Image Based Analysis for Bone Marrow Cancer Detection using Soft Computing Techniques: A Review
Keywords:
Leukemia, classification, detection, segmentation, featureAbstract
Bone marrow is a vital component of the human body, as it contains stem cells that form blood cells and immune system cells. Bone marrow cancer is due to hematologic disorders that affect the blood and can affect the bone marrow, white blood cells, platelets, and red blood cells. Every year, many people are diagnosed with bone marrow cancer. Bone marrow cancer can be detected by extracting features of a white blood cell. White blood cell classification is challenging for hematologists. Hematologists can identify malignant white blood cells by examining a peripheral blood smear under a microscope. However, the process is time-consuming, difficult, and expensive. Image processing and soft computing play a significant role in identifying early indicators of bone marrow cancer. The proposed study aims to investigate the existing cancer detection systems and their components. Further, this study majorly focuses on showing critical analysis of bone marrow cancer detection using image processing that includes enhancement, segmentation, feature extraction, classification, and accuracy which may help hematologists and oncologists to detect bone marrow cancer at early stages. Further, the paper also foresees the future aspects in the area of bone marrow cancer recognition systems. In comparison to manual detection techniques, these soft computing techniques are precise, trustworthy, and quick.
Downloads
References
National Cancer Institute. Available Online:
https://www.cancer.gov/ about-cancer/ understanding/what-is-cancer (accessed on 21 November 2022)
Patel, N., & Mishra, A. (2015). Automated leukaemia detection using microscopic images. Procedia Computer Science, 58, 635-642.
International Agency for Research on Cancer. Available Online: https://gco.iarc.fr/today/about (accessed on 21 November 2022)
World Life Expectancy. Available Online: https://www.world lifeexpectancy.com/india-lymphomas (accessed on 21 November 2022)
World Life Expectancy. Available Online: https://www.worldlifeexpectancy.com/india-leukemia (accessed on 24 November 2022)
International Agency for Research on Cancer. Available Online: https://gco.iarc.fr/today/data/ factsheets/populations/356-india-fact-sheets.pdf (accessed on 5 December 2022)
Rehman, N. Abbas, T. Saba, S. I. ur Rahman, Z. Mehmood, and H. Kolivand, “Classification of acute lymphoblastic leukemia using deep learning,” Microscopy Research and Technique, vol. 81, no. 11, pp. 1310–1317, Oct. 2018, doi: https://doi.org/10.1002/ jemt.23139.
N. Ahmed, A. Yigit, Z. Isik, and A. Alpkocak, “Identification of Leukemia Subtypes from Microscopic Images Using Convolutional Neural Network,” Diagnostics, vol. 9, no. 3, p. 104, Aug. 2019,
doi: https://doi.org/10.3390/diagnostics9030104.
S. Shafique and S. Tehsin, “Acute Lymphoblastic Leukemia Detection and Classification of Its Subtypes Using Pretrained Deep Convolutional Neural Networks,” Technology in Cancer Research & Treatment, vol. 17, p. 153303381880278, Jan. 2018,
doi: https://doi.org/10.1177/1533033818802789.
M. Claro, L. Vogado, R. Veras, A. Santana, J. Tavares, J. Santos, and V. Machado, “Convolution Neural Network Models for Acute Leukemia Diagnosis,” IEEE Xplore, Jul. 01, 2020. https://ieeexplore.ieee.org/document/9145406.
J. Rawat, A. Singh, B. HS, J. Virmani, and J. S. Devgun, “Computer assisted classification framework for prediction of acute lymphoblastic and acute myeloblastic leukemia,” Biocybernetics and Biomedical Engineering, vol. 37, no. 4, pp. 637–654, 2017, doi: https://doi.org/10.1016/ j.bbe.2017.07.003.
Y. Li, R. Zhu, L. Mi, Y. Cao, and D. Yao, “Segmentation of White Blood Cell from Acute Lymphoblastic Leukemia Images Using Dual-Threshold Method,” Computational and Mathematical Methods in Medicine, vol. 2016, pp. 1–12, 2016,
doi: https://doi.org/10.1155/2016/9514707.
