, or build upon the material, they must distribute contributions under the same license as the original.