Real-Time Hand Gesture Recognition for Improved Communication with Deaf and Hard of Hearing Individuals
Keywords:
Convolutional neural network, Deep learning, Gesture recognition, Sign language recognition, Hearing disabilityAbstract
People who lack knowledge in sign language often face difficulty communicating effectively with those who are deaf or hard of hearing, but in such cases hand gesture recognition technology can provide an easy-to-use alternative for computer communication. This research concentrates on developing a hand gesture recognition system that works in real-time and utilizes universal physical traits present in all human hands as its basis for identifying these movements by automating the identification of sign gestures obtained through webcam footage using Convolutional Neural Networks (CNN) alongside additional algorithms integrated into this framework. Natural hand gestures are used for communication while the system prioritizes segmentation of these movements, and the automated recognition feature of the system is highly beneficial for people with hearing disabilities as it can help eliminate long-lasting communication barriers. The system also has potential applications in areas like human-machine interfaces and immersive gaming technology, so all parties involved can benefit from the ease that real-time hand gesture recognition brings through its potential as a tool for improving communication and reducing barriers faced by those who are deaf or hard of hearing.
Downloads
References
J. Padgett, R. (2014). The Contribution of American Sign Language to Sign-Print Bilingualism in Children. Journal of Communication Disorders, Deaf Studies & Hearing Aids, 02(02). https://doi.org/10.4172/2375-4427.1000108
Evaluation of Measures to Facilitate Access to Care for Pregnant Deaf Patients: Use of Interpreters and Training of Caregivers in Sign Language. (2013). Journal of Communication Disorders, Deaf Studies & Hearing Aids, 01(01). https://doi.org/10.4172/2375-4427.1000103
Novogrodsky, R., Fish, S., & Hoffmeister, R. (2014). The Acquisition of Synonyms in American Sign Language (ASL): Toward a Further Understanding of the Components of ASL Vocabulary Knowledge. Sign Language Studies, 14(2), 225–249. https://doi.org/10.1353/sls.2014.0003
Ahmed, T. (2012). A Neural Network based Real Time Hand Gesture Recognition System. International Journal of Computer Applications, 59(4), 17–22. https://doi.org/10.5120/9535-3971
Amer Kadhim, R., & Khamees, M. (2020). A Real-Time American Sign Language Recognition System using Convolutional Neural Network for Real Datasets. TEM Journal, 937–943. https://doi.org/10.18421/tem93-14
Sign Language Translation Using Deep Convolutional Neural Networks. (2020). KSII Transactions on Internet and Information Systems, 14(2). https://doi.org/10.3837/tiis.2020.02.009
Kumbhar, A., Sathe, V., Pathak, A., & Kodmelwar, M. K. (2015). Hand tracking in HCI framework intended for wireless interface. International Journal of Computer Engineering in Research Trends , 2(12), 821-824.
Damatraseta, F., Novariany, R., & Ridhani, M. A. (2021). Real-time BISINDO Hand Gesture Detection and Recognition with Deep Learning CNN. Jurnal Informatika Kesatuan, 1(1), 71–80. https://doi.org/10.37641/jikes.v1i1.774
K, S., & R, P. (2022). Sign Language Recognition System Using Neural Networks. International Journal for Research in Applied Science and Engineering Technology, 10(6), 827–831. https://doi.org/10.22214/ijraset.2022.43787
M. M. Venkata Chalapathi, M. Rudra Kumar, Neeraj Sharma, S. Shitharth, "Ensemble Learning by High-Dimensional Acoustic Features for Emotion Recognition from Speech Audio Signal", Security and Communication Networks, vol. 2022, Article ID 8777026, 10 pages, 2022. https://doi.org/10.1155/2022/8777026
Boinpally, A., Ventrapragada, S. B., Prodduturi, S. R., Depa, J. R., & Sharma, K. V. (2023). Vision-based hand gesture recognition for Indian sign language using convolution neural network. International Journal of Computer Engineering in Research Trends, 10(1), 1-9. https://doi.org/10.47577/IJCERT/2023/V10I0101
Rudra Kumar, M., Rashmi Pathak, and Vinit Kumar Gunjan. "Diagnosis and Medicine Prediction for COVID-19 Using Machine Learning Approach." Computational Intelligence in Machine Learning: Select Proceedings of ICCIML 2021. Singapore: Springer Nature Singapore, 2022. 123-133.
