Deep learning-Assisted Glaucoma Diagnosis and Model Design
Keywords:
Glaucoma, GON, CNN, enormous, TVGH, classifier, diagnosis, characteristicAbstract
Purpose:
To evaluate the key factors used for classification and methods to improve the glaucomatous optic neuropathy (GON) in the form of systematic study along with fundus pictures as constraint sample be detected.
Methods:
Retrospectively, 940 fundus photographs from Eye hospital were gathered. The clinical and demographic details were noted together with the constitutional and functioning metrics of the pictures with GON. Convectional neural networks (CNNs) were built using transfer learning based on VGGNet to recognize GON. When CNN classifier had least rating the concluding classification will be construct with the cup-to-disc would be created by a extractor named support vector Machine. Scores were used to prevent missing instances with advanced GON. The TVGH dataset was used to create the CNN classifier, which was subsequently improved by fusing the training pictures from the TVGH and Drishti-GS datasets. CNN's primary characteristics for classification were identified using the class activation map (CAM). Classifier’s performance were evaluated with Area under receiver operating characteristic curve (AUC) and the diagnostic accuracy will enable to ccomparing the ensemble model.
Results:
While the comparison to ensemble model's accuracy rate of 92.8 percent, the CNN classifier's accuracy, sensitivity, and specificity were 95.0 percent, 95.7 percent, and 94.2 percent, respectively, in 187 EH test images, respectively, in 187 EH test pictures, and the AUC was 0.992 as opposed to the ensemble model's accuracy rate of 92.8 percent. The accuracy of the CNN, the fine-tuned CNN, and the ensemble model for the Drishti-GS test pictures was 33.3 percent, 80.3 percent [33], and 80.3 percent, respectively. Neither moderately nor severely ill photos were incorrectly classified using the CNN classifier. Class-discriminate areas discovered by CAM co-localized with established GON traits.
Conclusions:
When enormous image datasets are not readily accessible for developing evident learning model towards glaucoma diagnosis, The combo model or a personalized CNN classifier might be as practicable designs.
Downloads
References
S. S S, J. Surendiran, N. Yuvaraj, M. Ramkumar, C. N. Ravi and R. G. Vidhya, "Classification of Diabetes using Multilayer Perceptron," 2022 IEEE International Conference on Distributed Computing and Electrical Circuits and Electronics (ICDCECE), 2022, pp. 1-5, doi: 10.1109/ICDCECE53908.2022.9793085.
Ernest PJ, Schouten JS, Beckers HJ, Hendrikse F, Prins MH, Webers CA. An evidence-based review of prognostic factors for glaucomatous visual field progression. Ophthalmology. 2013; 120(3):512–19. https://doi.org/10.1016/j.ophtha.2012.09.005 PMID: 23211636
Chen PP. Blindness in patients with treated open-angle glaucoma. Ophthalmology. 2003; 110(4):726– 733. https://doi.org/10.1016/S0161-6420(02)01974-7 PMID: 12689894
Surendiran, J., Theetchenya, S., Benson Mansingh, P. M., Sekar, G., Dhipa, M., Yuvaraj, N., & Alene, A. (2022). Segmentation of Optic Disc and Cup Using Modified Recurrent Neural Network. BioMed Research International, 2022.
Mitchell P, Smith W, Attebo K, Healey PR. Prevalence of open-angle glaucoma in Australia. The Blue Mountains Eye Study. Ophthalmology 1996; 103(10):1661–9. https://doi.org/10.1016/s0161-6420(96) 30449-1 PMID: 8874440
Burr JM, Mowatt G, Herna´ndez R, Siddiqui MA, Cook J, Lourenco T, et al. The clinical effectiveness and cost-effectiveness of screening for open angle glaucoma: a systematic review and economic evalu- ation. Health Technol Assess. 2007; 11(41): iii-iv, ix-x, 1–190. https://doi.org/10.3310/hta11410 PMID: 17927922
Thomas SM, Jeyaraman MM, Hodge WG, Hutnik C, Costella J, Malvankar-Mehta MS. The effective- ness of teleglaucoma versus in-patient examination for glaucoma screening: a systematic review and meta-analysis. PLoS One. 2014; 9(12):e113779. https://doi.org/10.1371/journal.pone.0113779 PMID: 25479593
Hertzog LH, Albrecht KG, LaBree L, Lee PP. Glaucoma care and conformance with preferred practice patterns. Examination of the private, community-based ophthalmologist. Ophthalmology. 1996; 103 (7):1009–13. https://doi.org/10.1016/s0161-6420(96)30573-3 PMID: 8684788
D.Saravanan, J.Surendiran,”A new framework for video data retrieval using hierarchical clustering technique”, International Journal of Engineering and Advanced Technology (IJEAT) ISSN: 2249 – 8958, Volume-8, Issue-6S3, September 2019.
