A New Hybrid CNN-LSTM Model for the X-Ray Image-Based Detection of Paediatric Croup Cough Disease
Keywords:
COVID-19, CNN, pertinent, achieves, alternative, insufficientAbstract
The importance of computer-based illness detection has grown recently, especially in the medical field where radiological imaging methods like X-ray, CT, and MRI are essential for identifying a wide range of ailments. The development of the Coronavirus (COVID-19) has tested the limits of medical innovation. Due to the similarity in symptoms, it can be difficult for doctors to differentiate COVID-19 from respiratory diseases such croup, bronchitis, and laryngitis. The primary age range for children who contract croup is between six months and three years old. However, croup and COVID-19 symptoms frequently coincide. Due to the insufficient availability of pertinent information in medical pictures, x-ray-based croup detection is not frequently used, therefore an improved computer-based detection method offers a viable alternative for early diagnosis. In this context, we suggest a hybrid model for the categorization of croup cough that combines Convolutional Neural Network (CNN) and Long Short-Term Memory (LSTM). The LSTM is used for classification, whereas the CNN is used for feature extraction. Based on the experimental findings, the combined CNN-LSTM model, although being trained on a very short dataset, achieves an amazing testing accuracy of 97.66%.
Downloads
References
Lars J Grimm, MD, MHS Assistant Professor, Department of Diagnostic Radiology, Duke University Medical Center, https://emedicine.medscape.com/article/407964-overview
Almendra M, Pereira MP, Gonçalves CS, Bonfadini M, Brites V, Estrada JF. Croup and COVID-19. J Paediatr Child Health. 2022 Sep;58(9):1691-1692. doi: 10.1111/jpc.15987. Epub 2022 Apr 16. PMID: 35429196; PMCID: PMC9115154.
Guttmann, A., Weinstein, M., Austin, P., Bhamani, A., & Anderson, G. (2013). Variability in the emergency department use of discretionary radiographs in children with common respiratory conditions: The mixed effect of access to pediatrician care. Canadian Journal of Emergency Medicine, 15(1), 8-17. doi:10.2310/8000.2012.120649
Lakshmanaprabu SK, Mohanty SN, Shankar K, Arunkumar N, Ramirez G. Optimal deep learning model for classification of lung cancer on CT images. Future Generat Comput Syst 2019; 92:374–82. https://doi.org/10.1016/j.future.2018.10.009.
Jiang X. Feature extraction for image recognition and computer vision. In: Proc. - 2009 2nd IEEE Int. Conf. Comput. Sci. Inf. Technol. ICCSIT. vol. 2009; 2009. p. 1–15. https://doi.org/10.1109/ICCSIT.2009.5235014
Chen Y, Gou X, Feng X, Liu Y, Qin G, Feng Q, Yang W, Chen W. Bone suppression of chest radiographs with cascaded convolutional networks in wavelet domain. IEEE Access 2019;7:83 46–57. https://doi.org/10.1109/ACCESS.2018.2890300.
Rahimzadeh M, Attar A. A new modified deep convolutional neural network for detecting COVID-19 from X-ray images. http://arxiv.org/abs/2004.08052; 2020.
Apostolopoulos ID, Mpesiana TA. Covid-19: automatic detection from X-ray images utilizing transfer learning with convolutional neural networks. Phys Eng Sci Med 2020:1–8. https://doi.org/10.1007/s13246-020-00865-4.
Horry MJ, Chakraborty S, Paul M, Ulhaq A, Pradhan B. X-ray image based COVID 19 detection using pre-trained deep learning models. 2007.
Loey M, Smarandache F, Khalifa NEM. Within the lack of chest COVID-19 X-ray dataset: a novel detection model based on GAN and deep transfer learning. Symmetry (Basel) 2020;12:651. https://doi.org/10.3390/sym12040651.
Singh M, Bansal S. Transfer learning based ensemble support vector machine model for automated COVID-19 detection using lung computerized tomography scan data. https://assets.researchsquare.com/files/rs-32493/v1_stamped.pdf; 2020.
Shahakar, M. ., Mahajan, S. ., & Patil, L. . (2023). Load Balancing in Distributed Cloud Computing: A Reinforcement Learning Algorithms in Heterogeneous Environment. International Journal on Recent and Innovation Trends in Computing and Communication, 11(2), 65–74. https://doi.org/10.17762/ijritcc.v11i2.6130
Fong SJ, Li G, Dey N, Crespo RG, Herrera-Viedma E. Composite Monte Carlo decision making under high uncertainty of novel coronavirus epidemic using hybridized deep learning and fuzzy rule induction. Appl Soft Comput J 2020;93. https://doi.org/10.1016/j.asoc.2020.106282.
