Understanding Customer Behaviour: A Comprehensive Survey of Segmentation and Classification Techniques in the Age of Big Data
Keywords:
Customer segmentation, Classification techniques, Marketing research, Machine learning, Behavioral segmentation, K-Means Clustering, Decision Trees, Support Vector Machines (SVM), Neural NetworksAbstract
The contemporary business environment necessitates an understanding of customer behavior and preferences to optimize marketing strategies and improve customer satisfaction. Customer segmentation and classification techniques have emerged as fundamental tools for organizations to differentiate their customers based on meaningful differences and create targeted marketing campaigns. This research paper provides a comprehensive survey of the latest customer segmentation and classification techniques used in marketing research. This paper examines traditional and modern approaches to customer segmentation and classification, encompassing demographic, geographic, psychographic, and behavioral segmentation. Furthermore, this paper investigates the latest advancements in customer classification techniques, such as machine learning, data mining, and artificial intelligence. Additionally, this paper discusses the challenges and limitations of these techniques and proposes future research directions. The findings of this research have significant implications for marketing practitioners and scholars interested in optimizing their marketing strategies and improving customer satisfaction.
Downloads
References
Alsayat, A. (2023). Customer decision-making analysis based on big social data using machine learning: a case study of hotels in Mecca. Neural Computing and Applications, 35(6), 4701–4722. https://doi.org/10.1007/s00521-022-07992-x
Ciupac-Ulici, M., Beju, D. G., Bresfelean, V. P., & Zanellato, G. (2023). Which Factors Contribute to the Global Expansion of M-Commerce? Electronics (Switzerland), 12(1). https://doi.org/10.3390/electronics12010197
Yoo, S., & Kim, J. (2023). Merchant Recommender System Using Credit Card Payment Data. Electronics (Switzerland), 12(4). https://doi.org/10.3390/electronics12040811
Jain, S., Obermeier, G., Auinger, A., Werth, D., & Kiss, G. (2023). Design Principles of a Mixed-Reality Shopping Assistant System in Omnichannel Retail. Applied Sciences (Switzerland), 13(3). https://doi.org/10.3390/app13031384
Sonia, Sharma, K., & Bajaj, M. (2023). DeepWalk Based Influence Maximization (DWIM): Influence Maximization Using Deep Learning. Intelligent Automation and Soft Computing, 35(1), 1087–1101. https://doi.org/10.32604/iasc.2023.026134
van Maasakkers, L., Fok, D., & Donkers, B. (2023). Next-basket prediction in a high-dimensional setting using gated recurrent units. Expert Systems with Applications, 212. https://doi.org/10.1016/j.eswa.2022.118795
Yıldız, E., Güngör Şen, C., & Işık, E. E. (2023). A Hyper-Personalized Product Recommendation System Focused on Customer Segmentation: An Application in the Fashion Retail Industry. Journal of Theoretical and Applied Electronic Commerce Research, 18(1), 571–596. https://doi.org/10.3390/jtaer18010029
Deenadayalan, D., & Kangaiammal, A. (2023). User Feature Similarity Supported Collaborative Filtering for Page Recommendation Using Hybrid Shuffled Frog Leaping Algorithm. International Journal of Intelligent Engineering and Systems, 16(1), 301–313. https://doi.org/10.22266/ijies2023.0228.27
Selvasheela, K., Abirami, A. M., & Askarunisa, A. K. (2023). Effective Customer Review Analysis Using Combined Capsule Networks with Matrix Factorization Filtering. Computer Systems Science and Engineering, 44(3), 2537–2552. https://doi.org/10.32604/csse.2023.029148
Dang Tran, H., Le, N., & Nguyen, V.-H. (2023). Customer Churn Prediction in the Banking Sector Using Machine Learning-Based Classification Models. Interdisciplinary Journal of Information, Knowledge, and Management, 18, 087–105. https://doi.org/10.28945/5086
Zhang, Z., & Wang, Y. (2023). Product Line Optimization for Car-Sharing Platforms in the Sustainable Transportation. Journal of Advanced Transportation, 2023. https://doi.org/10.1155/2023/9786689
Hai, T., Zhou, J., Lu, Y., Jawawi, D. N., Sinha, A., Bhatnagar, Y., & Anumbe, N. (2023). Posterior probability and collaborative filtering based Heterogeneous Recommendations model for user/item Application in use case of IoVT. Computers and Electrical Engineering, 105. https://doi.org/10.1016/j.compeleceng.2022.108532
Akanferi, A. A., Asampana, I., Matey, A. H., & Tanye, H. A. (2022). Adoption of Mobile Commerce and Mobile Payments in Ghana: an Examination of Factors Influencing Public Servants. Interdisciplinary Journal of Information, Knowledge, and Management, 17, 287–313. https://doi.org/10.28945/4981
Rudolf, S., Blömeke, S., Niemeyer, J. F., Lawrenz, S., Sharma, P., Hemminghaus, S., Mennenga, M., Schmidt, K., Rausch, A., Spengler, T. S., & Herrmann, C. (2022). Extending the Life Cycle of EEE—Findings from a Repair Study in Germany: Repair Challenges and Recommendations for Action. Sustainability (Switzerland), 14(5). https://doi.org/10.3390/su14052993
Kennedyd, S. I., Marjerison, R. K., Yu, Y., Zi, Q., Tang, X., & Yang, Z. (2022). E-Commerce Engagement: A Prerequisite for Economic Sustainability—An Empirical Examination of Influencing Factors. Sustainability (Switzerland), 14(8). https://doi.org/10.3390/su14084554
Salazar, M. B., Marín-Raventós, G., & Herrera, G. L. (2022). Designing Diagnosis Instruments to Determine e-Commerce Readiness for Micro and Small Enterprises in Rural Areas. CLEI Eletronic Journal (CLEIej), 25(2), 11. https://doi.org/10.19153/CLEIEJ.25.2.11
Nicolescu, L., & Tudorache, M. T. (2022). Human-Computer Interaction in Customer Service: The Experience with AI Chatbots—A Systematic Literature Review. Electronics (Switzerland), 11(10). https://doi.org/10.3390/electronics11101579
Shi, Y., Li, X., Zhougong, S., Li, X., & Wang, H. (2022). Precise Marketing Classification of Agricultural Products for E-Commerce Live Broadcast Platform Using Clustering. Mobile Information Systems, 2022. https://doi.org/10.1155/2022/1062938
Almahmood, R. J. K., & Tekerek, A. (2022). Issues and Solutions in Deep Learning-Enabled Recommendation Systems within the E-Commerce Field. Applied Sciences (Switzerland), 12(21). https://doi.org/10.3390/app122111256
Kaur, R., Singh, R., Gehlot, A., Priyadarshi, N., & Twala, B. (2022). Marketing Strategies 4.0: Recent Trends and Technologies in Marketing. Sustainability (Switzerland), 14(24). https://doi.org/10.3390/su142416356
Lingaitienė, O., Grybaitė, V., & Burinskienė, A. (2022). Core Elements Affecting Sharing Evidence from the European Union. Sustainability (Switzerland), 14(7). https://doi.org/10.3390/su14073845
Iwendi, C., Ibeke, E., Eggoni, H., Velagala, S., & Srivastava, G. (2022). Pointer-Based Item-to-Item Collaborative Filtering Recommendation System Using a Machine Learning Model. International Journal of Information Technology and Decision Making, 21(1), 463–484. https://doi.org/10.1142/S0219622021500619
Pinto, J. P., Veloso, C. M., Sousa, B. B., Valeri, M., Walter, C. E., & Lopes, E. (2022). Managerial Practices and (Post) Pandemic Consumption of Private Labels: Online and Offline Retail Perspective in a Portuguese Context. Sustainability (Switzerland), 14(17). https://doi.org/10.3390/su141710813
Kompan, M., Gaspar, P., MacIna, J., Cimerman, M., & Bielikova, M. (2022). Exploring Customer Price Preference and Product Profit Role in Recommender Systems. IEEE Intelligent Systems, 37(1), 89–98. https://doi.org/10.1109/MIS.2021.3092768
Sugiono, S., Sulistyorini, D. H., Sakinah, N., & Nugroho, W. S. (2022). Re-Designing of Railway Carriages To Increase Ergonomic and Accessible: a Case Studi of Indonesian Railway. Eastern-European Journal of Enterprise Technologies, 4(1–118), 13–22. https://doi.org/10.15587/1729-4061.2022.253415
