A Novel Intrusion Detection Techniques of the Computer Networks Using Machine Learning
Keywords:
Intrusion Detection Techniques, Machine -Learning, Computer Networks, Classification ApproachAbstract
Network intrusion is an unauthorized work of a computer network. Protecting the computer network against unauthorized users, even internal ones, is the goal of the network access programme. We will create a local network detection, which is a prophecy mold to discriminate amid "good quality" or typical associations and "appalling" associations, which sometimes referenced to as intruders or attacks. Evaluation of the findings for accessibility was the goal. In the Knowledge discovery Cups 1999 dataset for predicting, we also concentrated on machine learning-based classification to facilitate acquire greatest training and testing, to access our strategy for using currently available technologies. To generate various classification models, used varieties machine-learning based techniques and comparing each other for detecting best fit model for the computer networks with respect to time and accuracy.
Downloads
References
S. M. Othman, F. M. Ba-Alwi, N. T. Alsohybe, and A. Y. Al-Hashida, “Intrusion detection model using machine learning algorithm on Big Data environment,” J. Big Data, vol. 5, no. 1, 2018, doi: 10.1186/s40537-018-0145-4.
C. Aishwarya*, N. Venkateswaran, T. Supriya, and V. Sreeja, “Intrusion Detection System using KDD Cup 99 Dataset,” Int. J. Innov. Technol. Explor. Eng., vol. 4, no. 9, pp. 3169–3171, 2020, doi: 10.35940/ijitee.d2017.029420.
“IDS Security 2022,” 2022. https://www.comodo.com/ids-in-security.php
V. Degeler, R. French, and K. Jones, “Self-Healing Intrusion Detection System Concept,” in Proceedings - 2nd IEEE International Conference on Big Data Security on Cloud, IEEE BigDataSecurity 2016, 2nd IEEE International Conference on High Performance and Smart Computing, IEEE HPSC 2016 and IEEE International Conference on Intelligent Data and S, 2016, no. February 2021, pp. 351–356. doi: 10.1109/BigDataSecurity-HPSC-IDS.2016.27.
T. Wisanwanichthan and M. Thammawichai, “A Double-Layered Hybrid Approach for Network Intrusion Detection System Using Combined Naive Bayes and SVM,” IEEE Access, vol. 9, pp. 138432–138450, 2021, doi: 10.1109/ACCESS.2021.3118573.
N. Mishra and S. Mishra, “A Review on Big Data Classification : using Machine Learning Technique to Classify Intrusion,” Int. J. Res. Anal. Rev., vol. 7, no. 1, pp. 162–165, 2020, [Online]. Available: http://ijrar.org/viewfull.php?&p_id=IJRAR1BAP032
A. Mohammad, A. Maen, K. Szilveszter, and A. Mouhammd, “Evaluation of Machine Learning Algorithms for Intrusion Detection System in WSN,” in International Symposium on Intelligent Systems and Informatics, 2017, vol. 15, pp. 277–282. doi: 10.1109/SISY.2017.8080566.
H. Shapoorifard and P. Shamsinejad, “Intrusion Detection using a Novel Hybrid Method Incorporating an Improved KNN,” Int. J. Comput. Appl., vol. 173, no. 1, pp. 5–9, 2017, doi: 10.5120/ijca2017914340.
W. Wang, M. Zhu, X. Zeng, X. Ye, and Y. Sheng, “Malware traffic classification using convolutional neural network for representation learning,” in International Conference on Information Networking, 2017, no. January, pp. 712–717. doi: 10.1109/ICOIN.2017.7899588.
G. Zhao, C. Zhang, and L. Zheng, “Intrusion detection using deep belief network and probabilistic neural network,” in Proceedings - 2017 IEEE International Conference on Computational Science and Engineering and IEEE/IFIP International Conference on Embedded and Ubiquitous Computing, CSE and EUC 2017, 2017, vol. 1, no. May, pp. 639–642. doi: 10.1109/CSE-EUC.2017.119.
B. A. Tama, M. Comuzzi, and K. H. Rhee, “TSE-IDS: A Two-Stage Classifier Ensemble for Intelligent Anomaly-Based Intrusion Detection System,” IEEE Access, vol. 7, pp. 94497–94507, 2019, doi: 10.1109/ACCESS.2019.2928048.
R. Jiadong, L. Xinqian, W. Qian, H. Haitao, and Z. Xiaolin, “An Multi-Level Intrusion Detection Method Based on KNN Outlier Detection and Random Forests,” J. Comput. Res. Dev., vol. 56, no. 3, pp. 566–575, 2019, doi: 10.7544/issn1000-1239.2019.20180063.
