Feature-Based Machine Intelligent Mapping of Cancer Beating Molecules
Keywords:
Anti-cancer, Prediction Model, Stacked Ensemble Neural networks, Machine learning, OncologyAbstract
Despite the fact that cancer has been treated using a variety of surgical and therapeutic techniques such as chemotherapy, target therapy, immunotherapy, and hormone therapy, understanding cancer cell biology, and cancer metastatic mechanisms are critical. According to recent studies, up to 30–40% of cancers can be avoided simply by changing one's diet and lifestyle. Diet and nutritional factors are vital in the prevention of a variety of diseases, and they have a substantial impact on patients' disease outcomes both during and after therapy. In this,the mechanical response of food particles plays a major role to modulate muscle activity and the dielectric properties of lossy materials are affected by frequency, temperature, and material composition. Hence a unique, network machine learning approach is used for finding mechanical and electrical features that are proposed, that intern used for cancer-fighting. The groundwork models that planned to employ stacked ensemble learning methods. ElasticNet, pairwise support vector regression, Kernelized Bayesian Multitask Learning,and Neural networks use in this prediction. In terms of sensitivity and activity, the two signatures indicated cell lines and drugs. A sensitivity signature shows the changes in gene expression that cause cell death in a particular cell line. During the action, mechanical, electrical properties and expressions are taken to suppress cancer in the sensitive line. To improve the fitness analysis in the drug response,the cost function is calculated in this research.
Downloads
References
National Cancer Institute (NCI): Cancer Stat Facts: Cancer of Any Site, (2019). https://seer.cancer.gov/statfacts/html/all.html.
American Cancer Society (ACS), Key Statistics for Lung Cancer, (2020). https ://www.cancer.org/cancer/lung-cancer/about/key-statistics.html.
Wiesweg M.,(2019). Machine learning-based predictors for immune checkpoint inhibitor therapy of non-small-cell lung cancer, Ann Oncol:30(4):655e7.
Heo, J. (2019). Machine learning-based model for prediction of outcomes in acute stroke, Stroke:50(5):1263e5.
Gunther,M.,Juchum, M.,Kelter,G.,Fiebig, H., & Laufer, S.(2016). Lung cancer: Egfr inhibitors with low nanomolar activity against a therapy resistant l858r/t790m/c797s mutant AngewandteChemie, International Edition, vol. 55, no. 36, pp. 10890–10894.
Qureshi,R., Nawaz,M., Ghosh, A., &Yan, H.(2019). Parametric models for understanding atomic trajectories in different domains of lung cancer-causing protein, IEEE Access, vol. 7, pp. 67551–67563.
Ikemura,S.,Yasuda.H., Matsumoto,S.,Kamada,M.,Hamamoto, J., Masuzawa,K.,Kobayashi,K., Manabe,T.,D., Arai, I., &Nakachi.(2019). Molecular dynamics simulation-guided drug sensitivity prediction for lung cancer with rare egfr mutations.Proceedings of the National Academy of Sciences, vol. 116, no. 20, pp. 10025–10030.
Lee, G.,Y., H., &Lim, C., T.(2007). Biomechanics approaches to studying human diseases.Trends Biotechnol, 25: 111–118.
Lim, C.,T., Zhou, E., H., Li, A.,Vedula, S., R., K., & Fu HX.(2006). Experimental techniques for single-cell and single molecule biomechanics, Mater Sci Eng C, 26: 1278–1288.
Garnett, M., J.(2012). Systematic identification of genomic markers of drug sensitivity in cancer cells.Nature, 483, 570–575.
Barretina, J., (2012). The cancer cell line encyclopedia enables predictive modeling of anticancer drug sensitivity Nature, 483, 603–607.
James Surowiecki.(2014). The Wisdom of Crowds.
Ding, H., Takigawa, I.&Mamitsuka,H.(2013). Similarity-based machine learning methods for predicting drug-target interactions: a brief review. Brief Bioinform, 5(5):734-47.
Lin Zhang, Xing Chen, Na-Na Guan, Hui Liu &Jian-QiangLi.(2018). A Hybrid Interpolation Weighted Collaborative Filtering Method for Anti-cancer Drug Response Prediction, vol no.9, article 1017.
Chuanying Liu, Dong Wei, Ju Xiang, Fuquan Ren, Li Huang, Jidong Lang, Geng Tian, Yushuang Li &JialiangYang.(2020).An Improved Anticancer Drug-Response Prediction Based on an Ensemble Method Integrating Matrix Completion and Ridge Regression, vol no.21.