Alamdari, P. M., Navimipour, N. J., Hosseinzadeh, M., Safaei, A. A., & Darwesh, A. (2022). Image-based Product Recommendation Method for E-commerce Applications Using Convolutional Neural Networks. Acta Informatica Pragensia, 11(1), 15–35. https://doi.org/10.18267/j.aip.167
Kutlimuratov, A., Abdusalomov, A. B., Oteniyazov, R., Mirzakhalilov, S., & Whangbo, T. K. (2022). Modeling and Applying Implicit Dormant Features for Recommendation via Clustering and Deep Factorization. Sensors, 22(21). https://doi.org/10.3390/s22218224
Zhao, X., & Keikhosrokiani, P. (2022). Sales prediction and product recommendation model through user behavior analytics. Computers, Materials and Continua, 70(2), 3855–3874. https://doi.org/10.32604/cmc.2022.019750
Lee, C., Han, D., Han, K., & Yi, M. (2022). Improving Graph-Based Movie Recommender System Using Cinematic Experience. Applied Sciences (Switzerland), 12(3). https://doi.org/10.3390/app12031493
Bataineh, A. Q. (2022). Analyzing the role of social media marketing in changing customer experience. International Journal of Data and Network Science, 6(3), 761–768. https://doi.org/10.5267/j.ijdns.2022.3.005
Indriasari, E., Prabowo, H., Gaol, F. L., & Purwandari, B. (2022). Intelligent Digital Banking Technology and Architecture: A Systematic Literature Review. International Journal of Interactive Mobile Technologies, 16(19), 98–117. https://doi.org/10.3991/ijim.v16i19.30993
Shema, A. (2022). Effects of increasing credit limit in digital microlending: A study of airtime lending in East Africa. Electronic Journal of Information Systems in Developing Countries, 88(3). https://doi.org/10.1002/isd2.12199
Gao, M., He, X., Chen, L., Liu, T., Zhang, J., & Zhou, A. (2022). Learning Vertex Representations for Bipartite Networks. IEEE Transactions on Knowledge and Data Engineering, 34(1), 379–393. https://doi.org/10.1109/TKDE.2020.2979980
Thaipisutikul, T. (2022). An Adaptive Temporal-Concept Drift Model for Sequential Recommendation. ECTI Transactions on Computer and Information Technology, 16(2), 221–235. https://doi.org/10.37936/ecticit.2022162.248019
Ahmad, J., Rehman, A., Rauf, H. T., Javed, K., Alkhayyal, M. A., & Alnuaim, A. A. (2022). Service Recommendations Using a Hybrid Approach in Knowledge Graph with Keyword Acceptance Criteria. Applied Sciences (Switzerland), 12(7). https://doi.org/10.3390/app12073544
Gao, S., & Meng, W. (2022). Development of a Personalized Recommendation System for E-Commerce Products for Distributed Storage Systems. Computational Intelligence and Neuroscience, 2022. https://doi.org/10.1155/2022/4752981
Yuliawati, A., Tohari, H., Mahendra, R., & Budi, I. (2022). On the Long Tail Products Recommendation using Tripartite Graph. International Journal of Advanced Computer Science and Applications, 13(1), 816–822. https://doi.org/10.14569/IJACSA.2022.0130195
Liu, L. (2022). E-Commerce Personalized Recommendation Based on Machine Learning Technology. Mobile Information Systems, 2022. https://doi.org/10.1155/2022/1761579
Shen, R. (2022). Prediction Method of User Behavior Label Based on the BP Neural Network. Scientific Programming, 2022. https://doi.org/10.1155/2022/7241956
Wei, Y. (2022). Advertising Image Design Skills of E-Commerce Products in the Context of the Internet of Things. Mobile Information Systems, 2022. https://doi.org/10.1155/2022/1022825
Jankeeparsad, R. W., & Tewari, D. (2022). Bitcoin: an Exploratory Study Investigating Adoption in South Africa. Interdisciplinary Journal of Information, Knowledge, and Management, 17, 191–214. https://doi.org/10.28945/4947
Hou, X. (2022). Core Technology Optimization of Intelligent Financial Technology Based on Collaborative Filtering Algorithm in Big Data Environment. Wireless Communications and Mobile Computing, 2022. https://doi.org/10.1155/2022/1693066
Li, L., Fu, L., & Zhang, W. (2022). Impact of Text Diversity on Review Helpfulness: A Topic Modeling Approach. Interdisciplinary Journal of Information, Knowledge, and Management, 17, 87–100. https://doi.org/10.28945/4922
Blagu, D., Szabo, D., Dragomir, D., Neamțu, C., & Popescu, D. (2022). Offering Carbon Smart Options through Product Development to Meet Customer Expectations. Sustainability (Switzerland), 14(16). https://doi.org/10.3390/su14169913
Al-Hazmi, N. M. (2022). E-marketing by Mobile Apps for Tourism Products and Its Impact on the Performance of Travel and Tourism Organizations. International Journal of Interactive Mobile Technologies, 16(12), 145–153. https://doi.org/10.3991/ijim.v16i12.30515
Beyari, H., & Garamoun, H. (2022). The Effect of Artificial Intelligence on End-User Online Purchasing Decisions: Toward an Integrated Conceptual Framework. Sustainability (Switzerland), 14(15). https://doi.org/10.3390/su14159637
Stitini, O., Kaloun, S., & Bencharef, O. (2022). An Improved Recommender System Solution to Mitigate the Over-Specialization Problem Using Genetic Algorithms. Electronics (Switzerland), 11(2). https://doi.org/10.3390/electronics11020242
Anggraeni, E. W., Handayati, Y., & Novani, S. (2022). Improving Local Food Systems through the Coordination of Agriculture Supply Chain Actors. Sustainability (Switzerland), 14(6). https://doi.org/10.3390/su14063281
An, Y., Meng, S., & Wu, H. (2022). Discover Customers’ Gender From Online Shopping Behavior. IEEE Access, 10, 13954–13965. https://doi.org/10.1109/ACCESS.2022.3147447
Bagwari, A., Sinha, A., Singh, N. K., Garg, N., & Kanti, J. (2022). CBIR-DSS: Business Decision Oriented Content-Based Recommendation Model for E-Commerce. Information (Switzerland), 13(10). https://doi.org/10.3390/info13100479
Sabitha, R., Vaishnavi, S., Karthik, S., & Bhavadharini, R. M. (2022). User interaction based recommender system using machine learning. Intelligent Automation and Soft Computing, 31(2), 1037–1049. https://doi.org/10.32604/iasc.2022.018985
Chopra, A. B., & Dixit, V. S. (2022). An adaptive RNN algorithm to detect shilling attacks for online products in hybrid recommender system. Journal of Intelligent Systems, 31(1), 1133–1149. https://doi.org/10.1515/jisys-2022-1023
Ali, N. M., Alshahrani, A., Alghamdi, A. M., & Novikov, B. (2022). SmartTips: Online Products Recommendations System Based on Analyzing Customers Reviews. Applied Sciences (Switzerland), 12(17). https://doi.org/10.3390/app12178823
Liu, W., & Ji, L. (2022). Multi-Feature Extraction Method of Power Customer’s Portrait based on Knowledge Map and Label Extraction. International Journal of Advanced Computer Science and Applications, 13(11), 129–138. https://doi.org/10.14569/IJACSA.2022.0131114
Chowdhury, M. N., Haque, H. M. Z., Tahmid, K. T., Salma, F. T. Z., & Ahmed, N. (2022). A Novel Approach for Product Recommendation Using Smartphone Sensor Data. International Journal of Interactive Mobile Technologies, 16(16), 190–204. https://doi.org/10.3991/ijim.v16i16.31617
Shajalal, M., Boden, A., & Stevens, G. (2022). Explainable product backorder prediction exploiting CNN: Introducing explainable models in businesses. Electronic Markets, 32(4), 2107–2122. https://doi.org/10.1007/s12525-022-00599-z
Almajali, D. A. (2022). Determinants of Online Behavior Among Jordanian Consumers: an Empirical Study of Opensooq. Interdisciplinary Journal of Information, Knowledge, and Management, 17, 339–359. https://doi.org/10.28945/4994
Jarman, D., Berry, J., Smith, R., Thung, F., & Lo, D. (2022). Legion: Massively Composing Rankers for Improved Bug Localization at Adobe. IEEE Transactions on Software Engineering, 48(8), 3010–3024. https://doi.org/10.1109/TSE.2021.3075215
Mohamed, S., Sethom, K., Namoun, A., Tufail, A., Kim, K. H., & Almoamari, H. (2022). Customer Profiling Using Internet of Things Based Recommendations. Sustainability (Switzerland), 14(18). https://doi.org/10.3390/su141811200
