Improving the Software Privacy in the OFDM 5G Communication Integrated with License Key in the Hardware Communication Parameters
Keywords:
5G communication, license key, Discrete Wavelet transform, OFDMAbstract
Over several decades, wireless multi-media applications demand for higher data rate information transmission which exhibits the drastic advancement in the connectivity, mobility and scalability of the network. The user of wireless comprises to fulfill the requirement of users with the problem of limited available Radio frequency (RF) for the varying signal strength in the multipath fading system. However, the 5G wireless communication technology uses the OFDM technology for effective information transmission. Even though the OFDM system exhibits the improved performance in terms of delay, fairness and maximization of the throughput. The conventional OFDM scheme subjected to challenge of ICI and license key. It is necessary to reduce the license key in the OFDM signal with technique such as License Key Partial Transmit sequences (LKPTS), Clipping, Selective Mapping (SLM) and block coding. The conventional technique exhibits the reduction in license key for the higher signal transmit power, BER and higher computational complexity. Among different license key reduction scheme LKPTS is flexible and effective for the varying number of subcarriers in the suitable modulation scheme. In this paper developed a modified partial transmit sequence (MLKPTS) for the reduction of license key in the OFDM signal. In the proposed MLKPTS scheme the sequences are trained based on the superimpose of the OFDM signal for resource allocation. The developed MLKPTS scheme uses the low band and high band estimated Discrete Wavelet transform (DWT). The MLKPTS uses the pulse shaping and interleaving for the selection of the sub carriers in the signal sequences. The pulse shapes are computed and evaluated based on the cyclic drift in the signal pulse sequences. The developed PAR computes the transmitted signal amplitude based on the in-phase and quadrature phase components. The simulation analysis expressed that the proposed MLKPTS scheme significantly reduces the license key in the OFDM signal sequences.
Downloads
References
Hussain, G. A., & Audah, L. (2021). A BCS code in the f-OFDM system: a promising candidate for 5G wireless communication systems. Journal of Ambient Intelligence and Humanized Computing, 1-10.
Hammed, Z. S., Ameen, S. Y., & Zeebaree, S. R. (2021, September). Massive MIMO-OFDM performance enhancement on 5G. In 2021 International Conference on Software, Telecommunications and Computer Networks (SoftCOM) (pp. 1-6). IEEE.
Mathur, H., & Deepa, T. (2021). A survey on advanced multiple access techniques for 5G and beyond wireless communications. Wireless Personal Communications, 118(2), 1775-1792.
Singh, M., Pottoo, S. N., Malhotra, J., Grover, A., & Aly, M. H. (2021). Millimeter-wave hybrid OFDM-MDM radio over free space optical transceiver for 5G services in desert environment. Alexandria Engineering Journal, 60(5), 4275-4285.
Rawat, A., Kaushik, R., & Tiwari, A. (2021). An Overview Of MIMO OFDM System For Wireless Communication. International Journal of Technical Research & Science, 6(X), 1-4.
Pandya, S., Wakchaure, M. A., Shankar, R., & Annam, J. R. (2021). Analysis of NOMA-OFDM 5G wireless system using deep neural network. The Journal of Defense Modeling and Simulation, 1548512921999108.
Al-Gharabally, M., Almutairi, A. F., & Krishna, A. (2021). Performance analysis of the two-piecewise linear companding technique on filtered-OFDM systems. IEEE Access, 9, 48793-48802.
Kansal, L., Gaba, G. S., Chilamkurti, N., & Kim, B. G. (2021). Efficient and Robust OFDM signal Communication Techniques for 5G Applications in Smart Cities. Energies, 14(13), 3986.
Kansal, L., Berra, S., Mounir, M., Miglani, R., Dinis, R., & Rabie, K. (2022). Performance Analysis of Massive MIMO-OFDM System Incorporated with Various Transforms for OFDM signal Communication in 5G Systems. Electronics, 11(4), 621.
Sarowa, S., Kumar, S., Rathee, D. S., & Kumar, N. (2021). Performance Analysis of Improved Self Cancellation Technique for Wavelet Based OFDM Through Simulink Model. Wireless Personal Communications, 118(2), 1055-1073.
Liu, J., Mei, K., Zhang, X., Ma, D., & Wei, J. (2019). Online extreme learning machine-based channel estimation and equalization for OFDM systems. IEEE Communications Letters, 23(7), 1276-1279.
Yi, X., & Zhong, C. (2020). Deep learning for joint channel estimation and signal detection in OFDM systems. IEEE Communications Letters, 24(12), 2780-2784.
Jiang, R., Wang, X., Cao, S., Zhao, J., & Li, X. (2019). Deep neural networks for channel estimation in underwater acoustic OFDM systems. IEEE access, 7, 23579-23594.
Mashhadi, M. B., & Gündüz, D. (2021). Pruning the pilots: Deep learning-based pilot design and channel estimation for MIMO-OFDM systems. IEEE Transactions on Wireless Communications, 20(10), 6315-6328.
Wang, B., Jian, M., Gao, F., Li, G. Y., & Lin, H. (2019). Beam squint and channel estimation for wideband mmWave massive MIMO-OFDM systems. IEEE Transactions on Signal Processing, 67(23), 5893-5908.
Soltani, M., Pourahmadi, V., & Sheikhzadeh, H. (2020). Pilot pattern design for deep learning-based channel estimation in OFDM systems. IEEE Wireless Communications Letters, 9(12), 2173-2176.
Unnisa, N., & Tatineni, M. (2022). Adaptive deep learning strategy with red deer algorithm for sparse channel estimation and hybrid precoding in millimeter wave massive MIMO-OFDM systems. Wireless Personal Communications, 122(4), 3019-3051.
Jiang, P., Wen, C. K., Jin, S., & Li, G. Y. (2021). Dual CNN-Based Channel Estimation for MIMO-OFDM Systems. IEEE Transactions on Communications, 69(9), 5859-5872.
Lin, Y., Jin, S., Matthaiou, M., & You, X. (2021). Channel estimation and user localization for IRS-assisted MIMO-OFDM systems. IEEE Transactions on Wireless Communications.
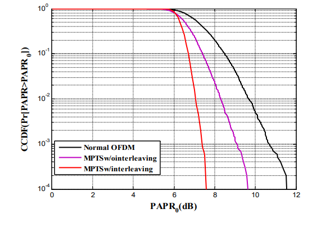
Downloads
Published
How to Cite
Issue
Section
License
Copyright (c) 2022 Avadhesh Kumar, M. Sunil Kumar, Pradnya A. Vikhar, Venkat Ghodke, Rajesh Rupchand Waghulde, Mansing Rathod

This work is licensed under a Creative Commons Attribution-ShareAlike 4.0 International License.
All papers should be submitted electronically. All submitted manuscripts must be original work that is not under submission at another journal or under consideration for publication in another form, such as a monograph or chapter of a book. Authors of submitted papers are obligated not to submit their paper for publication elsewhere until an editorial decision is rendered on their submission. Further, authors of accepted papers are prohibited from publishing the results in other publications that appear before the paper is published in the Journal unless they receive approval for doing so from the Editor-In-Chief.
IJISAE open access articles are licensed under a Creative Commons Attribution-ShareAlike 4.0 International License. This license lets the audience to give appropriate credit, provide a link to the license, and indicate if changes were made and if they remix, transform, or build upon the material, they must distribute contributions under the same license as the original.