Alsaadi, Z., Alshamani, E., Alrehaili, M., Alrashdi, A. A. D., Albelwi, S., & Elfaki, A. O. (2022). A Real Time Arabic Sign Language Alphabets (ArSLA) Recognition Model Using Deep Learning Architecture. Computers, 11(5), 78. https://doi.org/10.3390/computers11050078
Rudra Kumar, M., Rashmi Pathak, and Vinit Kumar Gunjan. "Machine Learning-Based Project Resource Allocation Fitment Analysis System (ML-PRAFS)." Computational Intelligence in Machine Learning: Select Proceedings of ICCIML 2021. Singapore: Springer Nature Singapore, 2022. 1-14.
Real time sign language detection system using deep learning techniques. (2022). Journal of Pharmaceutical Negative Results, 13(S01). https://doi.org/10.47750/pnr.2022.13.s01.126
Amer Kadhim, R., & Khamees, M. (2020). A Real-Time American Sign Language Recognition System using Convolutional Neural Network for Real Datasets. TEM Journal, 937–943. https://doi.org/10.18421/tem93-14
Kumar, P. ., Gupta, M. K. ., Rao, C. R. S. ., Bhavsingh, M. ., & Srilakshmi, M. (2023). A Comparative Analysis of Collaborative Filtering Similarity Measurements for Recommendation Systems. International Journal on Recent and Innovation Trends in Computing and Communication, 11(3s), 184–192.
Kumar, P. ., Gupta, M. K. ., Rao, C. R. S. ., Bhavsingh, M. ., & Srilakshmi, M. (2023). A Comparative Analysis of Collaborative Filtering Similarity Measurements for Recommendation Systems. International Journal on Recent and Innovation Trends in Computing and Communication, 11(3s), 184–192.
B, G. D., K, S. R., & P, V. K. (2023). SMERAS - State Management with Efficient Resource Allocation and Scheduling in Big Data Stream Processing Systems. International Journal of Computer Engineering in Research Trends, 10(4), 150–154. https://doi.org/10.22362/ijcertpublications.v10i4.5
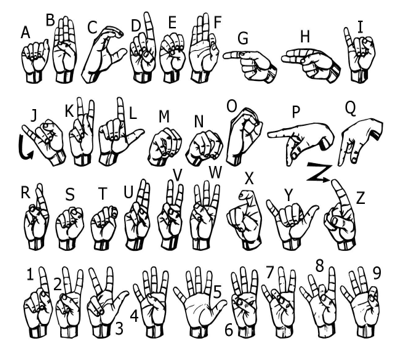
Downloads
Published
How to Cite
Issue
Section
License

This work is licensed under a Creative Commons Attribution-ShareAlike 4.0 International License.
All papers should be submitted electronically. All submitted manuscripts must be original work that is not under submission at another journal or under consideration for publication in another form, such as a monograph or chapter of a book. Authors of submitted papers are obligated not to submit their paper for publication elsewhere until an editorial decision is rendered on their submission. Further, authors of accepted papers are prohibited from publishing the results in other publications that appear before the paper is published in the Journal unless they receive approval for doing so from the Editor-In-Chief.
IJISAE open access articles are licensed under a Creative Commons Attribution-ShareAlike 4.0 International License. This license lets the audience to give appropriate credit, provide a link to the license, and indicate if changes were made and if they remix, transform, or build upon the material, they must distribute contributions under the same license as the original.