Somya, R., Winarko, E., & Priyanta, S. (2022). A hybrid recommender system based on customer behavior and transaction data using generalized sequential pattern algorithm. Bulletin of Electrical Engineering and Informatics, 11(6), 3422–3432. https://doi.org/10.11591/eei.v11i6.4021
Bonney, S. O., Song, J., Jingwei, Z., & Peng, Y. (2022). Consumer preference for cement brands used in concrete production: the Ghanaian perspective. Cogent Engineering, 9(1). https://doi.org/10.1080/23311916.2022.2062876
Xiao, M., Zhou, Q., Lu, L., Tao, X., He, W., & Zhou, Y. (2022). Applying Deep Learning-Based Personalized Item Recommendation for Mobile Service in Retailor Industry. Mobile Information Systems, 2022. https://doi.org/10.1155/2022/2364154
Gunawan, A. A., Bloemer, J., van Riel, A. C. R., & Essers, C. (2022). Institutional Barriers and Facilitators of Sustainability for Indonesian Batik SMEs: A Policy Agenda. Sustainability (Switzerland), 14(14). https://doi.org/10.3390/su14148772
Wu, M. Y., Ke, C. K., & Lai, S. C. (2022). Optimizing the Routing of Urban Logistics by Context-Based Social Network and Multi-Criteria Decision Analysis. Symmetry, 14(9). https://doi.org/10.3390/sym14091811
Yu, C. L., Shu, M. H., Ho, P. T., & Huang, J. C. (2022). Consumer Behavior Based on Big Data and Cloud Computing and Construction of Customer Perception Mobile Terminal System. Security and Communication Networks, 2022. https://doi.org/10.1155/2022/7531530
Mahmoud, M. A., Tsetse, E. K. K., Tulasi, E. E., & Muddey, D. K. (2022). Green Packaging, Environmental Awareness, Willingness to Pay and Consumers’ Purchase Decisions. Sustainability (Switzerland), 14(23). https://doi.org/10.3390/su142316091
Dogan, O., Kem, F. C., & Oztaysi, B. (2022). Fuzzy association rule mining approach to identify e-commerce product association considering sales amount. Complex and Intelligent Systems, 8(2), 1551–1560. https://doi.org/10.1007/s40747-021-00607-3
Jeong, J., Kim, D., Li, X., Li, Q., Choi, I., & Kim, J. (2022). An Empirical Investigation of Personalized Recommendation and Reward Effect on Customer Behavior: A Stimulus–Organism–Response (SOR) Model Perspective. Sustainability (Switzerland), 14(22). https://doi.org/10.3390/su142215369
Esheiba, L., Helal, I. M. A., Elgammal, A., & El-Sharkawi, M. E. (2022). A Data Warehouse-Based System for Service Customization Recommendations in Product-Service Systems. Sensors, 22(6). https://doi.org/10.3390/s22062118
Ahmad, A., Leifler, O., & Sandahl, K. (2022). Data visualisation in continuous integration and delivery: Information needs, challenges, and recommendations. IET Software, 16(3), 331–349. https://doi.org/10.1049/sfw2.12030
Sulikowski, P., Kucznerowicz, M., Bąk, I., Romanowski, A., & Zdziebko, T. (2022). Online Store Aesthetics Impact Efficacy of Product Recommendations and Highlighting. Sensors, 22(23). https://doi.org/10.3390/s22239186
Li, S., Zhu, B., Zhu, H., Liu, F., Zhang, Y., Wang, R., & Lu, H. (2022). Heterogeneous Attention Concentration Link Prediction Algorithm for Attracting Customer Flow in Online Brand Community. IEEE Access, 10, 20898–20912. https://doi.org/10.1109/ACCESS.2022.3151112
Kafkas, K., Perdahçı, Z. N., & Aydın, M. N. (2021). Discovering customer purchase patterns in product communities: An empirical study on co-purchase behavior in an online marketplace. Journal of Theoretical and Applied Electronic Commerce Research, 16(7), 2965–2980. https://doi.org/10.3390/jtaer16070162
Pazhaniraja, N., Sountharrajan, S., Suganya, E., & Karthiga, M. (2021). Top ‘N’ Variant Random Forest Model for High Utility Itemsets Recommendation. EAI Endorsed Transactions on Energy Web, 8(35), 1–7. https://doi.org/10.4108/eai.25-1-2021.168225
Vahidi Farashah, M., Etebarian, A., Azmi, R., & Ebrahimzadeh Dastjerdi, R. (2021). A hybrid recommender system based-on link prediction for movie baskets analysis. Journal of Big Data, 8(1). https://doi.org/10.1186/s40537-021-00422-0
Yin, H., Hu, Z., Peng, Y., Wang, Z., Xu, G., & Xu, Y. (2021). Deep semi-supervised learning with weight map for review helpfulness prediction. Computer Science and Information Systems, 18(4), 1159–1174. https://doi.org/10.2298/CSIS201228044Y
Pasquier, N., & Chatterjee, S. (2021). Customer choice modelling: A multilevel consensus clustering approach. Annals of Emerging Technologies in Computing, 5(2), 103–120. https://doi.org/10.33166/AETiC.2021.02.009
Chen, G., & Li, Z. (2021). A new method combining pattern prediction and preference prediction for next basket recommendation. Entropy, 23(11). https://doi.org/10.3390/e23111430
Al-Safi, J., & Kaleli, C. (2021). Item genre-based users similarity measure for recommender systems. Applied Sciences (Switzerland), 11(13). https://doi.org/10.3390/app11136108
Fu, L., & Ma, X. (2021). An Improved Recommendation Method Based on Content Filtering and Collaborative Filtering. Complexity, 2021. https://doi.org/10.1155/2021/5589285
Cassaniti, J., Kumoji, K., Rariewa, F., Ohkubo, S., & Oyenubi, O. (2021). Influence networks relating to health knowledge among nairobi⇔s micro-retailers and their clients. Electronic Journal of Knowledge Management, 18(3), 302–324. https://doi.org/10.34190/EJKM.18.3.2068
Kazmi, S. H. A., Ahmed, R. R., Soomro, K. A., Hashem E, A. R., Akhtar, H., & Parmar, V. (2021). Role of augmented reality in changing consumer behavior and decision making: Case of Pakistan. Sustainability (Switzerland), 13(24). https://doi.org/10.3390/su132414064
Le, N. B. Van, & Huh, J. H. (2021). Applying sentiment product reviews and visualization for bi systems in vietnamese e-commerce website: Focusing on vietnamese context. Electronics (Switzerland), 10(20). https://doi.org/10.3390/electronics10202481
Uz Zaman, M. W., Hafeez, Y., Hussain, S., Anwaar, H., Yang, S., Ali, S., Abbasi, A. A., & Song, O. Y. (2021). Recommender System for Configuration Management Process of Entrepreneurial Software Designing Firms. Computers, Materials and Continua, 67(2), 2373–2391. https://doi.org/10.32604/cmc.2021.015112
Alaa, R., Gawish, M., & Fernández-Veiga, M. (2021). Improving recommendations for online retail markets based on ontology evolution. Electronics (Switzerland), 10(14). https://doi.org/10.3390/electronics10141650
Fang, B., Hu, E., Shen, J., Zhang, J., & Chen, Y. (2021). Implicit Feedback Recommendation Method Based on User-Generated Content. Scientific Programming, 2021. https://doi.org/10.1155/2021/3982270
Schröter, I., Grillo, N. R., Limpak, M. K., Mestiri, B., Osthold, B., Sebti, F., & Mergenthaler, M. (2021). Webcam eye tracking for monitoring visual attention in hypothetical online shopping tasks. Applied Sciences (Switzerland), 11(19). https://doi.org/10.3390/app11199281
Chindyana, M., & Wulandhari, L. A. (2021). Segmentation of tourist interest on tourism object categories by comparing PSO K-means and DBSCAN method. Revue d’Intelligence Artificielle, 35(1), 23–37. https://doi.org/10.18280/ria.350103
Kuang, D., Li, X. F., & Bi, W. W. (2021). How to effectively design referral rewards to increase the referral likelihood for green products. Sustainability (Switzerland), 13(13). https://doi.org/10.3390/su13137177
Esheiba, L., Elgammal, A., Helal, I. M. A., & El-Sharkawi, M. E. (2021). A hybrid knowledge-based recommender for product-service systems mass customization. Information (Switzerland), 12(8). https://doi.org/10.3390/info12080296
Giat, Y., & Bouhnik, D. (2021). A decision support system and warehouse operations design for pricing products and minimizing product returns in a food plant. Interdisciplinary Journal of Information, Knowledge, and Management, 16, 39–54. https://doi.org/10.28945/4692
