Machine Learning Based Techniques for Paddy Yield Prediction for the State of Andhra Pradesh
Keywords:
food security, crop yield, machine learning, RFR, correlationAbstract
Timely and accurate crop yield prediction serves as a pillar for the country’s food security and frames the strategic policies for the government. In this study, we endeavoured to assess the effectiveness of three various machine learning-based methods to predict paddy yield for the Indian state of Andhra Pradesh. The models were developed using historical yield data for the years 2001 to 2020 along with the long-term derived satellite variables evapotranspiration (ET), leaf area index (LAI), land surface temperature (LST), normalised difference vegetation index (NDVI), and rainfall (RF). Multiple linear regression (MLR), support vector regression (SVR), and random forest regression (RFR) models were three different machine learning models that were assessed for performance. A correlation was established between these variables and crop yield. The highly correlated features model was built and the features with the least correlation were discarded. The performance of all three models was found to be satisfactory. The RFR model was found to have higher accuracy with an R2 value of 0.61 and an RMSE of 0.55 t ha-1. Whereas MLR and SVR were found to have R2 0.51 and 0.59, RMSE 0.59 t ha-1, and 0.54 t ha-1. The results from the current study have shown the capability of machine learning algorithms with limited datasets.
Downloads
References
Arumugam, P., Chemura, A., Schauberger, B., & Gornott, C. (2021). Remote Sensing based yield estimation of rice (Oryza sativa L.) using gradient boosted regression in India. Remote Sensing, 13(12), 2379. https://doi.org/10.3390/rs13122379
Bender, S. F., & van der Heijden, M. G. (2015). Soil biota enhance agricultural sustainability by improving crop yield, nutrient uptake and reducing nitrogen leaching losses. Journal of Applied Ecology, 52(1), 228-239. https://doi.org/10.1111/1365-2664.12351
Bisong, E. Google Collaboratory (2019). Building Machine Learning and Deep Learning Models on Google Cloud Platform. Apress, Berkeley, CA, 59-64. https://doi.org/10.1007/978-1-4842-4470-8_7
Chaurasiya, G., Saxena, S., Tripathy, R., Chaudhary, K. N., & Ray, S. S. (2017). Semi physical approach for sugarcane yield modelling with remotely sensed inputs. Vayumandal, 43(1), 11-22.
Cheng, M., Jiao, X., Shi, L., Penuelas, J., Kumar, L., Nie, C., ... & Jin, X. (2022). High-resolution crop yield and water productivity dataset generated using random forest and remote sensing. Scientific Data, 9(1), 1-13. https://doi.org/10.1038/s41597-022-01761-0
Chlingaryan, A., Sukkarieh, S., & Whelan, B. (2018). Machine learning approaches for crop yield prediction and nitrogen status estimation in precision agriculture: A review. Computers and electronics in agriculture, 151, 61-69. https://doi.org/10.1016/j.compag.2018.05.012
Dang, C., Liu, Y., Yue, H., Qian, J., & Zhu, R. (2021). Autumn crop yield prediction using data-driven approaches: -support vector machines, random forest, and deep neural network methods. Canadian Journal of Remote Sensing, 47(2), 162-181. https://doi.org/10.1080/07038992.2020.1833186
Debnath, S., Mishra, A., Mailapalli, D. R., Raghuwanshi, N. S., & Sridhar, V. (2021). Assessment of rice yield gap under a changing climate in India. Journal of Water and Climate Change, 12(4), 1245-1267. https://doi.org/10.2166/wcc.2020.086
DES. (2021). Directorate of economics and statistics, ministry of agriculture and farmers welfare, government of India. https://eands.dacnet.nic.in/
Didan, K. (2021). MODIS/Terra Vegetation Indices 16-Day L3 Global 250m SIN Grid V061 [Data set]. NASA EOSDIS Land Processes DAAC. Accessed 2022-12-23 from https://doi.org/10.5067/MODIS/MOD13Q1.061
Doraiswamy, P. C., Moulin, S., Cook, P. W., & Stern, A. (2003). Crop yield assessment from remote sensing. Photogrammetric engineering & remote sensing, 69(6), 665-674. https://doi.org/10.14358/PERS.69.6.665
Dubey, S. K., Gavli, A. S., Yadav, S. K., Sehgal, S., & Ray, S. S. (2018). Remote sensing-based yield forecasting for sugarcane (Saccharum officinarum L.) crop in India. Journal of the Indian Society of Remote Sensing, 46(11), 1823-1833. https://doi.org/10.1007/s12524-018-0839-2
Elavarasan, D., & Vincent, P. M. (2021). A reinforced random forest model for enhanced crop yield prediction by integrating agrarian parameters. Journal of Ambient Intelligence and Humanized Computing, 12(11), 10009-10022. https://doi.org/10.1007/s12652-020-02752-y
Everingham, Y., Sexton, J., Skocaj, D., & Inman-Bamber, G. (2016). Accurate prediction of sugarcane yield using a random forest algorithm. Agronomy for sustainable development, 36(2), 1-9. https://doi.org/10.1007/s13593-016-0364-z
Fang, H., Liang, S., & Hoogenboom, G. (2011). Integration of MODIS LAI and vegetation index products with the CSM–CERES–Maize model for corn yield estimation. International Journal of Remote Sensing, 32(4), 1039-1065. https://doi.org/10.1080/01431160903505310
Frieler, K., Schauberger, B., Arneth, A., Balkovič, J., Chryssanthacopoulos, J., Deryng, D., ... & Levermann, A. (2017). Understanding the weather signal in national crop‐yield variability. Earth's future, 5(6), 605-616. https://doi.org/10.1002/2016EF000525
Funk, Chris, Pete Peterson, Martin Landsfeld, Diego Pedreros, James Verdin, Shraddhanand Shukla, Gregory Husak, James Rowland, Laura Harrison, Andrew Hoell & Joel Michaelsen (2015). "The climate hazards infrared precipitation with stations-a new environmental record for monitoring extremes". Scientific Data 2, 150066. doi:10.1038/sdata.2015.66.
