Big Data Predictive Analysis for Type-2 Diabetes Based Heart Disease Using Feature Extraction and Classification by Machine Learning Architectures
Keywords:
Machine Learning, big data, predictive analysis, type 2 diabetes, dimensionality reductionAbstract
Machine learning (ML), a branch of AI, enables computers to learn without being explicitly programmed. ML is widely applied in the healthcare industry to forecast a variety of chronic conditions. For improved clinical paths to prevent complications and postpone the onset of diabetes, earlier diabetes prediction is essential. This research propose novel technique in type 2 diabetes based heart disease detection in big data predictive analysis using machine learning method. Input data has been collected as type 2 diabetes and processed for noise removal and dimensionality reduction. Then the processed data features has been extracted for detecting the abnormality of type 2 diabetes using regression model based linear discriminant analysis. The extracted features shows the abnormal type 2 diabetes and for predicting heart disease by classifying the extracted data using VGG-16 Net_gradient NN. Experimental analysis has been carried out in terms of accuracy, precision, recall, F-1 score, RMSE and MAP for various diabetes dataset. Proposed technique attained accuracy of 96%, precision of 67%, recall of 79%, F-1 score of 63%, RMSE of 66% and MAP of 68%.
Downloads
References
Ghojogh, B., Karray, F., & Crowley, M. (2019). Fisher and kernel Fisher discriminant analysis: Tutorial. arXiv preprint arXiv:1906.09436.
Wu, W., Wang, J., Cheng, M., & Li, Z. (2011). Convergence analysis of online gradient method for BP neural networks. Neural Networks, 24(1), 91-98.
Hossain, M. E., Uddin, S., & Khan, A. (2021). Network analytics and machine learning for predictive risk modelling of cardiovascular disease in patients with type 2 diabetes. Expert Systems with Applications, 164, 113918.
Nicolucci, A., Romeo, L., Bernardini, M., Vespasiani, M., Rossi, M. C., Petrelli, M., ... & Vespasiani, G. (2022). Prediction of complications of type 2 Diabetes: A Machine learning approach. Diabetes Research and Clinical Practice, 190, 110013.
Krishnamoorthi, R., Joshi, S., Almarzouki, H. Z., Shukla, P. K., Rizwan, A., Kalpana, C., & Tiwari, B. (2022). A novel diabetes healthcare disease prediction framework using machine learning techniques. Journal of Healthcare Engineering, 2022.
Abdalrada, A. S., Abawajy, J., Al-Quraishi, T., & Islam, S. M. S. (2022). Machine learning models for prediction of co-occurrence of diabetes and cardiovascular diseases: a retrospective cohort study. Journal of Diabetes & Metabolic Disorders, 1-11.
Hosseini Sarkhosh, S. M., Esteghamati, A., Hemmatabadi, M., & Daraei, M. (2022). Predicting diabetic nephropathy in type 2 diabetic patients using machine learning algorithms. Journal of Diabetes & Metabolic Disorders, 1-9.
Sampathkumar, A., Tesfayohani, M., Shandilya, S. K., Goyal, S. B., Shaukat Jamal, S., Shukla, P. K., ... & Albeedan, M. (2022). Internet of Medical Things (IoMT) and Reflective Belief Design-Based Big Data Analytics with Convolution Neural Network-Metaheuristic Optimization Procedure (CNN-MOP). Computational Intelligence and Neuroscience, 2022.
Kour, H., Sabharwal, M., Suvanov, S., & Anand, D. (2021). An assessment of type-2 diabetes risk prediction using machine learning techniques. In Proceedings of International Conference on Big Data, Machine Learning and their Applications (pp. 113-122). Springer, Singapore.
Hassan, M. M., Billah, M. A. M., Rahman, M. M., Zaman, S., Shakil, M. M. H., & Angon, J. H. (2021, July). Early predictive analytics in healthcare for diabetes prediction using machine learning approach. In 2021 12th International Conference on Computing Communication and Networking Technologies (ICCCNT) (pp. 01-05). IEEE.
Sharma, A., & Mishra, P. K. (2022). Performance analysis of machine learning based optimized feature selection approaches for breast cancer diagnosis. International Journal of Information Technology, 14(4), 1949-1960.
Arumugam, K., Naved, M., Shinde, P. P., Leiva-Chauca, O., Huaman-Osorio, A., & Gonzales-Yanac, T. (2021). Multiple disease prediction using Machine learning algorithms. Materials Today: Proceedings.
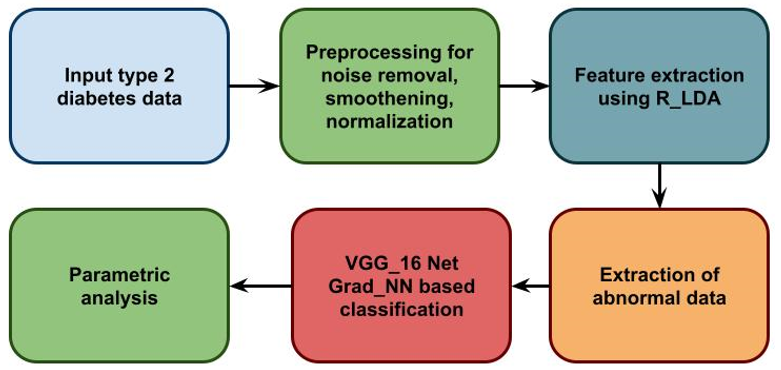
Downloads
Published
How to Cite
Issue
Section
License

This work is licensed under a Creative Commons Attribution-ShareAlike 4.0 International License.
All papers should be submitted electronically. All submitted manuscripts must be original work that is not under submission at another journal or under consideration for publication in another form, such as a monograph or chapter of a book. Authors of submitted papers are obligated not to submit their paper for publication elsewhere until an editorial decision is rendered on their submission. Further, authors of accepted papers are prohibited from publishing the results in other publications that appear before the paper is published in the Journal unless they receive approval for doing so from the Editor-In-Chief.
IJISAE open access articles are licensed under a Creative Commons Attribution-ShareAlike 4.0 International License. This license lets the audience to give appropriate credit, provide a link to the license, and indicate if changes were made and if they remix, transform, or build upon the material, they must distribute contributions under the same license as the original.