Simeone, A., Zeng, Y., & Caggiano, A. (2021). Intelligent decision-making support system for manufacturing solution recommendation in a cloud framework. International Journal of Advanced Manufacturing Technology, 112(3–4), 1035–1050. https://doi.org/10.1007/s00170-020-06389-1
Naous, D., & Legner, C. (2021). Leveraging market research techniques in is: A review and framework of conjoint analysis studies in the is discipline. Communications of the Association for Information Systems, 49, 126–155. https://doi.org/10.17705/1CAIS.04906
Sharma, S., Koehl, L., Bruniaux, P., Zeng, X., & Wang, Z. (2021). Development of an intelligent data-driven system to recommend personalized fashion design solutions. Sensors, 21(12). https://doi.org/10.3390/s21124239
Wu, Y., Huo, Z., Xing, W., Ma, Z., & Ahmed Aldeeb, H. M. (2021). Application of experience economy and recommendation algorithm in tourism reuse of industrial wasteland. Applied Mathematics and Nonlinear Sciences, 6(2), 227–238. https://doi.org/10.2478/amns.2021.2.00039
Suresh, A., & Carmel Mary Belinda, M. J. (2021). Accelerated - Generic gradient descent for e-commerce recommender systems. Indian Journal of Computer Science and Engineering, 12(1), 135–147. https://doi.org/10.21817/indjcse/2021/v12i1/211201157
Wu, C. H., Wang, Y., & Ma, J. (2021). Maximal Marginal Relevance-Based Recommendation for Product Customisation. Enterprise Information Systems. https://doi.org/10.1080/17517575.2021.1992018
Yoon, Y., Fu, Y., & Joo, J. (2021). Unintended CSR violation caused by online recommendation. Sustainability (Switzerland), 13(7). https://doi.org/10.3390/su13074053
Ye, X., & Chen, M. (2021). Personalized Recommendation for Mobile Internet Wealth Management Based on User Behavior Data Analysis. Scientific Programming, 2021. https://doi.org/10.1155/2021/9326932
Ohashi, F. K., Gaspar, M. A., Costa, I., Martins, F. S., & de Magalhães, F. L. F. (2021). Recommender System Architecture to support salesperson using Sales Force Automation Systems In large product portfolio companies. RISTI - Revista Iberica de Sistemas e Tecnologias de Informacao, 42, 46–61. https://doi.org/10.17013/RISTI.42.46-61
Kit, T. C., & Azmi, N. F. M. (2021). Customer Profiling for Malaysia Online Retail Industry using K-Means Clustering and RM Model. International Journal of Advanced Computer Science and Applications, 12(1), 106–113. https://doi.org/10.14569/IJACSA.2021.0120114
Werder, K., Richter, J., Hennel, P., Dreesen, T., Fischer, M., & Weingarth, J. (2021). A Three-Pronged View on Organizational Agility. IT Professional, 23(2), 89–95. https://doi.org/10.1109/MITP.2020.3016488
Korösi, G., & Vinkó, T. (2021). A Practical Framework for Real Life Webshop Sales Promotion Targeting. Informatica (Slovenia), 45(4), 625–632. https://doi.org/10.31449/inf.v45i4.3066
Olsson, T., Wnuk, K., & Jansen, S. (2021). A validated model for the scoping process of quality requirements: a multi-case study. Empirical Software Engineering, 26(2). https://doi.org/10.1007/s10664-020-09896-7
Islam, M. M., & Baek, J. H. (2021). Deep learning based real age and gender estimation from unconstrained face image towards smart store customer relationship management. Applied Sciences (Switzerland), 11(10). https://doi.org/10.3390/app11104549
Feng, D., Fu, X., Jiang, S., & Jing, L. (2021). Conceptual solution decision based on rough sets and shapley value for product‐service system: Customer value‐economic objective trade‐off perspective. Applied Sciences (Switzerland), 11(22). https://doi.org/10.3390/app112211001
Hasnul Hadi, M. H., Ker, P. J., Thiviyanathan, V. A., Tang, S. G. H., Leong, Y. S., Lee, H. J., Hannan, M. A., Jamaludin, M. Z., & Mahdi, M. A. (2021). The amber-colored liquid: A review on the color standards, methods of detection, issues and recommendations. Sensors, 21(20). https://doi.org/10.3390/s21206866
Stöckli, D. R., & Khobzi, H. (2021). Recommendation systems and convergence of online reviews: The type of product network matters! Decision Support Systems, 142. https://doi.org/10.1016/j.dss.2020.113475
Hanafi, & Mohd Aboobaider, B. (2021). Word Sequential Using Deep LSTM and Matrix Factorization to Handle Rating Sparse Data for E-Commerce Recommender System. Computational Intelligence and Neuroscience, 2021. https://doi.org/10.1155/2021/8751173
Sadreddini, Z., Donmez, I., & Yanikomeroglu, H. (2021). Cancel-for-Any-Reason Insurance Recommendation Using Customer Transaction-Based Clustering. IEEE Access, 9, 39363–39374. https://doi.org/10.1109/ACCESS.2021.3064929
Kobayashi, M., & Takeda, T. (2020). Product recommendation based on analysis of aesthetic elements used to customer’s favorite products. Computer-Aided Design and Applications, 18(4), 682–691. https://doi.org/10.14733/cadaps.2021.682-691
Goyani, M., & Chaurasiya, N. (2020). A Review of Movie Recommendation System: Limitations, Survey and Challenges. Electronic Letters on Computer Vision and Image Analysis, 19(3), 18–37. https://doi.org/10.5565/rev/elcvia.1232
Lee, D., Gopal, A., & Park, S. H. (2020). Different but equal? a field experiment on the impact of recommendation systems on mobile and personal computer channels in retail. Information Systems Research, 31(3), 892–912. https://doi.org/10.1287/ISRE.2020.0922
Wu, Z., Li, L., & Liu, H. (2020). Process Knowledge Recommendation System for Mechanical Product Design. IEEE Access, 8, 112795–112804. https://doi.org/10.1109/ACCESS.2020.3002922
Hu, S., Kumar, A., Al-Turjman, F., Gupta, S., Seth, S., & Shubham. (2020). Reviewer Credibility and Sentiment Analysis Based User Profile Modelling for Online Product Recommendation. IEEE Access, 8, 26172–26189. https://doi.org/10.1109/ACCESS.2020.2971087
Abbas, K., Afaq, M., Khan, T. A., & Song, W. C. (2020). A blockchain and machine learning-based drug supply chain management and recommendation system for smart pharmaceutical industry. Electronics (Switzerland), 9(5). https://doi.org/10.3390/electronics9050852
Liu, R., Liu, R., Pugliese, A., & Subrahmanian, V. S. (2020). STARS: Defending against Sockpuppet-Based Targeted Attacks on Reviewing Systems. ACM Transactions on Intelligent Systems and Technology, 11(5). https://doi.org/10.1145/3397463
Wang, G., & Wu, N. (2020). A Comparative Study on Contract Recommendation Model: Using Macao Mobile Phone Datasets. IEEE Access, 8, 39747–39757. https://doi.org/10.1109/ACCESS.2020.2975029
Diao, W., Saxena, S., & Pecht, M. G. (2020). Analysis of Specified Capacity in Power Banks. IEEE Access, 8, 21326–21332. https://doi.org/10.1109/ACCESS.2020.2969410
Li, Z., Wang, H., Ding, D., Hu, S., Zhang, Z., Liu, W., Gao, J., Zhang, Z., & Zhang, J. (2020). Deep Interest-Shifting Network with Meta-Embeddings for Fresh Item Recommendation. Complexity, 2020. https://doi.org/10.1155/2020/8828087
Abugabah, A., Cheng, X., & Wang, J. (2020). Learning context-aware outfit recommendation. Symmetry, 12(6). https://doi.org/10.3390/SYM12060873
Lamberz, J., Litfin, T., Teckert, Ö., & Meeh-Bunse, G. (2020). Is there a Link between Sustainability, Perception and Buying Decision at the Point of Sale? Business Systems Research, 11(3), 1–13. https://doi.org/10.2478/bsrj-2020-0023
Fabra, J., Álvarez, P., & Ezpeleta, J. (2020). Log-based session profiling and online behavioral prediction in e-commerce websites. IEEE Access, 8, 171834–171850. https://doi.org/10.1109/ACCESS.2020.3024649
Ullah, F., Zhang, B., & Khan, R. U. (2020). Image-Based Service Recommendation System: A JPEG-Coefficient RFs Approach. IEEE Access, 8, 3308–3318. https://doi.org/10.1109/ACCESS.2019.2962315
Cao, R., Zhang, X., & Wang, H. (2020). A Review Semantics Based Model for Rating Prediction. IEEE Access, 8, 4714–4723. https://doi.org/10.1109/ACCESS.2019.2962075
Chodak, G. (2020). The problem of shelf-warmers in electronic commerce: a proposed solution. Information Systems and E-Business Management, 18(2), 259–280. https://doi.org/10.1007/s10257-020-00473-5
