Spiking Neural Network based Water management in Drought Area of Maharashtra India: A Case Study of Osmanabad District
Keywords:
Spiking Neural Network, Standard Precipitation Index, Drought Index, Metrological Drought Index, Drought Monitoring, Mean Drought Intensity, Drought MagnitudeAbstract
This paper proposes a machine learning technique for drought planning and risk reduction in agricultural regions dominated by smallholders. The proposed machine learning technique is Spiking Neural Network (SNN). Analysis of spatiotemporal features and patterns of meteorological drought at resolution is the major goal of the proposed control technique, which will help local risk planning and mitigation decisions as well as activities. Using publically accessible coarse scale data, the proposed method is employed in this study to produce high-resolution gridded precipitation products, which are then downscaled for investigation of meteorological droughts. Meteorological drought is defined by the Standard Precipitation Index (SPI). The purpose of the SNN for predicts the rainfall data resolution. By using coarse-scale precipitation data as a starting point for the high-resolution, spatiotemporal analysis of droughts, the proposed method is applied and adequately general to be adopted in other areas to enable local drought risk planning and targeted mitigation-decisions as well as movements. The proposed model is run on the MATLAB/Simulink platform, and the performance is evaluated using the existing methods. The proposed method helps to predict the drought and it has more success rate than the existing methods. The efficiency of proposed method is 0.9 which is way better than the existing techniques.
Downloads
References
A. AghaKouchak, A. Farahmand, F. S. Melton, J. Teixeira, M. C. Anderson, B. D. Wardlow, and C. R. Hain, 2015. Remote sensing of drought: Progress, challenges and opportunities. Reviews of Geophysics, 53(2), pp.452-480.
J. Bai, Q. Cui, D. Chen, H. Yu, X. Mao, L. Meng, and Y. Cai, 2018. Assessment of the SMAP-derived soil water deficit index (SWDI-SMAP) as an agricultural drought index in China. Remote Sensing, 10(8), p.1302.
J. P. Bloomfield and B. P. Marchant, 2013. Analysis of groundwater drought building on the standardised precipitation index approach. Hydrology and Earth System Sciences, 17(12), pp.4769-4787.
K. Devdatta Mokashi, and Prof. (Dr.) Vidula S. Sohoni, 2021. Identification of Water Conservation Zone By Application Of Electrical Resistivity Method In Parts of Osmanabad District, Maharashtra-India, International Journal of Engineering Trends and Technology, 69(3), pp.233-238.
T. Che, X. Li, R. Jin, R. Armstrong, and T. Zhang, 2008. Snow depth derived from passive microwave remote-sensing data in China. Annals of Glaciology, 49, pp.145-154.
Y. Chen, K. Yang, J. He, J. Qin, J. Shi, J. Du, and Q.He, 2011. Improving land surface temperature modeling for dry land of China. Journal of Geophysical Research: Atmospheres, 116(D20).
R. Li, A. Tsunekawa, and M. Tsubo, 2014. Index-based assessment of agricultural drought in a semi-arid region of Inner Mongolia, China. Journal of Arid Land, 6, pp.3-15.
F. N. Kogan, 1990. Remote sensing of weather impacts on vegetation in non-homogeneous areas. International Journal of remote sensing, 11(8), pp.1405-1419.
M. Kędzior and J. Zawadzki, 2016. Comparative study of soil moisture estimations from SMOS satellite mission, GLDAS database, and cosmic-ray neutrons measurements at COSMOS station in Eastern Poland. Geoderma, 283, pp.21-31.
G. Wong, H. A. J. van Lanen, and P. J. J. F. Torfs. 2013. Probabilistic analysis of hydrological drought characteristics using meteorological drought. Hydrological Sciences Journal, 58(2), pp.253-270.
A. K. Qin, V. L. Huang, and P. N. Suganthan, 2008. Differential evolution algorithm with strategy adaptation for global numerical optimization. IEEE transactions on Evolutionary Computation, 13(2), pp.398-417.
B. Sivakumar, 2011. Global climate change and its impacts on water resources planning and management: assessment and challenges. Stochastic Environmental Research and Risk Assessment, 25, pp.583-600.
S. Adarsh and M. Janga Reddy, 2015. Trend analysis of rainfall in four meteorological subdivisions of southern India using nonparametric methods and discrete wavelet transforms. International journal of Climatology, 35(6), pp.1107-1124.
P. Guhathakurta, and E.Saji, 2013. Detecting changes in rainfall pattern and seasonality index vis-à-vis increasing water scarcity in Maharashtra. Journal of Earth System Science, 122, pp.639-649.
J. Fito, N. Tefera, S. Demeku, and H. Kloos, 2017. Water footprint as an emerging environmental tool for assessing sustainable water use of the bioethanol distillery at Metahara sugarcane farm, Oromiya Region, Ethiopia. Water Conservation Science and Engineering, 2, pp.165-176.
A.Yinglan, G. Wang, T. Liu, S.Shrestha, B.Xue, and Z.Tan, 2019. Vertical variations of soil water and its controlling factors based on the structural equation model in a semi-arid grassland. Science of the Total Environment, 691, pp.1016-1026.