Bandyopadhyay SK, Dutta S. Machine learning approach for confirmation of COVID-19 cases: positive, negative. MedRxiv: Death and Release; 2020. https:// doi.org/10.1101/2020.03.25.20043505. 2020.03.25.20043505.
Khan AI, Shah JL, Bhat M. CoroNet: a deep neural network for detection and diagnosis of covid-19 from chest X-ray images. http://arxiv.org/abs/2004.04931; 2020.
Kermany, Daniel; Zhang, Kang; Goldbaum, Michael (2018), “Labeled Optical Coherence Tomography (OCT) and Chest X-Ray Images for Classification”, Mendeley Data, v2
http://dx.doi.org/10.17632/rscbjbr9sj.2
Croup by Armando HF Websites https://armandoh.org/disease/paediatrics-croup/
Rodney B. Boychuk, M.D., Drooling, Stridor, and a Barking Cough: Croup?? Radiology Cases in Pediatric Emergency Medicine Volume 1, Case 10 Kapiolani Medical Center For Women And Children University of Hawaii John A. Burns School of Medicine https://www.hawaii.edu/medicine/pediatrics/pemxray/v1c10.html
Germaine L Defendi, MD, MS, FAAP Associate Clinical Professor, Department of Pediatrics, Olive View-UCLA Medical centre, https://emedicine.medscape.com/article/962972-overview
Stanley Oiseth, Lindsay Jones, Evelin Maza, Croup Cough, The Lecturio Medical Concept Library https://www.lecturio.com/concepts/croup/
Gaillard, F., Murphy, A. Croup. Reference article, Radiopaedia.org. (accessed on 19 May 2022) https://doi.org/10.53347/rID-1185
Chaudhary, D. S. . (2021). ECG Signal Analysis for Myocardial Disease Prediction by Classification with Feature Extraction Machine Learning Architectures. Research Journal of Computer Systems and Engineering, 2(1), 06:10. Retrieved from https://technicaljournals.org/RJCSE/index.php/journal/article/view/12
Galvez RL, Bandala AA, Dadios EP, Vicerra RRP, Maningo JMZ. Object detection using convolutional neural networks. In: IEEE Reg. 10 Annu. Int. Conf. Proceedings/TENCON. Institute of Electrical and Electronics Engineers Inc.; 2019.p. 2023–7. https://doi.org/10.1109/TENCON.2018.8650517.
Tajbakhsh N, Shin JY, Gurudu SR, Hurst RT, Kendall CB, Gotway MB, Liang J. Convolutional neural networks for medical image analysis: full training or fine tuning? IEEE Trans Med Imag 2016; 35:1299–312. https://doi.org/10.1109/TMI.2016.2535302.
Md. Zabirul Islam, Md. Milon Islam, Amanullah Asraf, A combined deep CNN-LSTM network for the detection of novel coronavirus (COVID-19) using X-ray images, Informatics in medicine Unlocked, Volume 20, 2020, 100412,ISSN 2352-9148, https://doi.org/10.1016/j.imu.2020.100412.
Iqbal, S., N. Qureshi, A., Li, J. et al. On the Analyses of Medical Images Using Traditional Machine Learning Techniques and Convolutional Neural Networks. Arch Computat Methods Eng 30, 3173–3233 (2023). https://doi.org/10.1007/s11831-023-09899-9
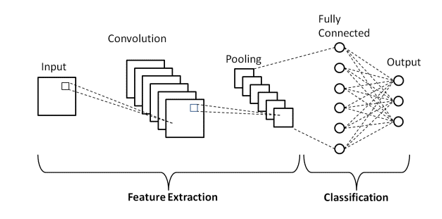
Downloads
Published
How to Cite
Issue
Section
License

This work is licensed under a Creative Commons Attribution-ShareAlike 4.0 International License.
All papers should be submitted electronically. All submitted manuscripts must be original work that is not under submission at another journal or under consideration for publication in another form, such as a monograph or chapter of a book. Authors of submitted papers are obligated not to submit their paper for publication elsewhere until an editorial decision is rendered on their submission. Further, authors of accepted papers are prohibited from publishing the results in other publications that appear before the paper is published in the Journal unless they receive approval for doing so from the Editor-In-Chief.
IJISAE open access articles are licensed under a Creative Commons Attribution-ShareAlike 4.0 International License. This license lets the audience to give appropriate credit, provide a link to the license, and indicate if changes were made and if they remix, transform, or build upon the material, they must distribute contributions under the same license as the original.