Enhancing Ultrasound Image Quality Using Machine Learning Techniques
Keywords:
Machine learning, deep learning, ultrasound imaging, medical diagnostics, NDEAbstract
Machine learning (ML) techniques are becoming more commonplace as a result of their utility in addressing complex issues in several contexts. Using ML methods in ultrasonic imaging applications is nothing new, but there has been a meteoric rise in research into this area over the last several years. Medical diagnostics and non-destructive assessment use ultrasonic imaging extensively, and both have benefited from the use of machine learning methods. In the former, which constitutes the bulk of the review, solutions that pertain to the detection/classification of material defects or specific patterns are reported, while in the latter, studies were categorised according to the body organ examined and the methodology adopted. Finally, the study's analysis is summarised, and the key benefits of machine learning are explored..
Downloads
References
S. Wen, B. Peng, X. Wei, J. Luo and J. Jiang, "Convolutional Neural Network-Based Speckle Tracking for Ultrasound Strain Elastography: An Unsupervised Learning Approach," in IEEE Transactions on Ultrasonics, Ferroelectrics, and Frequency Control, vol. 70, no. 5, pp. 354-367, May 2023, doi: 10.1109/TUFFC.2023.3243539.
Q. Chen, X. Min, H. Duan, Y. Zhu and G. Zhai, "Muiqa: Image Quality Assessment Database And Algorithm For Medical Ultrasound Images," 2021 IEEE International Conference on Image Processing (ICIP), Anchorage, AK, USA, 2021, pp. 2958-2962, doi: 10.1109/ICIP42928.2021.9506431.
Z. Zhou, Y. Wang, Y. Guo, Y. Qi and J. Yu, "Image Quality Improvement of Hand-Held Ultrasound Devices With a Two-Stage Generative Adversarial Network," in IEEE Transactions on Biomedical Engineering, vol. 67, no. 1, pp. 298-311, Jan. 2020, doi: 10.1109/TBME.2019.2912986.
Xiongkuo Min, Jiantao Zhou, Guangtao Zhai, Patrick Le Callet, Xiaokang Yang and Xinping Guan, "A metric for light field reconstruction compression and display quality evaluation", IEEE Transactions on Image Processing, vol. 29, pp. 3790-3804, 2020.
Huiyu Duan, Guangtao Zhai, Xiongkuo Min, Yucheng Zhu, Yi Fang and Xiaokang Yang, "Perceptual quality assessment of omnidirectional images", 2018 IEEE international symposium on circuits and systems (ISCAS), pp. 1-5, 2018
Xiongkuo Min, Guangtao Zhai, Ke Gu, Yutao Liu and Xiaokang Yang, "Blind image quality estimation via distortion aggravation", IEEE Transactions on Broadcasting, vol. 64, no. 2, pp. 508-517, 2018
Jie Hu, Li Shen and Gang Sun, "Squeeze-and-excitation networks", Proceedings of the IEEE conference on computer vision and pattern recognition, pp. 7132-7141, 2018.
Huiyu Duan, Guangtao Zhai, Xiaokang Yang, Duo Li and Wenhan Zhu, "Ivqad 2017: An immersive video quality assessment database", 2017 International Conference on Systems Signals and Image Processing (IWSSIP), pp. 1-5, 2017.
Wufeng Xue, Ali Islam, Mousumi Bhaduri and Shuo Li, "Direct multitype cardiac indices estimation via joint representation and regression learning", IEEE transactions on medical imaging, vol. 36, no. 10, pp. 2057-2067, 2017.
Lingyun Wu, Jie-Zhi Cheng, Shengli Li, Baiying Lei, Tianfu Wang and Dong Ni, "Fuiqa: Fetal ultrasound image quality assessment with deep convolutional networks", IEEE transactions on cybernetics, vol. 47, no. 5, pp. 1336-1349, 2017.
Xiongkuo Min, Ke Gu, Guangtao Zhai, Jing Liu, Xiaokang Yang and Chang Wen Chen, "Blind quality assessment based on pseudo-reference image", IEEE Transactions on Multimedia, vol. 20, no. 8, pp. 2049-2062, 2017
Forrest N Iandola, Song Han, Matthew W Moskewicz, Khalid Ashraf, William J Dally and Kurt Keutzer, "Squeezenet: Alexnet-level accuracy with 50x fewer parameters and!‘ 0.5 mb model size", arXiv preprint arXiv:1602.07360, 2016
Patil, R. A. ., & Patil, P. D. . (2023). Skewed Evolving Data Streams Classification with Actionable Knowledge Extraction using Data Approximation and Adaptive Classification Framework. International Journal on Recent and Innovation Trends in Computing and Communication, 11(1), 38–52. https://doi.org/10.17762/ijritcc.v11i1.5985
Heshalini Rajagopal, Li Sze Chow and Raveendran Paramesran, "Subjective versus objective assessment for magnetic resonance images", Proc. 17th Int. Conf. Commun. Inf. Technol. Eng, vol. 9, pp. 2426-2431, 2015.
Mr. Nikhil Surkar, Ms. Shriya Timande. (2012). Analysis of Analog to Digital Converter for Biomedical Applications. International Journal of New Practices in Management and Engineering, 1(03), 01 - 07. Retrieved from http://ijnpme.org/index.php/IJNPME/article/view/6
Michele A Saad, Alan C Bovik and Christophe Charrier, "Blind image quality assessment: A natural scene statistics approach in the dct domain", IEEE transactions on Image Processing, vol. 21, no. 8, pp. 3339-3352, 2012.
Peng Ye, Jayant Kumar, Le Kang and David Doermann, "Unsupervised feature learning framework for no-reference image quality assessment", 2012 IEEE conference on computer vision and pattern recognition, pp. 1098-1105, 2012.
D. Selvathi and V. S. Sharnitha, "Thyroid classification and segmentation in ultrasound images using machine learning algorithms," 2011 International Conference on Signal Processing, Communication, Computing and Networking Technologies, Thuckalay, India, 2011, pp. 836-841, doi: 10.1109/ICSCCN.2011.6024666.
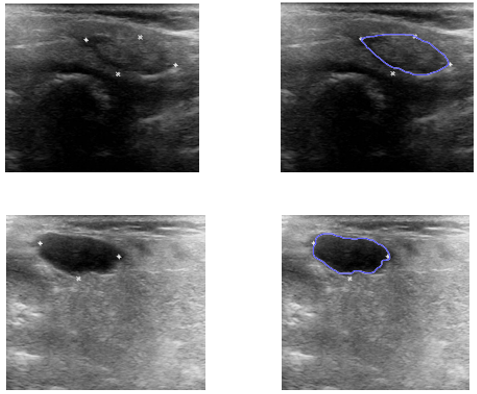
Downloads
Published
How to Cite
Issue
Section
License

This work is licensed under a Creative Commons Attribution-ShareAlike 4.0 International License.
All papers should be submitted electronically. All submitted manuscripts must be original work that is not under submission at another journal or under consideration for publication in another form, such as a monograph or chapter of a book. Authors of submitted papers are obligated not to submit their paper for publication elsewhere until an editorial decision is rendered on their submission. Further, authors of accepted papers are prohibited from publishing the results in other publications that appear before the paper is published in the Journal unless they receive approval for doing so from the Editor-In-Chief.
IJISAE open access articles are licensed under a Creative Commons Attribution-ShareAlike 4.0 International License. This license lets the audience to give appropriate credit, provide a link to the license, and indicate if changes were made and if they remix, transform, or build upon the material, they must distribute contributions under the same license as the original.