Yitan Zhu, Thomas Brettin, Yvonne.A.,Evrard, Alexander Partin, Fangfang Xia, Maulik Shukla, Hyun seungYoo, James, H.,Doroshow, Rick, L.,&Stevens.(2020), Ensemble transfer learning for the prediction of anticancer drug response, vol.10,18040.
Chen, R., Liu, X., &Jin,S.(2018). Machine learning for drug-target interaction prediction. Molecules,23(9):2208.
GuoshengLianga, WenguoFanb, Hui Luoa&Xiao Zhua.(2020). The emerging roles of artificial intelligence in cancer drug development and precision therapy, vol.128,110255.
Sachdev, K., &Gupta, M., K. (2019). A comprehensive review of feature-based methods for drug target interactionprediction.J Biomed Inform,93:103159.
Raghvi Bhardwaj&Nishtha Hooda.(2009). Prediction of Pathological Complete Response after Neoadjuvant Chemotherapy for breast cancer using ensemble machine learning, 2352-9148.
Aman Sharma&Rinkle Rani.(2019). Drug sensitivity prediction framework using ensemble and multitask learning.
Mehmet Tana, Ozan FıratÖzgüla, BatuhanBardaka, IşıksuEkşioğlua&SunaSabuncuoğlu.(2018). Drug response prediction by ensemble learning and drug-induced gene expression signatures. Grand No.115E274.
Fangfang Xia et.al: “A cross-study analysis of drug response prediction in cancer cell lines.” (2021):1-14.
Senousy,M.B.,El-Deeb, H.M.,Badran, K., &Ibrahim Ali Al-Khlil.(2012).Ensemble Learning Based on Ranking Attribute Value (ELBRAV) for imbalanced Biomedical Data Classification. ISSN-1110-2586: vol. 36 No. 1.
Aman Sharma, Rinkle Rani.(2018). Kernelized similarity-based regularized matrix factorization framework for prediction anti-cancer drug responses.KSRMF: vol.1779-1790.
EmdadiAkram, EslahchiChangiz. Dsplmf. (2020). A method for cancer drug sensitivity prediction using a novel regularization approach in logistic matrix factorization.Frontiers in Genetics, 11:75. pmid:32174963.
SuphavilaiChayaporn, Bertrand Denis&Nagarajan Niranjan(2018).Predicting cancer drug response using a recommender system Bioinformatics., 34(22):3907–3914, pmid:29868820.
Zhang Naiqian, Wang Haiyun, Fang Yun, Wang Jun, Zheng Xiaoqi,&Shirley Liu X.(2015). Predicting anticancer drug responses using a dual-layer integrated cell line-drug network model, PLoSComput Biol:11(9): e1004498: pmid:26418249.
Lakshman Kumar pappala, Dr. SelviRajendran.P.(2021). A Novel Music Genre Classification using Convolution Neural Networks: IEEE conference on communication and electronics system,8-10.
Dr. Selvi Rajendran,P.,&Dr.Anithaashri.T.P. CNN-based Framework for identifying the Indian Currency Denomination for Physically Challenged People.: IOP Conference Series: Materials Science and Engineering for the publication.
Dr. SelviRajendran,P.,&Dr. Angilna Geetha.(2021). Optimization of hospital Bed Occupancy in Hospital Using a Double Deep Q Network.International Conference on Intelligent Communication Technologies and Virtual Mobile Network : (ICICV-2021):4-6.
Chen, Joy IongZong, Hengjinda, P.(2021). Early Prediction of Coronary Artery Disease (CAD) by Machine Learning Method-A Comparative Study.Journal of Artificial Intelligence 3, no. 01: 17-3
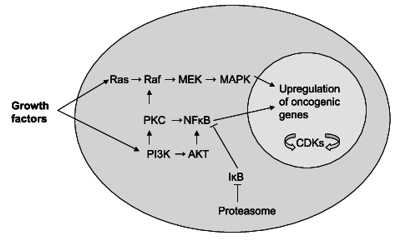
Downloads
Published
How to Cite
Issue
Section
License

This work is licensed under a Creative Commons Attribution-ShareAlike 4.0 International License.
All papers should be submitted electronically. All submitted manuscripts must be original work that is not under submission at another journal or under consideration for publication in another form, such as a monograph or chapter of a book. Authors of submitted papers are obligated not to submit their paper for publication elsewhere until an editorial decision is rendered on their submission. Further, authors of accepted papers are prohibited from publishing the results in other publications that appear before the paper is published in the Journal unless they receive approval for doing so from the Editor-In-Chief.
IJISAE open access articles are licensed under a Creative Commons Attribution-ShareAlike 4.0 International License. This license lets the audience to give appropriate credit, provide a link to the license, and indicate if changes were made and if they remix, transform, or build upon the material, they must distribute contributions under the same license as the original.