S. Ravikumar, and A. Shanmugam, “WBC image segmentation and classification using RVM,” Applied Mathematical Sciences, vol. 8, no. 45, pp. 2227–2237, 2014, doi: https://doi.org/10.12988/ ams.2014.43191.
H. Kandil and O. A. Hassan, “Automatic Segmentation of Acute Leukemia Cells,” International Journal of Computer Applications, vol. 133, no. 10, pp. 1–8, Jan. 2016, Accessed: Feb. 22, 2023. [Online]. Available:
https://www.ijcaonline.org/archives/ volume133/number10/23819-2016907904.
N. Patel, and A. Mishra, “Automated Leukaemia Detection Using Microscopic Images,” Procedia Computer Science, vol. 58, pp. 635–642, 2015, doi: https://doi.org/10.1016/j.procs.2015.08.082.
M. D. Joshi, A. H. Karode, and S. R. Suralkar, “White Blood Cells Segmentation and Classification to Detect Acute Leukemia,” International Journal of Emerging Trends & Technology in Computer Science (IJETTCS), vol. 2, no. 2278–6856, pp. 147–151, May 2013.
J. Rawat, A. Singh, B. HS, J. Virmani, and J. S. Devgun, “Computer assisted classification framework for prediction of acute lymphoblastic and acute myeloblastic leukemia,” Biocybernetics and Biomedical Engineering, vol. 37, no. 4, pp. 637–654, 2017, doi: https://doi.org/10.1016/ j.bbe.2017.07.003.
H. N. Fakhouri, and S. H. Al-Sharaeh, “A Hybrid Methodology for Automation the Diagnosis of Leukemia Based on Quantitative and Morphological Feature Analysis,” Modern Applied Science, vol. 12, no. 3, p. 56, Feb. 2018, doi: https://doi.org/10.5539/mas.v12n3p56.
E. Abdulhay, M. A. Mohammed, D. A. Ibrahim, N. Arunkumar, and V. Venkatraman, “Computer Aided Solution for Automatic Segmenting and Measurements of Blood Leucocytes Using Static Microscope Images,” Journal of Medical Systems, vol. 42, no. 4, Feb. 2018,
doi: https://doi.org/10.1007/s10916-018-0912-y.
Rehman, N. Abbas, T. Saba, S. I. ur Rahman, Z. Mehmood, and H. Kolivand, “Classification of acute lymphoblastic leukemia using deep learning,” Microscopy Research and Technique, vol. 81, no. 11, pp. 1310–1317, Oct. 2018,
doi: https://doi.org/10.1002/jemt.23139.
S. Alférez, A. Merino, L. E. Mujica, M. Ruiz, L. Bigorra, and J. Rodellar, “Automatic classification of atypical lymphoid B cells using digital blood image processing,” International Journal of Laboratory Hematology, vol. 36, no. 4, pp. 472–480, Aug. 2014,
doi: https://doi.org/10.1111/ijlh.12175.
F. Kazemi, T. A. Najafabadi, and B. N. Araabi, “Automatic Recognition of Acute Myelogenous Leukemia in Blood Microscopic Images Using K-means Clustering and Support Vector Machine,” Journal of medical signals and sensors, vol. 6, no. 3, pp. 183–93, 2016, Available:
https://www.ncbi.nlm.nih.gov/pmc/articles/PMC4973462/
S. Mohapatra, D. Patra, S. Kumar, and S. Satpathy, “Lymphocyte image segmentation using functional link neural architecture for acute leukemia detection,” Biomedical Engineering Letters, vol. 2, no. 2, pp. 100–110, Jun. 2012, doi: https://doi.org/10.1007/s13534-012-0056-9.
M. Benazzouz, I. Baghli, and M. A. Chikh, “Microscopic image segmentation based on pixel classification and dimensionality reduction,” International Journal of Imaging Systems and Technology, vol. 23, no. 1, pp. 22–28, Feb. 2013, doi: https://doi.org/10.1002/ima.22032.
