Novel Technique in Content Based Image Retrieval using Classification by Deep Learning in Artificial Intelligence
Keywords:
Content-based image retrieval, classification, DL, noise removal, ranking matrix, distance calculationAbstract
A popular technique for retrieving images from sizable and unlabeled image collections is content-based image retrieval (CBIR). One of the subcategories of the soft computing phenomenon known as "deep learning" allows for the retrieval of data from millions of separated images. This research propose novel technique in comprehensive description for content based image retrieval by classification utilizing DL techniques. Here input image has been collected and processed for noise removal. The processed image has been extracted and classified using FFCNN. Based on extracted features by calculating the similarity index of the images based on ranking matrix and distance calculation the image has been retrieved. The experimental analysis has been carried out in terms of accuracy, precision, recall, RMSE. the proposed technique attained accuracy of 95%, precision of 79%, recall of 68% and RMSE of 61%.
Downloads
References
Bartłomiej Mellera, Kamil Matula, “Image classification with Feed-Forward Neural Networks”, Symposium for Young Scientists in Technology, Engineering and Mathematics, Online, May 20 2020.
Garg, M., & Dhiman, G. (2021). A novel content-based image retrieval approach for classification using GLCM features and texture fused LBP variants. Neural Computing and Applications, 33(4), 1311-1328.
Saleem, S., Amin, J., Sharif, M., Anjum, M. A., Iqbal, M., & Wang, S. H. (2022). A deep network designed for segmentation and classification of leukemia using fusion of the transfer learning models. Complex & Intelligent Systems, 8(4), 3105-3120.
Jaiswal, A., Gianchandani, N., Singh, D., Kumar, V., & Kaur, M. (2021). Classification of the COVID-19 infected patients using DenseNet201 based deep transfer learning. Journal of Biomolecular Structure and Dynamics, 39(15), 5682-5689.
Yu, H., Yang, L. T., Zhang, Q., Armstrong, D., & Deen, M. J. (2021). Convolutional neural networks for medical image analysis: state-of-the-art, comparisons, improvement and perspectives. Neurocomputing, 444, 92-110.
Shankar, K., & Perumal, E. (2021). A novel hand-crafted with deep learning features based fusion model for COVID-19 diagnosis and classification using chest X-ray images. Complex & Intelligent Systems, 7(3), 1277-1293.
Bansal, M., Kumar, M., & Kumar, M. (2021). 2D object recognition: a comparative analysis of SIFT, SURF and ORB feature descriptors. Multimedia Tools and Applications, 80(12), 18839-18857.
El Asnaoui, K., Chawki, Y., & Idri, A. (2021). Automated methods for detection and classification pneumonia based on x-ray images using deep learning. In Artificial intelligence and blockchain for future cybersecurity applications (pp. 257-284). Springer, Cham.
Wang, Z., Zhao, W., Du, W., Li, N., & Wang, J. (2021). Data-driven fault diagnosis method based on the conversion of erosion operation signals into images and convolutional neural network. Process Safety and Environmental Protection, 149, 591-601.
Ibrahim, A. U., Ozsoz, M., Serte, S., Al-Turjman, F., & Yakoi, P. S. (2021). Pneumonia classification using deep learning from chest X-ray images during COVID-19. Cognitive Computation, 1-13.
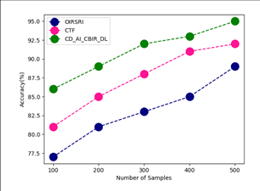
Downloads
Published
How to Cite
Issue
Section
License
Copyright (c) 2022 Nilanjana Saha , P. Thiruvannamalai Sivasankar , Rahama Salman , Badria Sulaiman Alfurhood , Aishwary Awasthi , Sukhwant Singh Bindra

This work is licensed under a Creative Commons Attribution-ShareAlike 4.0 International License.
All papers should be submitted electronically. All submitted manuscripts must be original work that is not under submission at another journal or under consideration for publication in another form, such as a monograph or chapter of a book. Authors of submitted papers are obligated not to submit their paper for publication elsewhere until an editorial decision is rendered on their submission. Further, authors of accepted papers are prohibited from publishing the results in other publications that appear before the paper is published in the Journal unless they receive approval for doing so from the Editor-In-Chief.
IJISAE open access articles are licensed under a Creative Commons Attribution-ShareAlike 4.0 International License. This license lets the audience to give appropriate credit, provide a link to the license, and indicate if changes were made and if they remix, transform, or build upon the material, they must distribute contributions under the same license as the original.