Badalà F, Nouri-Mahdavi K, Raoof DA, Leeprechanon N, Law SK, Caprioli J. Optic disk and nerve fiber layer imaging to detect glaucoma. Am J Ophthalmol. 2007; 144(5):724–32. https://doi.org/10.1016/j. ajo.2007.07.010 PMID: 17868631
O’Neill EC, Gurria LU, Pandav SS, Kong YX, Brennan JF, Xie J, et al. Glaucomatous optic neuropathy evaluation project: factors associated with underestimation of glaucoma likelihood. JAMA Ophthalmol. 2014; 132(5):560–6. https://doi.org/10.1001/jamaophthalmol.2014.96 PMID: 24699817
J.Surendiran, Dr.S.V.Saravanan, F.Elizabeth Catherine,”GLAUCOMA DETECTION USING FUZZY C- MEAN (FCM)”, IJPT| Sep-2016 | Vol. 8 | Issue No.3 | 16149-16163
Garway-Heath DF, Ruben ST, Viswanathan A, Hitchings RA. Vertical cup/disc ratio in relation to optic disc size: its value in the assessment of the glaucoma suspect. Br J Ophthalmol. 1998; 82(10):1118– 24. https://doi.org/10.1136/bjo.82.10.1118 PMID: 9924296.
Gu J, Wang Z, Kuen J, Ma L, Shahroudy A, Shuai B, et al. Recent advances in convolutional neural net- works. ArXiv: 1512.07108v6. 2017 [cited 2017 Oct 19]. Available from: https://arxiv.org/abs/1512.07108.
J.Surendiran, Dr.S.V.Saravanan, K.KManivannan,”Detection of Glaucoma Based on Color Moments and SVM Classifier Using K Mean Clustering”, IJPT| Sep-2016 | Vol. 8 | Issue No.3 | 16139-16148.
Li Z, He Y, Keel S, Meng W, Chang RT, He M. Efficacy of a deep learning system for detecting glauco- matous optic neuropathy based on color fundus photographs. Ophthalmology. 2018; 125(8):1199– 1206. https://doi.org/10.1016/j.ophtha.2018.01.023 PMID: 29506863
Phene S, Carter Dunn R, Hammel N, Liu Y, Krause J, Kitade N, et al. Deep learning to assess glaucoma risk and associated features in fundus images. ArXiv: 1812.08911v1. 2018 [cited 2018 Dec 21]. Avail- able from: https://arxiv.org/abs/1812.08911.
Jonas JB, Aung T, Bourne RR, Bron AM, Ritch R, Panda-Jonas S. Glaucoma. Lancet. 2017; 390 (10108):2183–2193. https://doi.org/10.1016/S0140-6736(17)31469-1 PMID: 28577860
Gulshan V, Peng L, Coram M, Stumpe MC, Wu D, Narayanaswamy A, et al. Development and valida- tion of a deep learning algorithm for detection of diabetic retinopathy in retinal fundus photographs. JAMA. 2016; 316(22):2402–2410. https://doi.org/10.1001/jama.2016.17216 PMID: 27898976
Krause J, Gulshan V, Rahimy E, Karth P, Widner K, Corrado GS, et al. Grader variability and the impor- tance of reference standards for evaluating machine learning models for diabetic retinopathy. Ophthal- mology. 2018; 125(8):1264–1272. https://doi.org/10.1016/j.ophtha.2018.01.034 PMID: 29548646
Sivaswamy J, Krishnadas S, Chakravarty A, Joshi GD, Ujjwal, Syed TA. A comprehensive retinal image dataset for the assessment of glaucoma from the optic nerve head analysis. JSM Biomed Imaging Data Pap. 2015, 2(1):1004.