M. M. Amin, S. Kermani, A. Talebi, and M. G. Oghli, “Recognition of Acute Lymphoblastic Leukemia Cells in Microscopic Images Using K-Means Clustering and Support Vector Machine Classifier,” Journal of Medical Signals and Sensors, vol. 5, no. 1, pp. 49–58, 2015, Available: https://www.ncbi.nlm.nih.gov/pmc/ articles/PMC 4335145/
M. Moradi Amin, N. Samadzadehaghdam, S. Kermani, and A. Talebi, “Enhanced Recognition of Acute Lymphoblastic Leukemia Cells in Microscopic Images based on Feature Reduction using Principle Component Analysis,” Frontiers in Biomedical Technologies, vol. 2, no. 3, pp. 128–136, Sep. 2015, Accessed: Feb. 22, 2023. [Online]. Available: https://fbt.tums.ac.ir/index.php/fbt/article/view/56
Subhan, and P. Kaur, “Significant Analysis of Leukemic Cells Extraction and Detection Using KNN and Hough Transform Algorithm,” International Journal of Computer Science Trends and Technology (IJCST), vol. 3, no. 1, pp. 27–33, Jan. 2015.
L. Putzu, G. Caocci, and C. Di Ruberto, “Leucocyte classification for leukaemia detection using image processing techniques,” Artificial Intelligence in Medicine, vol. 62, no. 3, pp. 179–191, Nov. 2014, doi: https://doi.org/10.1016/j.artmed.2014.09.002.
E. U. Francis, M. Y. Mashor, R. Hassan, and A. A. Abdullah, “Screening of bone marrow slide images for Leukemia using Multilayer Perceptron (MLP),” IEEE Xplore, Sep. 01, 2011.
https://ieeexplore.ieee.org/abstract/document/6108795
Z. Moshavash, H. Danyali, and M. S. Helfroush, “An Automatic and Robust Decision Support System for Accurate Acute Leukemia Diagnosis from Blood Microscopic Images,” Journal of Digital Imaging, vol. 31, no. 5, pp. 702–717, Apr. 2018, doi: https://doi.org/10.1007/s10278-018-0074-y.
T. Pansombut, S. Wikaisuksakul, K. Khongkraphan, and A. Phon-on, “Convolutional Neural Networks for Recognition of Lymphoblast Cell Images,” Computational Intelligence and Neuroscience, vol. 2019, p. e7519603, Jun. 2019, doi: https://doi.org/ 10.1155/2019/7519603.
P. Viswanathan, “Fuzzy C Means Detection of Leukemia Based on Morphological Contour Segmentation,” Procedia Computer Science, vol. 58, pp. 84–90, 2015, doi: https://doi.org/10.1016/ j.procs.2015.08.017.
N. H. Harun, A. S. Abdul. Nasir, M. Y. Mashor, and R. Hassan, “Unsupervised Segmentation Technique for Acute Leukemia Cells Using Clustering Algorithms,” International Journal of Computer, Control, Quantum and Information Engineering, vol. 9, no. 1, pp. 253–259, Jan. 2015.
M. MoradiAmin, A. Memari, N. Samadzadehaghdam, S. Kermani, and A. Talebi, “Computer aided detection and classification of acute lymphoblastic leukemia cell subtypes based on microscopic image analysis,” Microscopy Research and Technique, vol. 79, no. 10, pp. 908–916, Jul. 2016, doi: https://doi.org/10.1002/ jemt.2 2718.
K. Lakshminarayanan, S. Ranjani, J. S. Pavithra, and A. S. Grace, “Acute Lymphoblastic Leukemia Detection and Classification Using Random Forest Classifier,” Francis Xavier Journal of Science Engineering and Management (FXJSEM), vol. 2, no. 1, pp. 10–13, Oct. 2021.
N. Chatap, and S. Shibu, “Analysis of blood samples for counting leukemia cells using Support vector machine and nearest neighbour,” OSR Journal of Computer Engineering (IOSR-JCE), vol. 16, no. 5, pp. 79–87, Sep. 2014.
