A Classification Framework for Making Decisions on Diabetes Data Trials
Keywords:
Data mining, classification, Patient Liver Dataset, clustering frameworkAbstract
One of the most prevalent metabolic conditions that raise blood sugar is diabetes. The complexity of this condition's interdependence on different circumstances makes early detection difficult. To aid medical professionals in the diagnosing process, crucial decision support systems must be developed. This study suggests creating a type 2 diabetes predictive model with high classification accuracy. The study was divided into two main categories. The proposed data mining model can be viewed as to consist of distinct phases namely classification and clustering. Two Classification phase includes the analysis of Data Mining Classification Algorithms and identifying the best algorithm for the health dataset. Classification is performed on Indian Pima Diabetes Dataset. The clustering framework is a method to identify groups within patient records and to detect clusters of attributes that can be used to identify the target class. This is the proposed data mining model that can detect clusters and classes in a given patient record. For achieving better accuracy, clusterization is performed on second dataset i.e., Indian Patient Liver Dataset. The study suggests adopting a bigger population sample without geographic restrictions as a potential solution. Also, it would be interesting to investigate tuning on top of normalised data to further improve accuracy because the created SVM model did not undergo any special hyperparameter tweaking.
Downloads
References
E. Guldogan, Z. Tunc, A. Acet, and C. Colak, “Performance evaluation of different artificial neural network models in the classification of type 2 diabetes mellitus,” The Journal of Cognitive Systems, vol. 5, no. 1, pp. 5–9, 2020. View at: Google Scholar
T. M. Alam, M. A. Iqbal, Y. Ali et al., “A model for early prediction of diabetes,” Informatics in Medicine Unlocked, vol. 16, 2019.
A. Ahmad, A. Mustapha, E. D. Zahadi, N. Masah, and N. Y. Yahaya, “Comparison between neural networks against decision tree in improving prediction accuracy for diabetes mellitus,” in Digital Information Processing and Communications, pp. 537–545, Springer, Berlin, Germany, 2011.
Z. Soltani and A. Jafarian, “A new artificial neural networks approach for diagnosing diabetes disease type II,” International Journal of Advanced Computer Science and Applications, vol. 7, no. 6, pp. 89–94, 2016.
L. AlThunayan, N. AlSahdi, and L. Syed, “Comparative analysis of different classification algorithms for prediction of diabetes disease,” in Proceedings of the Second International Conference on Internet of Things, Data and Cloud Computing, pp. 1–6, New York, NY, USA, 2017.
International Diabetes Federation, 2020, IDF SEA Members., 2020, https://idf.org/our-network/regions-members/south-east-asia/members/94-india.html.
B. M. Patil, R. C. Joshi, and D. Toshniwal, “Hybrid prediction model for Type-2 diabetic patients,” Expert Systems with Applications, vol. 37, no. 12, pp. 8102–8108, 2010.
J. C. Pickup, “Inflammation and activated innate immunity in the pathogenesis of type 2 diabetes,” Diabetes Care, vol. 27, no. 3, pp. 813–823, 2004.
D. W. Guthrie and R. A. Guthrie, “Nursing management of diabetes mellitus: a guide to the pattern approach,” Home Healthcare Nurse, vol. 23, no. 2, p. 119, 2005.
E. I. Mohamed, R. Linder, G. Perriello, N. Di Daniele, S. J. Pöppl, and A. De Lorenzo, “Predicting Type 2 diabetes using an electronic nose-based artificial neural network analysis,” Diabetes, Nutrition & Metabolism, vol. 15, no. 4, p. 215, 2002.
Mayo Clinic Staff, 2020, Prediabetes—symptoms and causes, 2020, https://www.mayoclinic.org/diseases-conditions/prediabetes/symptoms-causes/syc-20355278.
N. C. Sharma, 2019, Government survey found 11.8% prevalence of diabetes in India, 2019, https://www.livemint.com/science/health/government-survey-found-11-8-prevalence-of-diabetes-in-india-11570702665713.html.
S. Wild, G. Roglic, A. Green, R. Sicree, and H. King, “Global prevalence of diabetes- estimates for the year 2000 and projections for 2030,” Diabetes Care, vol. 27, no. 5, 2004.
World Health Organization, Global Report on Diabetes, WHO, Geneva, Switzerland, 2016, https://www.who.int/publications/i/item/9789241565257.
A. A. AlJarullah, “Decision tree discovery for the diagnosis of type II diabetes,” in Proceedings of the 2011 International Conference on Innovations in Information Technology, pp. 303–307, Abu Dhabi, UAE, April 2011.
U.S. Department of Health and Human Services, Diabetes: A National Plan for Action. The Importance of Early Diabetes Detection, U.S. Department of Health and Human Services, Washington, D.C, USA, 2004, U.S. Department of Health and Human Services, Washington, D.C, USA, 2004, https://aspe.hhs.gov/report/diabetes-national-plan-action/importance-early-diabetes-detection.