Gunjal, S. N., Yadav, S. K., & Kshirsagar, D. B. (2020). A distributed item based similarity approach for collaborative filtering on hadoop framework. Advances in Parallel Computing, 37, 407–415. https://doi.org/10.3233/APC200176
Kala, K. U., & Nandhini, M. (2020). Gated recurrent unit architecture for context-aware recommendations with improved similarity measures. KSII Transactions on Internet and Information Systems, 14(2), 538–561. https://doi.org/10.3837/tiis.2020.02.004
Lee, H. I., Choi, I. Y., Moon, H. S., & Kim, J. K. (2020). A multi-period product recommender system in online food market based on recurrent neural networks. Sustainability (Switzerland), 12(3). https://doi.org/10.3390/su12030969
Jintana, J., Sopadang, A., & Ramingwong, S. (2020). Matching consignees/shippers recommendation system in courier service using data analytics. Applied Sciences (Switzerland), 10(16). https://doi.org/10.3390/app10165585
Chu, P. M., Mao, Y. S., Lee, S. J., & Hou, C. L. (2020). Leveraging user comments for recommendation in E-commerce. Applied Sciences (Switzerland), 10(7). https://doi.org/10.3390/app10072540
Babeetha, S., Muruganantham, B., Ganesh Kumar, S., & Murugan, A. (2020). An enhanced kernel weighted collaborative recommended system to alleviate sparsity. International Journal of Electrical and Computer Engineering, 10(1), 447–454. https://doi.org/10.11591/ijece.v10i1.pp447-454
Khalid, J., Abbas, A., Mahmood, M. Q., Tariq, A., Khatoon, M., Azhar, S., Akbar, R., Rafia, Akbar, A., Meer, A., & Ud Din, M. J. (2020). Significance of electronic word of mouth (e-WOM) in opinion formation. International Journal of Advanced Computer Science and Applications, 2, 537–544. https://doi.org/10.14569/ijacsa.2020.0110268
Al-Hadi, I. A. A. Q., Sharef, N. M., Sulaiman, M. N., Mustapha, N., & Nilashi, M. (2020). Latent Based Temporal Optimization Approach for Improving the Performance of Collaborative Filtering. PeerJ Computer Science, 6. https://doi.org/10.7717/PEERJ-CS.331
Pradeep, I. K., Bhaskar, M. J., & Satyanarayana, B. (2020). Data science and deep learning applications in the e-commerce industry: A survey. Indian Journal of Computer Science and Engineering, 11(5), 497–509. https://doi.org/10.21817/indjcse/2020/v11i5/201105135
Shafqat, W., & Byun, Y. C. (2020). Enabling ‘untact’ culture via online product recommendations: An optimized graph-CNN based approach. Applied Sciences (Switzerland), 10(16). https://doi.org/10.3390/APP10165445
Anoop, V. S., & Asharaf, S. (2020). Aspect-oriented sentiment analysis: A topic modeling-powered approach. Journal of Intelligent Systems, 29(1), 1166–1178. https://doi.org/10.1515/jisys-2018-0299
Ehikioya, S. A., & Lu, S. (2020). A traffic tracking analysis model for the effective management of e-commerce transactions. International Journal of Networked and Distributed Computing, 8(3), 171–193. https://doi.org/10.2991/ijndc.k.200515.006
Li, W., & Xu, B. (2020). Aspect-Based Fashion Recommendation with Attention Mechanism. IEEE Access, 8, 141814–141823. https://doi.org/10.1109/ACCESS.2020.3013639
Wang, D., & Chen, Y. (2020). A novel many-objective recommendation algorithm for multistakeholders. IEEE Access, 8, 196482–196499. https://doi.org/10.1109/ACCESS.2020.3034716
Pan, H. (2020). Consumer engagement in online brand communities: Community values, brand symbolism and social strategies. Interdisciplinary Journal of Information, Knowledge, and Management, 15, 65–90. https://doi.org/10.28945/4536
Beheshti, A., Yakhchi, S., Mousaeirad, S., Ghafari, S. M., Goluguri, S. R., & Edrisi, M. A. (2020). Towards cognitive recommender systems. Algorithms, 13(8). https://doi.org/10.3390/A13080176
Shahbazi, Z., Hazra, D., Park, S., & Byun, Y. C. (2020). Toward improving the prediction accuracy of product recommendation system using extreme gradient boosting and encoding approaches. Symmetry, 12(9). https://doi.org/10.3390/SYM12091566
Pratama, B. Y., Budi, I., & Yuliawati, A. (2020). Product recommendation in offline retail industry by using collaborative filtering. International Journal of Advanced Computer Science and Applications, 11(9), 635–643. https://doi.org/10.14569/IJACSA.2020.0110975
Bai, Y., Jia, S., Wang, S., & Tan, B. (2020). Customer loyalty improves the effectiveness of recommender systems based on complex network. Information (Switzerland), 11(3). https://doi.org/10.3390/INFO11030171
Khrais, L. T. (2020). Role of artificial intelligence in shaping consumer demand in e-commerce. Future Internet, 12(12), 1–14. https://doi.org/10.3390/fi12120226
Sundar, A. P., Li, F., Zou, X., Gao, T., & Russomanno, E. D. (2020). Understanding shilling attacks and their detection traits: A comprehensive survey. IEEE Access, 8, 171703–171715. https://doi.org/10.1109/ACCESS.2020.3022962
Naicker, N., & Maharaj, M. S. (2020). Investigating agile requirements engineering practices in the South African software development market. Journal of Computing and Information Technology, 28(1), 33–58. https://doi.org/10.20532/cit.2020.1004868
Aspeling, J. M., & Mason, R. B. (2020). Towards an e-learning support strategy for the retail sector in South Africa. International Journal of Web-Based Learning and Teaching Technologies, 15(3), 1–18. https://doi.org/10.4018/IJWLTT.2020070101
Jaffali, S., Jamoussi, S., Smaili, K., & Ben Hamadou, A. (2020). Like-tasted user groups to predict ratings in recommender systems. Social Network Analysis and Mining, 10(1). https://doi.org/10.1007/s13278-020-00643-w
Holder, C. J., Ricketts, S., & Obara, B. (2020). Convolutional networks for appearance-based recommendation and visualisation of mascara products. Machine Vision and Applications, 31(1). https://doi.org/10.1007/s00138-019-01053-5
Hu, H., Tang, Y., Xie, Y., Dai, Y., & Dai, W. (2020). Cognitive computation on consumer’s decision making of internet financial products based on neural activity data. Computer Science and Information Systems, 17(2), 689–704. https://doi.org/10.2298/CSIS200229013H
Chaudhary, N., & Chowdhury, D. R. (2019). Data preprocessing for evaluation of recommendation models in E-commerce. Data, 4(1). https://doi.org/10.3390/data4010023
Shoja, B. M., & Tabrizi, N. (2019). Customer Reviews Analysis with Deep Neural Networks for E-Commerce Recommender Systems. IEEE Access, 7, 119121–119130. https://doi.org/10.1109/ACCESS.2019.2937518
Yeh, H., & Kuo, F. (2019). Effects of online advocacy banners on customers after abandoning products in online shopping carts. Interdisciplinary Journal of Information, Knowledge, and Management, 14, 165–181. https://doi.org/10.28945/4310
Górajski, M., & Machowska, D. (2019). How do loyalty programs affect goodwill? An optimal control approach. 4or, 17(3), 297–316. https://doi.org/10.1007/s10288-018-0386-2
Long, H. V., Son, L. H., Khari, M., Arora, K., Chopra, S., Kumar, R., Le, T., & Baik, S. W. (2019). A new approach for construction of geodemographic segmentation model and prediction analysis. Computational Intelligence and Neuroscience, 2019. https://doi.org/10.1155/2019/9252837
Sariki, T. P., & Bharadwaja Kumar, G. (2019). Exploiting visual content of book front cover to aggrandize the content based book recommendation system. International Journal of Innovative Technology and Exploring Engineering, 8(12), 1631–1634. https://doi.org/10.35940/ijitee.L3158.1081219
Rahman, M. M., & Hasan, M. K. (2019). A framework for predicting personalized product packages. International Journal of Advanced Trends in Computer Science and Engineering, 8(4), 1069–1075. https://doi.org/10.30534/ijatcse/2019/13842019
Poirson, E., & Cunha, C. Da. (2019). A recommender approach based on customer emotions. Expert Systems with Applications, 122, 281–288. https://doi.org/10.1016/j.eswa.2018.12.035