J. Rhee, J. Im, and G. J. Carbone, 2010. Monitoring agricultural drought for arid and humid regions using multi-sensor remote sensing data. Remote Sensing of environment, 114(12), pp.2875-2887.
K. E. Saxton and W. J. Rawls, 2006. Soil water characteristic estimates by texture and organic matter for hydrologic solutions. Soil science society of America Journal, 70(5), pp.1569-1578.
W. Shangguan, Y. Dai, Q. Duan, B. Liu, and H. Yuan, 2014. A global soil data set for earth system modeling. Journal of Advances in Modeling Earth Systems, 6(1), pp.249-263.
S. Shukla and A. W. Wood, 2008. Use of a standardized runoff index for characterizing hydrologic drought. Geophysical research letters, 35(2).
P. C. Spennemann, J. A. Rivera, A. C. Saulo, and O. C. Penalba, 2015. A comparison of GLDAS soil moisture anomalies against standardized precipitation index and multisatellite estimations over South America. Journal of Hydrometeorology, 16(1), pp.158-171.
K. Zhou, J. Li, T. Zhang, and A. Kang.2021. The use of combined soil moisture data to characterize agricultural drought conditions and the relationship among different drought types in China. Agricultural Water Management, 243, p.106479.
M. I. Hossain, M. N. Bari, S. U. Miah, A.-Kafy, and N. M. R. Nasher, 2021. Application of modified managed aquifer recharge model for groundwater management in drought-prone water-stressed Barind Tract, Bangladesh. Environmental Challenges, 4, p.100173.
M. Hiloidhari, V. Vijay, R. Banerjee, D. C. Baruah, and A. B. Rao, 2021. Energy-carbon-water footprint of sugarcane bioenergy: A district-level life cycle assessment in the state of Maharashtra, India. Renewable and Sustainable Energy Reviews, 151, p.111583.
N. Neeti, C. M. ArunMurali, V. M. Chowdary, N. H. Rao, and M. Kesarwani, 2021. Integrated meteorological drought monitoring framework using multi-sensor and multi-temporal earth observation datasets and machine learning algorithms: A case study of central India. Journal of Hydrology, 601, p.126638.
P. Quintana-Seguí, A. Barella-Ortiz, S. Regueiro-Sanfiz, and G. Miguez-Macho, 2020. The utility of land-surface model simulations to provide drought information in a water management context using global and local forcing datasets. Water Resources Management, 34, pp.2135-2156.
S. M. Ahmed, 2020. Impacts of drought, food security policy and climate change on performance of irrigation schemes in Sub-saharan Africa: The case of Sudan. Agricultural Water Management, 232, p.106064.
S. Nandi and J. R. Manne, 2020. Spatiotemporal analysis of water balance components and their projected changes in near-future under climate change over Sina Basin, India. Water Resources Management, 34, pp.2657-2675.
S. Adarsh and M. Janga Reddy, 2015. Trend analysis of rainfall in four meteorological subdivisions of southern India using nonparametric methods and discrete wavelet transforms. International journal of Climatology, 35(6), pp.1107-1124.
A. Ahmadalipour, H. Moradkhani, and M. Svoboda, 2017. Centennial drought outlook over the CONUS using NASA‐NEX downscaled climate ensemble. International Journal of Climatology, 37(5), pp.2477-2491.
Y. Bao and X. Wen, 2017. Projection of China’s near-and long-term climate in a new high-resolution daily downscaled dataset NEX-GDDP. Journal of Meteorological Research, 31(1), pp.236-249.
S. N. Gosling, R. G. Taylor, N. W. Arnell, and M. C. Todd, 2011. A comparative analysis of projected impacts of climate change on river runoff from global and catchment-scale hydrological models. Hydrology and Earth System Sciences, 15(1), pp.279-294.
W. O. Ochola and P. Kerkides, 2003. A Markov chain simulation model for predicting critical wet and dry spells in Kenya: analysing rainfall events in the Kano Plains. Irrigation and Drainage: The journal of the International Commission on Irrigation and Drainage, 52(4), pp.327-342.
X. Liang, D. P. Lettenmaier, E. F. Wood, and S. J. Burges, 1994. A simple hydrologically based model of land surface water and energy fluxes for general circulation models. Journal of Geophysical Research: Atmospheres, 99(D7), pp.14415-14428.
J. Abolverdi and D. Khalili, 2010. Probabilistic analysis of extreme regional meteorological droughts by L-moments in a semi-arid environment. Theoretical and Applied Climatology, 102, pp.351-366.
D. C. Edossa, M. S. Babel, and A. Das Gupta, 2010. Drought analysis in the Awash river basin, Ethiopia. Water resources management, 24, pp.1441-1460.
V. K. Lohani and G. V. Loganathan, 1997. An early warning system for drought management using the palmer drought index 1. JAWRA Journal of the American Water Resources Association, 33(6), pp.1375-1386.
V. K. Lohani, G. V. Loganathan, and S. Mostaghimi, 1998. Long-term analysis and short-term forecasting of dry spells by Palmer Drought Severity Index. Hydrology Research, 29(1), pp.21-40.