P. Tao, Z. Sun, and Z. Sun, “An Improved Intrusion Detection Algorithm Based on GA and SVM,” IEEE Access, vol. 6, pp. 13624–13631, 2018, doi: 10.1109/ACCESS.2018.2810198.
Y. Ding and Y. Zhai, “Intrusion detection system for NSL-KDD dataset using convolutional neural networks,” ACM Int. Conf. Proceeding Ser., pp. 81–85, 2018, doi: 10.1145/3297156.3297230.
T. Su, H. Sun, J. Zhu, S. Wang, and Y. Li, “BAT: Deep Learning Methods on Network Intrusion Detection Using NSL-KDD Dataset,” IEEE Access, vol. 8, pp. 29575–29585, 2020, doi: 10.1109/ACCESS.2020.2972627.
Y. Fu, Y. Du, Z. Cao, Q. Li, and W. Xiang, “A Deep Learning Model for Network Intrusion Detection with Imbalanced Data,” Electron., vol. 11, no. 898, pp. 1–13, 2022, doi: 10.3390/electronics11060898.
K. Vengatesan, A. Kumar, R. Naik, and D. K. Verma, “Anomaly based novel intrusion detection system for network traffic reduction,” in Proceedings of the International Conference on I-SMAC (IoT in Social, Mobile, Analytics and Cloud), I-SMAC 2018, 2018, no. August, pp. 688–690. doi: 10.1109/I-SMAC.2018.8653735.
Z. Ahmad, A. Shahid Khan, C. Wai Shiang, J. Abdullah, and F. Ahmad, “Network intrusion detection system: A systematic study of machine learning and deep learning approaches,” Trans. Emerg. Telecommun. Technol., vol. 32, no. 1, pp. 1–29, 2021, doi: 10.1002/ett.4150.
S. Sah, “Machine Learning: A Review of Learning Types,” ResearchGate, no. July, 2020, doi: 10.20944/preprints202007.0230.v1.
E. Burns, “machine learning,” Machine Learning, 2021.
“machine-learning-algorithms.” https://www.javatpoint.com/machine-learning-algorithms
A. Abedinia, “Survey of the Decision Trees Algorithms (CART, C4.5, ID3) | by Aydin Abedinia | Medium,” 2019.
“machine-learning-decision-tree-classification-algorithm.”https://www.javatpoint.com/machine-learning-decision-tree-classification-algorithm
“Random Forest Algorithm,” 2018. https://www.javatpoint.com/machine-learning-random-forest-algorithm
“logistic-regression-in-machine-learning.” https://www.javatpoint.com/logistic-regression-in-machine-learning
“Gradient Descent in Machine Learning.” https://www.javatpoint.com/gradient-descent-in-machine-learning
N. Mishra, S. Mishra, and B. Patnaik, “A Novel Intrusion Detection System Based on Random Oversampling and Deep Neural Network,” Indian J. Comput. Sci. Eng., vol. 13, no. 6, pp. 1924–1936, 2022, doi: 10.21817/indjcse/2022/ v13i6/221306136.
S. J. Stolfo, W. Fan, W. Lee, A. Prodromidis, and P. K. Chan, “KDD-CUP-99 Task Description,” 1999.
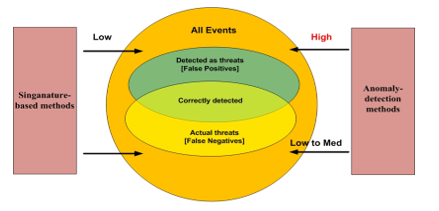
Downloads
Published
How to Cite
Issue
Section
License

This work is licensed under a Creative Commons Attribution-ShareAlike 4.0 International License.
All papers should be submitted electronically. All submitted manuscripts must be original work that is not under submission at another journal or under consideration for publication in another form, such as a monograph or chapter of a book. Authors of submitted papers are obligated not to submit their paper for publication elsewhere until an editorial decision is rendered on their submission. Further, authors of accepted papers are prohibited from publishing the results in other publications that appear before the paper is published in the Journal unless they receive approval for doing so from the Editor-In-Chief.
IJISAE open access articles are licensed under a Creative Commons Attribution-ShareAlike 4.0 International License. This license lets the audience to give appropriate credit, provide a link to the license, and indicate if changes were made and if they remix, transform, or build upon the material, they must distribute contributions under the same license as the original.