L. H. S. Vogado, R. M. S. Veras, Flavio. H. D. Araujo, R. R. V. Silva, and K. R. T. Aires, “Leukemia diagnosis in blood slides using transfer learning in CNNs and SVM for classification,” Engineering Applications of Artificial Intelligence, vol. 72, pp. 415–422, Jun. 2018, doi: https://doi.org/10.1016/ j.engappai.2018.04.024.
M. Shaheen, R. Khan, R. R. Biswal, M. Ullah, A. Khan, M. I. Uddin, and A. Waheed, “Acute Myeloid Leukemia (AML) Detection Using AlexNet Model,” Complexity, vol. 2021, pp. 1–8, May 2021, doi: https://doi.org/10.1155/ 2021/6658192.
S. Shafique and S. Tehsin, “Acute Lymphoblastic Leukemia Detection and Classification of Its Subtypes Using Pretrained Deep Convolutional Neural Networks,” Technology in Cancer Research & Treatment, vol. 17, p. 153303381880278, Jan. 2018, doi: https://doi.org/10.1177/1533033818802789.
S. Mishra, B. Majhi, and P. K. Sa, “Texture feature based classification on microscopic blood smear for acute lymphoblastic leukemia detection,” Biomedical Signal Processing and Control, vol. 47, pp. 303–311, Jan. 2019,
doi: https://doi.org/10.1016/j.bspc. 2018.08.012.
M. Claro, L. Vogado, R. Veras, A. Santana, J. Tavares, J. Santos, and V. Machado, “Convolution Neural Network Models for Acute Leukemia Diagnosis,” IEEE Xplore, Jul. 01, 2020. https://ieeexplore.ieee.org/document/9145406.
M. M. Amin, S. Kermani, A. Talebi, and M. G. Oghli, “Recognition of Acute Lymphoblastic Leukemia Cells in Microscopic Images Using K-Means Clustering and Support Vector Machine Classifier,” Journal of Medical Signals and Sensors, vol. 5, no. 1, pp. 49–58, 2015, Available: https://www.ncbi.nlm.nih.gov/ pmc/articles/PMC 4335145/
H. Kandil, and O. A. Hassan, “Automatic Segmentation of Acute Leukemia Cells,” International Journal of Computer Applications, vol. 133, no. 10, pp. 1–8, Jan. 2016, Accessed: Feb. 22, 2023. [Online]. Available:
https://www.ijcaonline.org/archives/volume133/ number10/23819-2016907904
G. Singh, G. Bathla, and S. Kaur, “A review to detect leukemia cancer in medical images,” IEEE Xplore, Apr. 01, 2016.
https://ieeexplore.ieee.org/abstract/document/7813896
V. Singhal, and P. Singh, “Local Binary Pattern for automatic detection of Acute Lymphoblastic Leukemia,” IEEE Xplore, Feb. 01, 2014. https://ieeexplore.ieee.org/abstract/document/6811261.
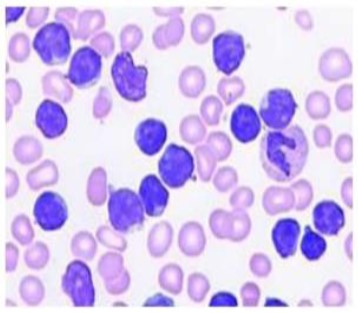
Downloads
Published
How to Cite
Issue
Section
License

This work is licensed under a Creative Commons Attribution-ShareAlike 4.0 International License.
All papers should be submitted electronically. All submitted manuscripts must be original work that is not under submission at another journal or under consideration for publication in another form, such as a monograph or chapter of a book. Authors of submitted papers are obligated not to submit their paper for publication elsewhere until an editorial decision is rendered on their submission. Further, authors of accepted papers are prohibited from publishing the results in other publications that appear before the paper is published in the Journal unless they receive approval for doing so from the Editor-In-Chief.
IJISAE open access articles are licensed under a Creative Commons Attribution-ShareAlike 4.0 International License. This license lets the audience to give appropriate credit, provide a link to the license, and indicate if changes were made and if they remix, transform, or build upon the material, they must distribute contributions under the same license as the original.