Classification of Uncertain ImageNet Retinal Diseases using ResNet Model
Keywords:
Convolutional Neural Networks (CNN), Image Processing, uncertain ImageNet dataset, pretrained modelAbstract
An automated method is required to detect retinal diseases. The goal of this study is to build automated screening methods for retinopathy (DR) and other eye diseases using deep learning. In this study present an approach for detecting retinal illness using a CNN. In order to find these patterns, CNN may be employed by extracting features from the data. A color Fundus image-based technique is proposed by the authors of this study to detect retinal disorders. Without prompt diagnosis and treatment, retinal disorders may cause permanent vision loss. The condition must be diagnosed at an early stage in order to get the right therapy and cure it. Deep learning models may be used to train and test the data in order to classify different retinal disorders, where several common retinal diseases and conditions are classified and normalized. This study looked at how retinal pictures may be used to classify eye illnesses using CNN. Over eight different retinal illnesses are included in this dataset, which applied a CNN model to Pretrained on Classification of uncertain ImageNet Retinal Diseases using ResNet Model has been implemented, CNN is built for retina pictures with varying task functions and depths. Various filtering and pooling strategies are tried and shown to have a significant impact on network performance. This is possible because employing a convolutional neural network to process the retinal pictures. It has been discovered that this suggested method has an accuracy rate of more than 80%.
Downloads
References
Adel, A., Soliman, M. M., Khalifa, N. E. M., & Mostafa, K. (2020, December). Automatic Classification of Retinal Eye Diseases from Optical Coherence Tomography using Transfer Learning. In 2020 16th International Computer Engineering Conference (ICENCO) (pp. 37-42). IEEE.
Bhadra, R., & Kar, S. (2020, October). Retinal Disease Classification from Optical Coherence Tomographical Scans using Multilayered Convolution Neural Network. In 2020 IEEE Applied Signal Processing Conference (ASPCON) (pp. 212-216). IEEE..
Berrimi, M., & Moussaoui, A. (2020, October). Deep learning for identifying and classifying retinal diseases. In 2020 2nd International Conference on Computer and Information Sciences (ICCIS) (pp. 1-6). IEEE..
El‐Hag, N. A., Sedik, A., El‐Shafai, W., El‐Hoseny, H. M., Khalaf, A. A., El‐Fishawy, A. S., ... & El‐Banby, G. M. (2021). Classification of retinal images based on convolutional neural network. Microscopy Research and Technique, 84(3), 394-414..
Hassan, B., Qin, S., & Ahmed, R. (2020, December). RRI-Net: classification of multi-class retinal diseases with deep recurrent residual inception network using OCT scans. In 2020 IEEE International Symposium on Signal Processing and Information Technology (ISSPIT) (pp. 1-6). IEEE..
Das, A., Giri, R., Chourasia, G., & Bala, A. A. (2019, July). Classification of retinal diseases using transfer learning approach. In 2019 International Conference on Communication and Electronics Systems (ICCES) (pp. 2080-2084). IEEE..
Hasan, M. K., Tanha, T., Amin, M. R., Faruk, O., Khan, M. M., Aljahdali, S., & Masud, M. (2021). Cataract Disease Detection by Using Transfer Learning-Based Intelligent Methods. Computational and Mathematical Methods in Medicine, 2021..
Noronha, K., Navya, K. T., & Nayak, K. P. (2012, December). Support system for the automated detection of hypertensive retinopathy using fundus images. In International Conference on Electronic Design and Signal Processing (ICEDSP) (pp. 7-11)..
Xiao, Z., Zhang, X., Geng, L., Zhang, F., Wu, J., Tong, J., ... & Shan, C. (2017). Automatic non-proliferative diabetic retinopathy screening system based on color fundus image. Biomedical engineering online, 16(1), 1-19..
He, K., Zhang, X., Ren, S., & Sun, J. (2016). Deep residual learning for image recognition. In Proceedings of the IEEE conference on computer vision and pattern recognition (pp. 770-778)..
Shorten, C., & Khoshgoftaar, T. M. (2019). A survey on image data augmentation for deep learning. Journal of big data, 6(1), 1-48..
Priyanka Konatham, Mounika Venigalla, Lakshmi Pooja Amaraneni, K. Suvarna Vani, “ Automatic Detection of Optic Disc for Diabetic Retinopathy”, IJITEEE,May 2020.
Maryada, S. K. R., Booker, W. L., Danala, G., Ha, C. A., Mudduluru, S., Hougen, D. F., & Zheng, B. (2022, April). Applying a novel two-stage deep-learning model to improve accuracy in detecting retinal fundus images. In Medical Imaging 2022: Computer-Aided Diagnosis (Vol. 12033, pp. 135-142). SPIE.