Springenberg JT, Dosovitskiy A, Brox T, Riedmiller M. Striving for simplicity: the all convolutional net. ArXiv: 1412.6806. 2014 [cited 2014 Dec 21]. Available from: https://arxiv.org/abs/1412.6806.
Simonyan K, Zisserman A. Very Deep Convolutional networks for large-scale image recognition. ArXiv: 1409.1556. 2014 [cited 2014 Sep 4]. Available from: https://arxiv.org/abs/1409.1556.
Yosinski J, Clune J, Bengio Y, Lipson H. How transferable are features in deep neural networks? ArXiv: 1411.1792v1. 2014 [cited 2014 Nov 6]. Available from: https://arxiv.org/abs/1411.1792v1
Orlando JI, Prokofyeva E, Fresno MD, Blaschko MB. Convolutional neural network transfer for auto- mated glaucoma identification. Proceedings of the 12th International Symposium on Medical Informa- tion Processing and Analysis. 2016 Dec 5–7; Tandil Argentina; SPIE; 2016. Vol. 10160; pp. 1–10. https://doi.org/10.1117/12.2255740.
Zhou B, Khosla A, Lapedriza A, Oliva A, Torralba A. Learning deep features for discriminative localiza- tion. ArXiv: 1512.04150. 2015 [cited 2015 Dec 14]. Available from: https://arxiv.org/abs/1512.04150.
Lin M, Chen Q, Yan S. Network in network. ArXiv: 1312.4400. 2013 [cited 2013 Dec 16]. Available from: https://arxiv.org/abs/1312.4400.
Ayub J, Ahmad J, Muhammad J, Aziz L, Ayub S, Akram U, et al. Glaucoma detection through optic disc and cup segmentation using K-mean clustering. Proceedings of 2016 International Conference on Computing, Electronic and Electrical Engineering (ICE Cube).2016 April 11–12; Quetta Pakistan; IEEE; 2016. https://doi.org/10.1109/ICECUBE.2016.7495212.
Pedregosa F, Varoquaux G, Gramfort A, Michel V, Thirion B, Grisel O, et al. Scikit-learn: Machine Learning in Python. JMLR. 2011; 12:825–2830. http://www.jmlr.org/papers/volume12/pedregosa11a/ pedregosa11a.pdf
Liu H, Li L, Wormstone IM, Qiao C, Zhang C, Liu P, et al. Development and validation of a deep learning system to detect glaucomatous optic neuropathy using fundus photographs. JAMA Ophthalmol. 2019; 137(12):1353–1360. https://doi.org/ 10.1001/jamaophthalmol.2019.3501
Diaz-Pinto A, Morales S, Naranjo V, Kohler T, Mossi JM, Navea A. CNNs for automatic glaucoma assessment using fundus images: an extensive validation. Biomed Eng Online. 2019; 18(1):29. https:// doi.org/10.1186/s12938-019-0649-y PMID: 30894178
Ko, Yu-Chieh, et al. “Deep Learning Assisted Detection of Glaucomatous Optic Neuropathy and Potential Designs for a Generalizable Model.” Deep Learning Assisted Detection of Glaucomatous Optic Neuropathy and Potential Designs for a Generalizable Model | PLOS ONE, journals.plos.org, 14 May 2020, https://journals.plos.org/plosone/article?id=10.1371/journal.pone.0233079.
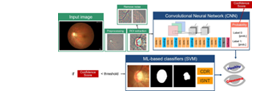
Downloads
Published
How to Cite
Issue
Section
License
Copyright (c) 2023 J. Surendiran, M. Meena

This work is licensed under a Creative Commons Attribution-ShareAlike 4.0 International License.
All papers should be submitted electronically. All submitted manuscripts must be original work that is not under submission at another journal or under consideration for publication in another form, such as a monograph or chapter of a book. Authors of submitted papers are obligated not to submit their paper for publication elsewhere until an editorial decision is rendered on their submission. Further, authors of accepted papers are prohibited from publishing the results in other publications that appear before the paper is published in the Journal unless they receive approval for doing so from the Editor-In-Chief.
IJISAE open access articles are licensed under a Creative Commons Attribution-ShareAlike 4.0 International License. This license lets the audience to give appropriate credit, provide a link to the license, and indicate if changes were made and if they remix, transform, or build upon the material, they must distribute contributions under the same license as the original.