U-In-Effnet: Semantic Segmentation With The Effect Of Magnifying Glasss
Keywords:
Unet, EfficientNet, Semantic Segmentation, CNNAbstract
Each of the growing advancement in the field of Semantic segmentation has taken us a step ahead towards effectively achieving Artificial Intelligence. CNN has again and again proved its semantic competency in extracting rich features at various level of abstraction. Semantic segmentation of the image for better understanding of the objects and its context is essential for wide range of applications ranging from scene classification to automatic driving vehicles. An encoder- decoder inspired U-net architecture using Efficient Net as backbone is been proposed. To replicate the effect of magnifying glass for diverse feature rich extraction inception blocks with different kernel size is added into decoder. The proposed method is tested on the most unstructured, delineated Indian Driving Dataset (IDD) and the popular benchmark Pascal VOC 2012 dataset. The architecture with its well defined segmentation map outperforms the previous benchmark results by attaining mean Intersection over Union (mIoU) of 0.78 and 0.63 on Pascal VOC and IDDLite dataset respectively.
Downloads
References
T. Deng, "A Survey of Convolutional Neural Networks for Image Classification: Models and Datasets,” 2022 International Conference on Big Data, Information and Computer Network (BDICN), 2022, pp. 746-749, doi: 10.1109/BDICN55575.2022.00145.
Yujian Mo, Yan Wu, Xinneng Yang, Feilin Liu, Yujun Liao. “Review the state-of-the-art technologies of semantic segmentation based on deep learning”, Neurocomputing, Vol. 493, 2022, pp 626-646, ISSN 0925-2312, doi: https://doi.org/10.1016/j.neucom.2022.01.005.
E. Shelhamer, J. Long, and T. Darrell. Fully convolutional networks for semantic segmentation. IEEE Transactions on Pattern Analysis and Machine Intelligence, 39(4):640–651, April 2017.
Olaf Ronneberger, Philipp Fischer, and Thomas Brox. Unet: Convolutional networks for biomedical image segmentation. In Medical Image Computing and Computer-Assisted Intervention – MICCAI 2015, pages 234–241, Cham, 2015. Springer International Publishing.
H. Zhao, J. Shi, X. Qi, X. Wang and J. Jia, "Pyramid Scene Parsing Network," 2017 IEEE Conference on Computer Vision and Pattern Recognition (CVPR), 2017, pp. 6230-6239, doi: 10.1109/CVPR.2017.660.
Liang-Chieh Chen, Yukun Zhu, George Papandreou, Florian Schroff, and Hartwig Adam. Encoder-decoder with atrous separable convolution for semantic image segmentation. 2018 Europian Conference on Computer Vision (ECCV), 2018.
K. He, G. Gkioxari, P. Dollár and R. Girshick, "Mask R-CNN," 2017 IEEE International Conference on Computer Vision (ICCV), 2017, pp. 2980-2988, doi: 10.1109/ICCV.2017.322.
Parekh D, Poddar N, Rajpurkar A, Chahal M, Kumar N, Joshi GP, Cho W. A Review on Autonomous Vehicles: Progress, Methods and Challenges. Electronics. 2022; 11(14):2162. https://doi.org/10.3390/electronics11142162.
Kugelman, J., Allman, J., Read, S.A. et al. A comparison of deep learning U-Net architectures for posterior segment OCT retinal layer segmentation. Sci Rep 12, 14888. 2022. doi: https://doi.org/10.1038/s41598-022-18646-2.
Wang, Jinke et al. Automatic Liver Segmentation Using EfficientNet and Attention-Based Residual U-Net in CT. Journal of Digital Imaging. 2022. 35. 10.1007/s10278-022-00668-x.
Mohan, R., Valada, A. EfficientPS: Efficient Panoptic Segmentation. Int J Comput Vis 129, 1551–1579 (2021). https://doi.org/10.1007/s11263-021-01445-z.
B. Baheti, S. Innani, S. Gajre and S. Talbar, "Eff-UNet: A Novel Architecture for Semantic Segmentation in Unstructured Environment," 2020 IEEE/CVF Conference on Computer Vision and Pattern Recognition Workshops (CVPRW), 2020, pp. 1473-1481, doi: 10.1109/CVPRW50498.2020.00187.
Lu Haoran, She Yifei, Tie Jun, Xu Shengzhou. "Half-UNet: A Simplified U-Net Architecture for Medical Image Segmentation". Frontiers in Neuroinformatics, Vol. 16, 2022, doi: 10.3389/fninf.2022.911679.
Wu, J., Zhou, S., Zuo, S. et al. U-Net combined with multi-scale attention mechanism for liver segmentation in CT images. BMC Med Inform Decis Mak. 21, 283 (2021). https://doi.org/10.1186/s12911-021-01649-w.
Ayalew, Y.A., Fante, K.A. Mohammed, M. “Modified U-Net for liver cancer segmentation from computed tomography images with a new class balancing method”. BMC biomed eng 3, 4 (2021). https://doi.org/10.1186/s42490-021-00050-y.
Xiaomeng Feng, Taiping Wang, Xiaohang Yang, Minfei Zhang, Wanpeng Guo, Weina Wang. ConvWin-UNet: UNet-like hierarchical vision Transformer combined with convolution for medical image segmentation[J]. Mathematical Biosciences and Engineering, 2023, 20(1): 128-144. doi: 10.3934/mbe.2023007.