Subramanian, M., Kumar, M. S., Sathishkumar, V. E., Prabhu, J., Karthick, A., Ganesh, S. S., & Meem, M. A. (2022). Diagnosis of retinal diseases based on Bayesian optimization deep learning network using optical coherence tomography images. Computational Intelligence and Neuroscience, 2022.
Sunija, A. P., Kar, S., Gayathri, S., Gopi, V. P., & Palanisamy, P. (2021). Octnet: A lightweight cnn for retinal disease classification from optical coherence tomography images. Computer methods and programs in biomedicine, 200, 105877.
Elsharif, A. A. E. F., & Abu-Naser, S. S. (2022). Retina Diseases Diagnosis Using Deep Learning. International Journal of Academic Engineering Research (IJAER), 6(2).
Saikumar, K., Rajesh, V. A machine intelligence technique for predicting cardiovascular disease (CVD) using Radiology Dataset. Int J Syst Assur Eng Manag (2022). https://doi.org/10.1007/s13198-022-01681-7
Ke, A., Ellsworth, W., Banerjee, O., Ng, A. Y., & Rajpurkar, P. (2021, April). CheXtransfer: performance and parameter efficiency of ImageNet models for chest X-Ray interpretation. In Proceedings of the Conference on Health, Inference, and Learning (pp. 116-124).
Gelman, R., & Fernandez-Granda, C. (2022). Analysis of Transfer Learning for Select Retinal Disease Classification. Retina, 42(1), 174-183.
Ge, Z., & Wang, X. (2021). Evaluation of Various Open-Set Medical Imaging Tasks with Deep Neural Networks. arXiv preprint arXiv:2110.10888.
Mythreya, S., Murthy, A. S. D., Saikumar, K., & Rajesh, V. (2022). Prediction and Prevention of Malicious URL Using ML and LR Techniques for Network Security: Machine Learning. In Handbook of Research on Technologies and Systems for E-Collaboration During Global Crises (pp. 302-315). IGI Global.
Saikumar, K., Rajesh, V., Babu, B.S. (2022). Heart disease detection based on feature fusion technique with augmented classification using deep learning technology. Traitement du Signal, Vol. 39, No. 1, pp. 31-42. https://doi.org/10.18280/ts.390104
Kailasam, S., Achanta, S.D.M., Rama Koteswara Rao, P., Vatambeti, R., Kayam, S. (2022). An IoT-based agriculture maintenance using pervasive computing with machine learning technique. International Journal of Intelligent Computing and Cybernetics, 15(2), pp. 184–197
Nagendram, S., Nag, M. S. R. K., Ahammad, S. H., Satish, K., & Saikumar, K. (2022, January). Analysis For The System Recommended Books That Are Fetched From The Available Dataset. In 2022 4th International Conference on Smart Systems and Inventive Technology (ICSSIT) (pp. 1801-1804). IEEE.
Jothsna, V., Patel, I., Raghu, K., Jahnavi, P., Reddy, K. N., & Saikumar, K. (2021, March). A Fuzzy Expert System for The Drowsiness Detection from Blink Characteristics. In 2021 7th International Conference on Advanced Computing and Communication Systems (ICACCS) (Vol. 1, pp. 1976-1981). IEEE.
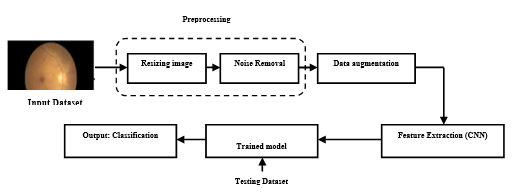
Downloads
Published
How to Cite
Issue
Section
License
Copyright (c) 2022 Lohitha Boyina, Karthik Boddu, Yamini Tankasala, K. Suvarna Vani

This work is licensed under a Creative Commons Attribution-ShareAlike 4.0 International License.
All papers should be submitted electronically. All submitted manuscripts must be original work that is not under submission at another journal or under consideration for publication in another form, such as a monograph or chapter of a book. Authors of submitted papers are obligated not to submit their paper for publication elsewhere until an editorial decision is rendered on their submission. Further, authors of accepted papers are prohibited from publishing the results in other publications that appear before the paper is published in the Journal unless they receive approval for doing so from the Editor-In-Chief.
IJISAE open access articles are licensed under a Creative Commons Attribution-ShareAlike 4.0 International License. This license lets the audience to give appropriate credit, provide a link to the license, and indicate if changes were made and if they remix, transform, or build upon the material, they must distribute contributions under the same license as the original.