Guo, B., Liu, Y., Ouyang, Y., Zheng, V. W., Zhang, D., & Yu, Z. (2019). Harnessing the Power of the General Public for Crowdsourced Business Intelligence: A Survey. IEEE Access, 7, 26606–26630. https://doi.org/10.1109/ACCESS.2019.2901027
Nelaturi, N., & Devi, G. L. (2019). A product recommendation model based on recurrent neural network. Journal Europeen Des Systemes Automatises, 52(5), 501–507. https://doi.org/10.18280/jesa.520509
Díez, J., Martínez-Rego, D., Alonso-Betanzos, A., Luaces, O., & Bahamonde, A. (2019). Optimizing novelty and diversity in recommendations. Progress in Artificial Intelligence, 8(1), 101–109. https://doi.org/10.1007/s13748-018-0158-4
Lee, D., & Hosanagar, K. (2019). How do recommender systems affect sales diversity? A cross-category investigation via randomized field experiment. Information Systems Research, 30(1), 239–259. https://doi.org/10.1287/isre.2018.0800
Lytvyn, V., Vysotska, V., Demchuk, A., Demkiv, I., Ukhanska, O., Hladun, V., Kovalchuk, R., Petruchenko, O., Dzyubyk, L., & Sokulska, N. (2019). Design of the architecture of an intelligent system for distributing commercial content in the internet space based on seo-technologies, neural networks, and machine learning. Eastern-European Journal of Enterprise Technologies, 2(2–98), 15–34. https://doi.org/10.15587/1729-4061.2019.164441
Ziemba, E., Eisenbardt, M., Mullins, R., & Dettmer, S. (2019). Prosumers’ engagement in business process innovation – the case of Poland and the UK. Interdisciplinary Journal of Information, Knowledge, and Management, 14, 119–143. https://doi.org/10.28945/4320
Penumetsa, H. K., & Mogalla, S. (2019). Ml based naive bayes methodology for rate prediction using textual rating and find actual or movie rating based on mbnbr optimization. International Journal of Innovative Technology and Exploring Engineering, 8(10), 314–321. https://doi.org/10.35940/ijitee.I8232.0881019
Bag, S., Ghadge, A., & Tiwari, M. K. (2019). An integrated recommender system for improved accuracy and aggregate diversity. Computers and Industrial Engineering, 130, 187–197. https://doi.org/10.1016/j.cie.2019.02.028
Sahoo, K., Samal, A. K., Pramanik, J., & Pani, S. K. (2019). Exploratory data analysis using python. International Journal of Innovative Technology and Exploring Engineering, 8(12), 4727–4735. https://doi.org/10.35940/ijitee.L3591.1081219
Saravana Kumar, S., & Murali, S. (2019). Brand awareness and preference towards qualcomm snapdragon in mobile among consumers. International Journal of Management, 10(4), 9–18. https://doi.org/10.34218/IJM.10.4.2019.002
Gallagher, C., Furey, E., & Curran, K. (2019). The application of sentiment analysis and text analytics to customer experience reviews to understand what customers are really saying. International Journal of Data Warehousing and Mining, 15(4), 21–47. https://doi.org/10.4018/IJDWM.2019100102
Lammatha, K., Chinnusamy, K., & Malgireddy, N. R. (2019). Swift five force LRP data process algorithm machine learning Ai data process. International Journal of Engineering and Advanced Technology, 8(6 Special Issue), 443–451. https://doi.org/10.35940/ijeat.F1095.0886S19
Shafqat, W., & Byun, Y. C. (2019). Topic predictions and optimized recommendation mechanism based on integrated topic modeling and deep neural networks in crowdfunding platforms. Applied Sciences (Switzerland), 9(24). https://doi.org/10.3390/app9245496
Serrano, W. (2019). Intelligent recommender system for big data applications based on the random neural network. Big Data and Cognitive Computing, 3(1), 1–29. https://doi.org/10.3390/bdcc3010015
Ramzan, B., Bajwa, I. S., Jamil, N., Amin, R. U., Ramzan, S., Mirza, F., & Sarwar, N. (2019). An Intelligent Data Analysis for Recommendation Systems Using Machine Learning. Scientific Programming, 2019. https://doi.org/10.1155/2019/5941096
Chen, K. S., & Yang, C. M. (2019). Quality Capability Assessment for Thin-Film Chip Resistor. IEEE Access, 7, 92511–92516. https://doi.org/10.1109/ACCESS.2019.2927657
Chen, J., & Abdul, A. (2019). A Session-Based Customer Preference Learning Method by Using the Gated Recurrent Units with Attention Function. IEEE Access, 7, 17750–17759. https://doi.org/10.1109/ACCESS.2019.2895647
Sangeetha, J., & Sinthu Janita Prakash, V. (2019). Opinion based memory access algorithms using collaborative filtering in recommender systems. International Journal of Innovative Technology and Exploring Engineering, 8(12), 606–612. https://doi.org/10.35940/ijitee.L3274.1081219
Hwangbo, H., & Kim, Y. (2019). Session-Based Recommender system for sustainable digital marketing. Sustainability (Switzerland), 11(12). https://doi.org/10.3390/SU11123336
Zhang, J. (2019). Personalised product recommendation model based on user interest. Computer Systems Science and Engineering, 34(4), 231–236. https://doi.org/10.32604/CSSE.2019.34.231
Bawiskar, M., & Injamuri, V. (2019). Aspect based mobile recommendation system. International Journal of Innovative Technology and Exploring Engineering, 8(10), 3840–3846. https://doi.org/10.35940/ijitee.J9996.0881019
Jain, A. M., & Carandang, C. B. (2018). Development of an Online Laguna Agricultural Trading Center. International Journal of Computing, 2(4), 131–150. https://doi.org/10.25147/ijcsr.2017.001.1.29
Ram Mohan Rao, P., Murali Krishna, S., & Siva Kumar, A. P. (2018). Privacy preservation techniques in big data analytics: a survey. Journal of Big Data, 5(1). https://doi.org/10.1186/s40537-018-0141-8
Erum, M., Waqas, M., Arshad, S., & Nawaz, T. (2018). Features Analysis of Online Shopping System Using WCM. EAI Endorsed Transactions on Scalable Information Systems, 5(16), 1–8. https://doi.org/10.4108/eai.13-4-2018.154471
Zhang, L., Zhang, H., & Hao, S. M. (2018). An equity fund recommendation system by combing transfer learning and the utility function of the prospect theory. Journal of Finance and Data Science, 4(4), 223–233. https://doi.org/10.1016/j.jfds.2018.02.003
Naveen, N., & Ganesh Kumar, S. (2018). Efficient mining and recommendation of extensive data through collaborative filtering in E-commerce: A survey. International Journal of Engineering and Technology(UAE), 7(2), 331–335. https://doi.org/10.14419/ijet.v7i2.24.12077
Lakshmanaprabu, S. K., Shankar, K., Gupta, D., Khanna, A., Rodrigues, J. J. P. C., Pinheiro, P. R., & de Albuquerque, V. H. C. (2018). Ranking analysis for online customer reviews of products using opinion mining with clustering. Complexity, 2018. https://doi.org/10.1155/2018/3569351
Venugopal, S., & Nagraj, G. (2018). A proficient web recommender system using hybrid possiblistic fuzzy clustering and Bayesian model approach. International Journal of Intelligent Engineering and Systems, 11(6), 190–198. https://doi.org/10.22266/IJIES2018.1231.19
Nasip, I., & Sudarmaji, E. (2018). Managing tax dispute due to IFRS-16 on the retrofits implementation in Indonesia. International Journal of Engineering and Technology(UAE), 7(3), 200–208. https://doi.org/10.14419/ijet.v7i3.21.17160
Girish, S., Ramamurthy, B., & Senthilnathan, T. (2018). Mining the web data for classifying and predicting users’ requests. International Journal of Electrical and Computer Engineering, 8(4), 2390–2398. https://doi.org/10.11591/ijece.v8i4.pp2390-2398
Sfenrianto, S., Saragih, M. H., & Nugraha, B. (2018). E-commerce recommender for usage bandwidth hotel. Indonesian Journal of Electrical Engineering and Computer Science, 9(1), 227–233. https://doi.org/10.11591/ijeecs.v9.i1.pp227-233
Al-Enezi, K. A., Al Shaikhli, I. F. T., & Aldabbagh, S. S. M. (2018). The influence of internet and social media on purchasing decisions in Kuwait. Indonesian Journal of Electrical Engineering and Computer Science, 10(2), 792–797. https://doi.org/10.11591/ijeecs.v10.i2.pp792-797
Ziemba, E., & Eisenbardt, M. (2018). The ways of prosumers’ knowledge sharing with organizations. Interdisciplinary Journal of Information, Knowledge, and Management, 13, 95–115. https://doi.org/10.28945/4067