Geetha, V., Punitha, A., Abarna, M., Akshaya, M., Illakiya, S., & Janani, A. P. (2020, July). An effective crop prediction using random forest algorithm. In 2020 International Conference on System, Computation, Automation and Networking (ICSCAN) (pp. 1-5). IEEE. https://doi.org/10.1109/ICSCAN49426.2020.9262311
Guhathakurta, P., Sanap, S., Menon, P., Prasad, A. K., Sangwan, N., & Advani, S. C. (2020). Observed rainfall variability and changes over Andhra Pradesh state. India Meteorological Department, Pune, India. https://imdpune.gov.in/Reports/rainfall%20variability%20page/reports/andhra_final.pdf
Gorelick, N., Hancher, M., Dixon, M., Ilyushchenko, S., Thau, D., & Moore, R. (2017). Google Earth Engine: Planetary-scale geospatial analysis for everyone. Remote sensing of Environment, 202, 18-27. https://doi.org/10.1016/j.rse.2017.06.031
Huang, J., Wang, X., Li, X., Tian, H., & Pan, Z. (2013). Remotely sensed rice yield prediction using multi-temporal NDVI data derived from NOAA's-AVHRR. Plos one, 8(8), e70816. https://doi.org/10.1371/journal.pone.0070816
Huang, J., Gómez-Dans, J. L., Huang, H., Ma, H., Wu, Q., Lewis, P. E., ... & Xie, X. (2019). Assimilation of remote sensing into crop growth models: Current status and perspectives. Agricultural and Forest Meteorology, 276, 107609. https://doi.org/10.1016/j.agrformet.2019.06.008
Jaikla, R., Auephanwiriyakul, S., & Jintrawet, A. (2008, May). Rice yield prediction using a support vector regression method. In 2008 5th International Conference on Electrical Engineering/Electronics, Computer, Telecommunications and Information Technology (Vol. 1, pp. 29-32). IEEE. https://doi.org/10.1109/ECTICON.2008.4600365
Jeong, J. H., Resop, J. P., Mueller, N. D., Fleisher, D. H., Yun, K., Butler, E. E., ... & Kim, S. H. (2016). Random forests for global and regional crop yield predictions. PloS one, 11(6), e0156571. https://doi.org/10.1371/journal.pone.0156571
Kumar, Y. J. N., Spandana, V., Vaishnavi, V. S., Neha, K., & Devi, V. G. R. R. (2020, June). Supervised machine learning approach for crop yield prediction in agriculture sector. In 2020 5th International Conference on Communication and Electronics Systems (ICCES) (pp. 736-741). IEEE. https://doi.org/10.1109/ICCES48766.2020.9137868
Li, Z., Wang, J., Xu, X., Zhao, C., Jin, X., Yang, G., & Feng, H. (2015). Assimilation of two variables derived from hyperspectral data into the DSSAT-CERES model for grain yield and quality estimation. Remote Sensing, 7(9), 12400-12418. https://doi.org/10.3390/rs70912400
Lobell, D. B., & Burke, M. B. (2008). Why are agricultural impacts of climate change so uncertain? The importance of temperature relative to precipitation. Environmental Research Letters, 3(3), 034007. https://doi.org/10.1088/1748-9326/3/3/034007
Manjunatha, A. V., & Kumar, P. (2015). Impact of national food security mission (NFSM) on input use, production, yield and income in Karnataka. Agricultural Development and Rural Transformation Centre Institute for Social and Economic Change, 79-81.