M. Komi, J. Li, Y. Zhai, and Z. Xianguo, “Application of data mining methods in diabetes prediction,” in Proceedings of the International Conference on Image, Vision and Computing Application, pp. 1006–1010, IEEE, Chengdu, China, June 2017.
Q. Wang, W. Cao, J. Guo, J. Ren, Y. Cheng, and D. N. Davis, “DMP_MI: an effective diabetes mellitus classification algorithm on imbalanced data with missing values,” IEEE Access, vol. 7, pp. 102232–102238, 2019.
M. A. Sarwar, N. Kamal, W. Hamid, and M. A. Shah, “Prediction of diabetes using machine learning algorithms in healthcare,” in Proceedings of the 24th International Conference on Automation and Computing, pp. 1–6, IEEE, Newcastle Upon Tyne, UK, September 2018.
F. G. Woldemichael and S. Menaria, “Prediction of diabetes using data mining techniques,” in Proceedings of the International Conference on Trends in Electronics and Informatics (ICOEI), pp. 414–418, IEEE, Tirunelveli, India, May 2018.
D. Sisodia and D. S. Sisodia, “Prediction of diabetes using classification algorithms,” Procedia Computer Science, vol. 132, pp. 1578–1585, 2018.
H. Wu, S. Yang, Z. Huang, J. He, and X. Wang, “Type 2 diabetes mellitus prediction model based on data mining,” Informatics in Medicine Unlocked, vol. 10, pp. 100–107, 2018.
R. Vaishali, R. Sasikala, S. Ramasubbareddy, S. Remya, and S. Nalluri, “Genetic algorithm-based feature selection and MOE Fuzzy classification algorithm on Pima Indians Diabetes dataset,” in Proceedings of the International Conference on Computing Networking and Informatics (ICCNI), pp. 1–5, IEEE, Lagos, Nigeria, October 2017.
O. Geman, I. Chiuchisan, and R. Toderean, “Application of adaptive neuro-fuzzy inference system for diabetes classification and prediction,” in Proceedings of the 2017 E-Health and Bioengineering Conference, EHB, pp. 639–642, IEEE, Sinaia, Romania, June 2017.
S. Ramesh, R. D. Caytiles, and N. C. S. Iyenga, “A deep learning approach to identify diabetes,” Advanced Science and Technology Letters, vol. 145, pp. 44–49, 2017.
Marcano-Cedeño, J. Torres, and D. Andina, “A prediction model to diabetes using Artificial Metaplasticity,” in New Challenges on Bioinspired Applications, pp. 418–425, Springer, Berlin, Germany, 2011.
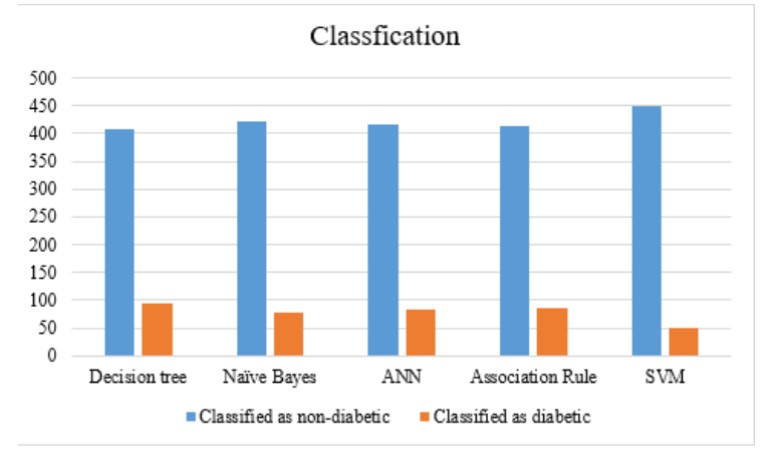
Downloads
Published
How to Cite
Issue
Section
License

This work is licensed under a Creative Commons Attribution-ShareAlike 4.0 International License.
All papers should be submitted electronically. All submitted manuscripts must be original work that is not under submission at another journal or under consideration for publication in another form, such as a monograph or chapter of a book. Authors of submitted papers are obligated not to submit their paper for publication elsewhere until an editorial decision is rendered on their submission. Further, authors of accepted papers are prohibited from publishing the results in other publications that appear before the paper is published in the Journal unless they receive approval for doing so from the Editor-In-Chief.
IJISAE open access articles are licensed under a Creative Commons Attribution-ShareAlike 4.0 International License. This license lets the audience to give appropriate credit, provide a link to the license, and indicate if changes were made and if they remix, transform, or build upon the material, they must distribute contributions under the same license as the original.