Guidotti, R., Gabrielli, L., Monreale, A., Pedreschi, D., & Giannotti, F. (2018). Discovering temporal regularities in retail customers’ shopping behavior. EPJ Data Science, 7(1). https://doi.org/10.1140/epjds/s13688-018-0133-0
Kanavos, A., Iakovou, S. A., Sioutas, S., & Tampakas, V. (2018). Large scale product recommendation of supermarket ware based on customer behaviour analysis. Big Data and Cognitive Computing, 2(2), 1–19. https://doi.org/10.3390/bdcc2020011
Magboul, I. H. M., & Abbad, M. (2018). Antecedents and adoption of e-banking in bank performance: The perspective of private bank employees. Interdisciplinary Journal of Information, Knowledge, and Management, 13, 361–381. https://doi.org/10.28945/4132
Zhang, J., Chen, D., & Lu, M. (2018). Combining sentiment analysis with a fuzzy kano model for product aspect preference recommendation. IEEE Access, 6, 59163–59172. https://doi.org/10.1109/ACCESS.2018.2875026
Koolen, D., Sadat-Razavi, N., & Ketter, W. (2017). Machine learning for identifying demand patterns of home energy management systems with dynamic electricity pricing. Applied Sciences (Switzerland), 7(11). https://doi.org/10.3390/app7111160
Aldayel, M., & Ykhlef, M. (2017). A new sentiment case-based recommender. IEICE Transactions on Information and Systems, E100D(7), 1484–1493. https://doi.org/10.1587/transinf.2016EDP7441
Bilici, E., & Saygın, Y. (2017). Why do people (not) like me?: Mining opinion influencing factors from reviews. Expert Systems with Applications, 68, 185–195. https://doi.org/10.1016/j.eswa.2016.10.001
Tewari, A. S., & Barman, A. G. (2017). Collaborative recommendation system using dynamic content based filtering, association rule mining and opinion mining. International Journal of Intelligent Engineering and Systems, 10(5), 57–66. https://doi.org/10.22266/ijies2017.1031.07
Gimenes, G., Cordeiro, R. L. F., & Rodrigues-Jr, J. F. (2017). ORFEL: Efficient detection of defamation or illegitimate promotion in online recommendation. Information Sciences, 379, 274–287. https://doi.org/10.1016/j.ins.2016.09.006
Morawski, J., Stepan, T., Dick, S., & Miller, J. (2017). A Fuzzy Recommender System for Public Library Catalogs. International Journal of Intelligent Systems, 32(10), 1062–1084. https://doi.org/10.1002/int.21884
Allauddin Mulla, R. ., Eknath Pawar, M. ., S. Banait, S. ., N. Ajani, S. ., Pravin Borawake, M. ., & Hundekari, S. . (2023). Design and Implementation of Deep Learning Method for Disease Identification in Plant Leaf. International Journal on Recent and Innovation Trends in Computing and Communication, 11(2s), 278–285. https://doi.org/10.17762/ijritcc.v11i2s.6147
Guo, Y., Wang, M., & Li, X. (2017). An interactive personalized recommendation system using the hybrid algorithm model. Symmetry, 9(10). https://doi.org/10.3390/sym9100216
Yang, M., Ma, Y., & Nie, J. (2017). Research on a personalized recommendation algorithm. International Journal of Grid and Distributed Computing, 10(1), 123–136. https://doi.org/10.14257/ijgdc.2017.10.1.12
Zhang, Q., Wu, D., Lu, J., Liu, F., & Zhang, G. (2017). A cross-domain recommender system with consistent information transfer. Decision Support Systems, 104, 49–63. https://doi.org/10.1016/j.dss.2017.10.002
Ho, C. T., Yang, J. M., & Chen, W. T. (2017). Factors affecting re-usage intentions of virtual communities supporting cosmetic products. Interdisciplinary Journal of Information, Knowledge, and Management, 12, 17–36. https://doi.org/10.28945/3644
Yuen, K. K. F. (2017). The fuzzy cognitive pairwise comparisons for ranking and grade clustering to build a recommender system: An application of smartphone recommendation. Engineering Applications of Artificial Intelligence, 61, 136–151. https://doi.org/10.1016/j.engappai.2017.02.001
Sung, H., Kim, W. J., & Shin, J. I. (2016). The antecedent and consequence of trust in social shopping. International Journal of Security and Its Applications, 10(11), 143–152. https://doi.org/10.14257/ijsia.2016.10.11.13
Choi, K., Suh, Y., & Yoo, D. (2016). Extended collaborative filtering technique for mitigating the sparsity problem. International Journal of Computers, Communications and Control, 11(5), 631–644. https://doi.org/10.15837/ijccc.2016.5.2152
Nam, K., Lee, Z., & Lee, B. G. (2016). How internet has reshaped the user experience of banking service? KSII Transactions on Internet and Information Systems, 10(2), 684–702. https://doi.org/10.3837/tiis.2016.02.014
Molina-Castillo, F. J., Rodriguez-Guirao, A., Lopez-Nicolas, C., & Bouwman, H. (2016). Analysis of mobile pre-payment (pay in advance) and post-payment (pay later) services. International Journal of Mobile Communications, 14(5), 499–517. https://doi.org/10.1504/IJMC.2016.078725
Lei, X., Qian, X., & Zhao, G. (2016). Rating Prediction Based on Social Sentiment from Textual Reviews. IEEE Transactions on Multimedia, 18(9), 1910–1921. https://doi.org/10.1109/TMM.2016.2575738
Preibusch, S., Peetz, T., Acar, G., & Berendt, B. (2016). Shopping for privacy: Purchase details leaked to PayPal. Electronic Commerce Research and Applications, 15, 52–64. https://doi.org/10.1016/j.elerap.2015.11.004
Mr. Ather Parvez Abdul Khalil. (2012). Healthcare System through Wireless Body Area Networks (WBAN) using Telosb Motes. International Journal of New Practices in Management and Engineering, 1(02), 01 - 07. Retrieved from http://ijnpme.org/index.php/IJNPME/article/view/4
Lu, J., Wu, D., Mao, M., Wang, W., & Zhang, G. (2015). Recommender system application developments: A survey. Decision Support Systems, 74, 12–32. https://doi.org/10.1016/j.dss.2015.03.008
Zhou, D., & Yao, Z. (2015). Optimal referral reward considering customer’s budget constraint. Future Internet, 7(4), 516–529. https://doi.org/10.3390/fi7040516
Sneha, Y. S., & Mahadevan, G. (2015). A novel approach to personalized recommender systems based on multi criteria ratings. Research Journal of Applied Sciences, Engineering and Technology, 9(10), 841–849. https://doi.org/10.19026/rjaset.9.2633
Oyza, I., & Edwin, A. M. (2015). Effectiveness of social media networks as a strategic tool for organizational marketing management. Journal of Internet Banking and Commerce, 1. https://doi.org/10.4172/1204-5357.S2-006
Wu, D., Zhang, G., & Lu, J. (2015). A fuzzy preference tree-based recommender system for personalized business-to-business e-services. IEEE Transactions on Fuzzy Systems, 23(1), 29–43. https://doi.org/10.1109/TFUZZ.2014.2315655
Honda, K., Nakano, T., Oh, C. H., Ubukata, S., & Notsu, A. (2015). Partially exclusive item partition in MMMs-induced fuzzy co-clustering and its effects in collaborative filtering. Journal of Advanced Computational Intelligence and Intelligent Informatics, 19(6), 810–817. https://doi.org/10.20965/jaciii.2015.p0810
Jatain, R. ., & Jailia, M. . (2023). Automatic Human Face Detection and Recognition Based On Facial Features Using Deep Learning Approach. International Journal on Recent and Innovation Trends in Computing and Communication, 11(2s), 268–277. https://doi.org/10.17762/ijritcc.v11i2s.6146
Hsieh, H. C., Chen, Y. C., & Lin, H. C. (2014). More precise: Stores recommendation under O2O commerce. International Journal of Computing and Digital Systems, 3(2), 91–99. https://doi.org/10.12785/IJCDS/030203
Zhong, H., Zhang, S., Wang, Y., & Shu, Y. (2014). Study on directed trust graph based recommendation for e-commerce system. International Journal of Computers, Communications and Control, 9(4), 510–523. https://doi.org/10.15837/ijccc.2014.4.228
Kalaivani, P., & Shunmuganathan, K. L. (2014). An agent based framework for sentiment classification of online reviews using ontology. Journal of Computer Science, 10(5), 809–820. https://doi.org/10.3844/jcssp.2014.809.820