Nihar, A., Patel, N. R., & Danodia, A. (2022). Machine-Learning-Based Regional Yield Forecasting for Sugarcane Crop in Uttar Pradesh, India. Journal of the Indian Society of Remote Sensing, 1-12. https://doi.org/10.1007/s12524-022-01549-0
Patel, N. R., Mohammed, A. J., & Rakhesh, D. (2006). Modelling of Wheat Yields Using Multi‐Temporal Terra/MODIS Satellite Data. Geocarto International, 21(1), 43-50. https://doi.org/10.1080/10106040608542373
Prasad, N. R., Patel, N. R., & Danodia, A. (2021). Crop yield prediction in cotton for regional level using random forest approach. Spatial Information Research, 29(2), 195-206. https://doi.org/10.1007/s41324-020-00346-6
Prasad, N. R., Patel, N. R., & Danodia, A. (2021). Cotton Yield Estimation Using Phenological Metrics Derived from Long-Term MODIS Data. Journal of the Indian Society of Remote Sensing, 49(11), 2597-2610. https://doi.org/10.1007/s12524-021-01414-6
Prasad, N. R., Patel, N. R., Danodia, A., & Manjunath, K. R. (2022). Comparative performance of semi-empirical based remote sensing and crop simulation model for cotton yield prediction. Modelling Earth Systems and Environment, 8(2), 1733-1747. https://doi.org/10.1007/s40808-021-01180-x
Ranjan, A. K., & Parida, B. R. (2019). Paddy acreage mapping and yield prediction using sentinel-based optical and SAR data in Sahibganj district, Jharkhand (India). Spatial Information Research, 27(4), 399-410. https://doi.org/10.1007/s41324-019-00246-4
Rashid, M., Bari, B. S., Yusup, Y., Kamaruddin, M. A., & Khan, N. (2021). A comprehensive review of crop yield prediction using machine learning approaches with special emphasis on palm oil yield prediction. IEEE Access, 9, 63406-63439. https://doi.org/10.1109/ACCESS.2021.3075159
Ray, D. K., Gerber, J. S., MacDonald, G. K., & West, P. C. (2015). Climate variation explains a third of global crop yield variability. Nature communications, 6(1), 1-9. v https://doi.org/10.1038/ncomms6989
Sakamoto, T., Gitelson, A. A., & Arkebauer, T. J. (2013). MODIS-based corn grain yield estimation model incorporating crop phenology information. Remote Sensing of Environment, 131, 215-231. https://doi.org/10.1016/j.rse.2012.12.017
Sandhu, S. S., Dhillon, B. S., & Singh, H. (2021). Rice yield variability in Punjab: an overview of five decades. Paddy and Water Environment, 19(4), 673-681. https://doi.org/10.1007/s10333-021-00866-3
Sarma, A. A. L. N., Kumar, T. L., & Koteswararao, K. (2008). Development of an agroclimatic model for the estimation of rice yield. J. Ind. Geophys. Union, 12(2), 89-96.
Schumacher, D. L., Keune, J., Van Heerwaarden, C. C., Vilà-Guerau de Arellano, J., Teuling, A. J., & Miralles, D. G. (2019). Amplification of mega-heatwaves through heat torrents fuelled by upwind drought. Nature Geoscience, 12(9), 712-717. https://doi.org/10.1038/s41561-019-0431-6
Setiyono, T., Nelson, A., & Holecz, F. (2014). Remote sensing-based crop yield monitoring and forecasting. Crop monitoring for improved food security.
Supit, I., Van Diepen, C. A., De Wit, A. J. W., Wolf, J., Kabat, P., Baruth, B., & Ludwig, F. (2012). Assessing climate change effects on European crop yields using the Crop Growth Monitoring System and a weather generator. Agricultural and Forest Meteorology, 164, 96-111.
Tilman, D., Balzer, C., Hill, J., & Befort, B. L. (2011). Global food demand and the sustainable intensification of agriculture. Proceedings of the national academy of sciences, 108(50), 20260-20264. https://doi.org/10.1073/pnas.1116437108
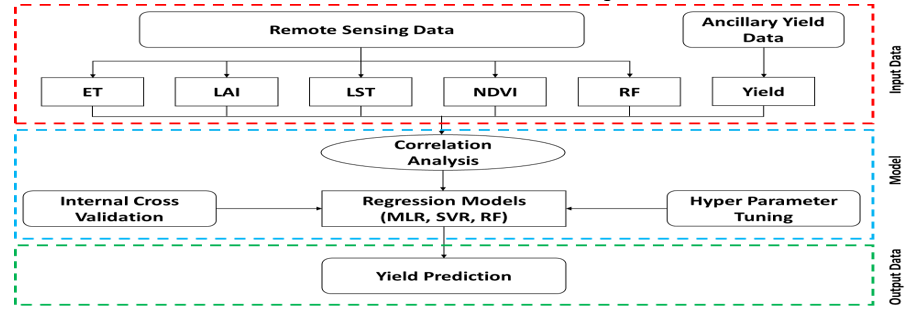
Downloads
Published
How to Cite
Issue
Section
License

This work is licensed under a Creative Commons Attribution-ShareAlike 4.0 International License.
All papers should be submitted electronically. All submitted manuscripts must be original work that is not under submission at another journal or under consideration for publication in another form, such as a monograph or chapter of a book. Authors of submitted papers are obligated not to submit their paper for publication elsewhere until an editorial decision is rendered on their submission. Further, authors of accepted papers are prohibited from publishing the results in other publications that appear before the paper is published in the Journal unless they receive approval for doing so from the Editor-In-Chief.
IJISAE open access articles are licensed under a Creative Commons Attribution-ShareAlike 4.0 International License. This license lets the audience to give appropriate credit, provide a link to the license, and indicate if changes were made and if they remix, transform, or build upon the material, they must distribute contributions under the same license as the original.