Mingxing Tan, Quoc V. Le. EfficientNet: Rethinking Model Scaling for Convolutional Neural Networks. Proceedings of the 36th International Conference on Machine Learning (ICML), 2019. http://arxiv.org/abs/1905.11946.
Sandler, Mark, Howard, Andrew, Zhu, Menglong, Zhmoginov, Andrey, Chen, Liang-Chieh. (2018). MobileNetV2: Inverted Residuals and Linear Bottlenecks. 4510-4520. 10.1109/CVPR.2018.00474.
Christian Szegedy, Sergey Ioffe, Vincent Vanhoucke, Alexander A. Alemi. Inception-v4, inception-resnet and the impact of residual connections on learning. In Proceedings of the Thirty-First AAAI Conference on Artificial Intelligence, AAAI’17, page 4278–4284. AAAI Press, 2017.
M. Everingham, L. Van Gool, C. K. I. Williams, J. Winn, A. Zisserman. The PASCAL Visual Object Classes Challenge 2011 (VOC 2011). Results. http://www.pascalnetwork. org/challenges/VOC/voc2011/workshop/index.html.
The World Health Organization. “Global Status Report on Road Safety”. 2018. Accessed on: Jan. 15, 2020.
Varma, Girish, Subramanian, C.V.et al. “IDD: A Dataset for Exploring Problems of Autonomous Navigation in Unconstrained Environments”. 1743-1751. 2019. 10.1109/WACV.2019.00190.
S. Gupta, P. Arbelaez, and J. Malik. Perceptual organization and recognition of indoor scenes from RGB-D images. In CVPR, 2013.
B. Hariharan, P. Arbel´aez, R. Girshick, and J. Malik. Simultaneous detection and segmentation. In European Conference on Computer Vision (ECCV), 2014.
S. Mehta, H. Hajishirzi and M. Rastegari, "DiCENet: Dimension-Wise Convolutions for Efficient Networks" in IEEE Transactions on Pattern Analysis & Machine Intelligence, vol. 44, no. 05, pp. 2416-2425, 2022. doi: 10.1109/TPAMI.2020.3041871.
Mehta, Sachin & Rastegari, Mohammad & Shapiro, Linda & Hajishirzi, Hannaneh. (2019). ESPNetv2: A Light-Weight, Power Efficient, and General Purpose Convolutional Neural Network. 9182-9192. 10.1109/CVPR.2019.00941.
Liu, Wei, Rabinovich, Andrew & Berg, Alexander. (2015). ParseNet: Looking Wider to See Better.CoRR. http://arxiv.org/abs/1506.04579.
L. -C. Chen, G. Papandreou, I. Kokkinos, K. Murphy and A. L. Yuille, "DeepLab: Semantic Image Segmentation with Deep Convolutional Nets, Atrous Convolution, and Fully Connected CRFs," in IEEE Transactions on Pattern Analysis and Machine Intelligence, vol. 40, no. 4, pp. 834-848, 1 April 2018, doi: 10.1109/TPAMI.2017.2699184.
Fisher Yu, Vladlen Koltun, and Thomas A. Funkhouser. Dilated residual networks. 2017 IEEE Conference on Computer Vision and Pattern Recognition (CVPR), pages 636– 644, 2017.
Eduardo Romera, Jose M. Alvarez, Luis Miguel Bergasa, and Roberto Arroyo. Erfnet: Efficient residual factorized convnet for real-time semantic segmentation. IEEE Transactions on Intelligent Transportation Systems, 19:263–272, 2018.
B. Baheti, S. Innani, S. Gajre and S. Talbar, "Eff-UNet: A Novel Architecture for Semantic Segmentation in Unstructured Environment," 2020 IEEE/CVF Conference on Computer Vision and Pattern Recognition Workshops (CVPRW), 2020, pp. 1473-1481, doi: 10.1109/CVPRW50498.2020.00187.
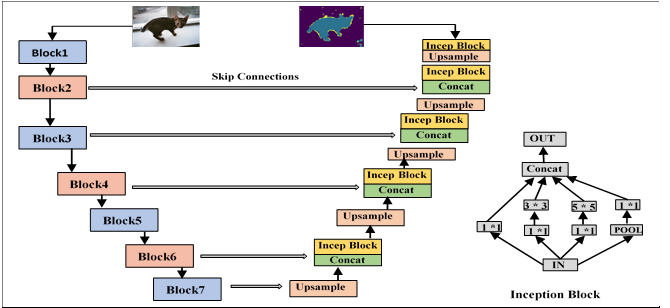
Downloads
Published
How to Cite
Issue
Section
License
Copyright (c) 2023 Shubha Rao A., Mahantesh K.

This work is licensed under a Creative Commons Attribution-ShareAlike 4.0 International License.
All papers should be submitted electronically. All submitted manuscripts must be original work that is not under submission at another journal or under consideration for publication in another form, such as a monograph or chapter of a book. Authors of submitted papers are obligated not to submit their paper for publication elsewhere until an editorial decision is rendered on their submission. Further, authors of accepted papers are prohibited from publishing the results in other publications that appear before the paper is published in the Journal unless they receive approval for doing so from the Editor-In-Chief.
IJISAE open access articles are licensed under a Creative Commons Attribution-ShareAlike 4.0 International License. This license lets the audience to give appropriate credit, provide a link to the license, and indicate if changes were made and if they remix, transform, or build upon the material, they must distribute contributions under the same license as the original.