Verma, R. ., Dhanda, N. ., & Nagar, V. . (2023). Analysing the Security Aspects of IoT using Blockchain and Cryptographic Algorithms. International Journal on Recent and Innovation Trends in Computing and Communication, 11(1s), 13–22. https://doi.org/10.17762/ijritcc.v11i1s.5990
A. K. Mishra, V. R. Desai, and V. P. Singh, 2007. Drought forecasting using a hybrid stochastic and neural network model. Journal of Hydrologic Engineering, 12(6), pp.626-638.
R. Modarres, 2010. Regional dry spells frequency analysis by L-moment and multivariate analysis. Water resources management, 24(10), pp.2365-2380.
S. Morid, V. Smakhtin, and M. Moghaddasi, 2006. Comparison of seven meteorological indices for drought monitoring in Iran. International Journal of Climatology: A Journal of the Royal Meteorological Society, 26(7), pp.971-985.
M. Abedinpour, A. Sarangi, T. B. S. Rajput, M. Singh, H. Pathak, and T. Ahmad, 2012. Performance evaluation of AquaCrop model for maize crop in a semi-arid environment. Agricultural Water Management, 110, pp.55-66.
C. Accadia, S. Mariani, M. Casaioli, A. Lavagnini, and A. Speranza, 2005. Verification of precipitation forecasts from two limited-area models over Italy and comparison with ECMWF forecasts using a resampling technique. Weather and Forecasting, 20(3), pp.276-300.
Mondal , D. (2021). Green Channel Roi Estimation in The Ovarian Diseases Classification with The Machine Learning Model . Machine Learning Applications in Engineering Education and Management, 1(1), 07–12.
A.Arabameri, O.AsadiNalivan, S. Chandra Pal, R.Chakrabortty, A.Saha, S. Lee, B.Pradhan, and TienD.Bui, 2020. Novel machine learning approaches for modelling the gully erosion susceptibility. Remote Sensing, 12(17), p.2833.
K. Chandrasekar, M. V. Sai, P. S. Roy, V. Jayaraman, and R. R. Krishnamurthy, 2009. Identification of agricultural drought vulnerable areas of Tamil Nadu, India--using GIS based multi criteria analysis. Asian Journal of Environment and Disaster Management, 1(1).
H. Chen, S.Guo, C.-yuXu, and V. P. Singh, 2007. Historical temporal trends of hydro-climatic variables and runoff response to climate variability and their relevance in water resource management in the Hanjiang basin. Journal of hydrology, 344(3-4), pp.171-184.
J. Cheng and J.-ping Tao, 2010. Fuzzy comprehensive evaluation of drought vulnerability based on the analytic hierarchy process:—an empirical study from Xiaogan City in Hubei Province. Agriculture and Agricultural Science Procedia, 1, pp.126-135.
S. S. Cooley, C. A. Williams, J. B. Fisher, G. H. Halverson, J. Perret, and C. M. Lee, 2019. Assessing regional drought impacts on vegetation and evapotranspiration: a case study in Guanacaste, Costa Rica. Ecological applications, 29(2), p.e01834.
K. L. Ebi and K. Bowen, 2016. Extreme events as sources of health vulnerability: Drought as an example. Weather and climate extremes, 11, pp.95-102.
A. Gayen, S. M. Haque, and S. Saha, 2020. Modeling of gully erosion based on random forest using GIS and R. Gully Erosion Studies from India and Surrounding Regions, pp.35-44.
C. Liu, W. Shen, L. Zhang, Y. Du, and Z. Yuan, 2021. Spike neural network learning algorithm based on an evolutionary membrane algorithm. IEEE Access, 9, pp.17071-17082.
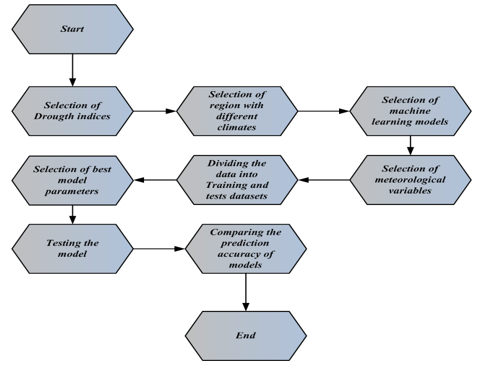
Downloads
Published
How to Cite
Issue
Section
License

This work is licensed under a Creative Commons Attribution-ShareAlike 4.0 International License.
All papers should be submitted electronically. All submitted manuscripts must be original work that is not under submission at another journal or under consideration for publication in another form, such as a monograph or chapter of a book. Authors of submitted papers are obligated not to submit their paper for publication elsewhere until an editorial decision is rendered on their submission. Further, authors of accepted papers are prohibited from publishing the results in other publications that appear before the paper is published in the Journal unless they receive approval for doing so from the Editor-In-Chief.
IJISAE open access articles are licensed under a Creative Commons Attribution-ShareAlike 4.0 International License. This license lets the audience to give appropriate credit, provide a link to the license, and indicate if changes were made and if they remix, transform, or build upon the material, they must distribute contributions under the same license as the original.