Brooks, A. L. (2014). Disruptive innovation in healthcare and rehabilitation. Studies in Computational Intelligence, 536, 323–351. https://doi.org/10.1007/978-3-642-45432-5_16
Casarejos, F., Frota, M. N., Penha-Lopes, G., Silva, V. V., & Particelli, F. (2014). Commitment to emissions restrictions of major consumers of electricity in Brazil. Sustainability (Switzerland), 6(9), 6377–6399. https://doi.org/10.3390/su6096377
Mesquida, A. L., Mas, A., Feliu, T. S., & Arcilla, M. (2014). Integración de Estándares de Gestión de TI mediante MIN-ITs. RISTI - Revista Iberica de Sistemas e Tecnologias de Informacao, E1, 31–45. https://doi.org/10.4304/risti.e1.31-45
Wang, T., & Wang, D. (2014). Why Amazon’s ratings might mislead you: The story of herding effects. Big Data, 2(4), 196–204. https://doi.org/10.1089/big.2014.0063
Vera-Del-Campo, J., Pegueroles, J., Hernández-Serrano, J., & Soriano, M. (2014). DocCloud: A document recommender system on cloud computing with plausible deniability. Information Sciences, 258, 387–402. https://doi.org/10.1016/j.ins.2013.04.007
Apeh, E., Gabrys, B., & Schierz, A. (2014). Customer profile classification: To adapt classifiers or to relabel customer profiles? Neurocomputing, 132, 3–13. https://doi.org/10.1016/j.neucom.2013.07.048
Jeong, W. H., Kim, S. J., Park, D. S., & Kwak, J. (2013). Performance improvement of a movie recommendation system based on personal propensity and secure collaborative filtering. Journal of Information Processing Systems, 9(1), 157–172. https://doi.org/10.3745/JIPS.2013.9.1.157
Jing, Y., & Liu, H. (2013). A model for collaborative filtering recommendation in E-commerce environment. International Journal of Computers, Communications and Control, 8(4), 560–570. https://doi.org/10.15837/ijccc.2013.4.577
Zhang, Z., Lin, H., Liu, K., Wu, D., Zhang, G., & Lu, J. (2013). A hybrid fuzzy-based personalized recommender system for telecom products/services. Information Sciences, 235, 117–129. https://doi.org/10.1016/j.ins.2013.01.025
Haruyama, S., Kim, S. K., Beiter, K. A., Dijkema, G. P. J., & De Weck, O. L. (2013). A new project-based curriculum of design thinking with systems engineering techniques. International Journal of System of Systems Engineering, 4(2), 162–173. https://doi.org/10.1504/IJSSE.2013.056296
Lu, H., Chen, Y., & Du, J. (2013). An interactive system based on Kansei Engineering to support clothing design process. Research Journal of Applied Sciences, Engineering and Technology, 6(24), 4531–4535. https://doi.org/10.19026/rjaset.6.3462
Yoon, V. Y., Hostler, R. E., Guo, Z., & Guimaraes, T. (2013). Assessing the moderating effect of consumer product knowledge and online shopping experience on using recommendation agents for customer loyalty. Decision Support Systems, 55(4), 883–893. https://doi.org/10.1016/j.dss.2012.12.024
Gómez-Barroso, J. L., Bacigalupo, M., Nikolov, S. G., Compañó, R., & Feijóo, C. (2012). Factors required for mobile search going mainstream. Online Information Review, 36(6), 846–857. https://doi.org/10.1108/14684521211287918
Kujala, S., Roto, V., Väänänen-Vainio-Mattila, K., Karapanos, E., & Sinnelä, A. (2011). UX Curve: A method for evaluating long-term user experience. Interacting with Computers, 23(5), 473–483. https://doi.org/10.1016/j.intcom.2011.06.005
Sepulveda, J., Gonzalez, J., Alfaro, M., & Camargo, M. (2010). A metrics-based diagnosis tool for enhancing innovation capabilities in SMEs. International Journal of Computers, Communications and Control, 5(5), 919–928. https://doi.org/10.15837/ijccc.2010.5.2255
Rodriguez, R. R., Ortiz, A., & Alfaro, J. J. (2009). Fostering collaborative meta-value chain practices. International Journal of Computer Integrated Manufacturing, 22(5), 385–394. https://doi.org/10.1080/09511920802389546
Dholakia, U. M., Blazevic, V., Wiertz, C., & Algesheimer, R. (2009). Communal service delivery: How customers benefit from ParticiPation in Firm-hosted virtual P3 communities. Journal of Service Research, 12(2), 208–226. https://doi.org/10.1177/1094670509338618
Martínez, L., Pérez, L. G., & Barranco, M. (2007). A multigranular linguistic content-based recommendation model. International Journal of Intelligent Systems, 22(5), 419–434. https://doi.org/10.1002/int.20207
Farsani, H. K., & Nematbakhsh, M. A. (2007). Designing a catalog management system - An ontology approach. Malaysian Journal of Computer Science, 20(1), 35–50. https://doi.org/10.22452/mjcs.vol20no1.4
Zhang, X., Edwards, J., & Harding, J. (2007). Personalised online sales using web usage data mining. Computers in Industry, 58(8–9), 772–782. https://doi.org/10.1016/j.compind.2007.02.004
Kim, J. W., Lee, K. M., Shaw, M. J., Chang, H. L., Nelson, M., & Easley, R. F. (2006). A preference scoring technique for personalized advertisements on Internet storefronts. Mathematical and Computer Modelling, 44(1–2), 3–15. https://doi.org/10.1016/j.mcm.2004.12.011
Guan, S. U., Chan, T. K., & Zhu, F. (2005). Evolutionary intelligent agents for e-commerce: Generic preference detection with feature analysis. Electronic Commerce Research and Applications, 4(4), 377–394. https://doi.org/10.1016/j.elerap.2005.07.002
Huang, Z., Chen, H., & Zeng, D. (2004). Applying associative retrieval techniques to alleviate the sparsity problem in collaborative filtering. ACM Transactions on Information Systems, 22(1), 116–142. https://doi.org/10.1145/963770.963775
Jannach, D., & Kreutler, G. (2004). A knowledge-based framework for the rapid development of conversational recommenders. Lecture Notes in Computer Science (Including Subseries Lecture Notes in Artificial Intelligence and Lecture Notes in Bioinformatics), 3306, 390–402. https://doi.org/10.1007/978-3-540-30480-7_40
Huang, Z., Chung, W., & Chen, H. (2004). A graph model for e-commerce recommender systems. Journal of the American Society for Information Science and Technology, 55(3), 259–274. https://doi.org/10.1002/asi.10372
Cheung, K. W., Tsui, K. C., & Liu, J. (2004). Extended Latent Class Models for Collaborative Recommendation. IEEE Transactions on Systems, Man, and Cybernetics Part A:Systems and Humans., 34(1), 143–148. https://doi.org/10.1109/TSMCA.2003.818877
Weng, S. S., & Liu, M. J. (2004). Feature-based recommendations for one-to-one marketing. Expert Systems with Applications, 26(4), 493–508. https://doi.org/10.1016/j.eswa.2003.10.008
Coyle, L., & Cunningham, P. (2004). Improving recommendation ranking by learning personal feature weights. Lecture Notes in Computer Science (Including Subseries Lecture Notes in Artificial Intelligence and Lecture Notes in Bioinformatics), 3155, 560–572. https://doi.org/10.1007/978-3-540-28631-8_41
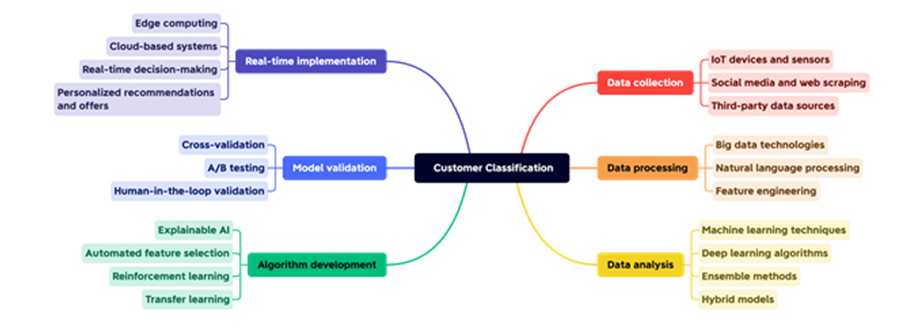
Downloads
Published
How to Cite
Issue
Section
License

This work is licensed under a Creative Commons Attribution-ShareAlike 4.0 International License.
All papers should be submitted electronically. All submitted manuscripts must be original work that is not under submission at another journal or under consideration for publication in another form, such as a monograph or chapter of a book. Authors of submitted papers are obligated not to submit their paper for publication elsewhere until an editorial decision is rendered on their submission. Further, authors of accepted papers are prohibited from publishing the results in other publications that appear before the paper is published in the Journal unless they receive approval for doing so from the Editor-In-Chief.
IJISAE open access articles are licensed under a Creative Commons Attribution-ShareAlike 4.0 International License. This license lets the audience to give appropriate credit, provide a link to the license, and indicate if changes were made and if they remix, transform, or build upon the material, they must distribute contributions under the same license as the original.