Machine Learning Approach for Early Disease Prediction and Risk Analysis
Keywords:
Precision in Disease Prediction, AI, MLAbstract
The optimistic future of AI integration in healthcare has become more imaginable in recent years because to AI's rapid development and the steady launch of AI research in the medical profession. The most significant promise for machine learning has been in applications like predicting how well a given drug will work. Clinicians have significant challenges when manually diagnosing abnormalities; this study aims to detect and predict people suffering from many diseases. Over time, both the accuracy and clarity of the pharmaceutical illness forecast have increased from the initial logistic regression to the machine learning prototype. This article takes a look at the many different machine learning frameworks available, as well as several common diseases and a brief explanation of the machine learning prediction methods used for each. Find the flaws in the current illness projection and estimate its growth going forward. Its overarching goal is to demonstrate ML's utility for disease prediction and to highlight the vital connection between ML and emerging medical technologies. The various feature extraction strategies available inside machine learning technologies may maintain their relevance in the field of medical study in the years to come.
Downloads
References
R. Manne, S.C. Kantheti, Application of artificial intelligence in healthcare: chances and challenges, Curr. J. Appl. Sci. Technol. 40 (6) (2021) 78–89, https:// doi.org/10.9734/cjast/2021/v40i631320.
M. Sivakami, P. Prabhu. Classification of algorithms supported factual knowledge recovery from cardiac data set, Int. J. Curr. Res. Rev. 13 (6) 161- 166. ISSN: 2231-2196 (Print) ISSN: 0975-5241 (Online).
M. Sivakami, P. Prabhu. A Comparative Review of Recent Data Mining Techniques for Prediction of Cardiovascular Disease from Electronic Health Records. In: Hemanth D., Shakya S., Baig Z. (eds) Intelligent Data Communication Technologies and Internet of Things. ICICI (2019).
P. Prabhu, S. Selvabharathi. Deep Belief Neural Network Model for Prediction of Diabetes Mellitus. In 2019 3rd International Conference on Imaging, Signal Processing and Communication, ICISPC (2019), 138–142, Institute of Electrical and Electronics Engineers Inc. ISBN:9781728136639. 2019.
N. Jothi, N.A. Rashid, W. Husain, Data mining in healthcare – A review, Procedia Comput. Sci. 72 (2015) 306–313.
H. Polat, H. Danaei Mehr, A. Cetin. Diagnosis of chronic kidney disease based on support vector machine by feature selection methods, J. Med. Syst. 41(4) (2017).
K.B. Wagholikar, V. Sundararajan, A.W. Deshpande, Modeling paradigms for medical diagnostic decision support: a survey and future directions, J. Med. Syst. 36 (5) (2012) 3029–3049.
E. Gürbüz, E. Kılıç, A new adaptive support vector machine for diagnosis of diseases, Expert Syst. 31 (5) (2014) 389–397.
M. Seera, C.P. Lim, A hybrid intelligent system for medical data classification, Expert Syst. Appl. 41 (5) (2014) 2239–2249.
Y. Kazemi, S.A. Mirroshandel, A novel method for predicting kidney stone type using ensemble learning, Artif. Intell. Med. 84 (2018) 117–126.
H. Barakat, P. Andrew, Bradley, H. Mohammed Nabil Barakat, Intelligible support vector machines for diagnosis of diabetes mellitus, IEEE Trans. Inf. Technol. Bio Med. J. 14 (4) (2009) 1–7.
R. Tina Patil, S.S. Sherekar, Performance analysis of Naive bayes and J48 classification algorithm for data classification, Int. J. Comput. Sci. Appl. 6 (2) (2013) 256–261.
Shruti Ratnakar, K. Rajeswari, Rose Jacob, Prediction of heart disease using genetic algorithm for selection of optimal reduced set of attributes, Int. J. Adv. Comput. Eng. Netw. 1 (2) (2013) 51–55.
S. Grampurohit, C. Sagarnal, Disease prediction using machine learning algorithms, Int. Conf. Emerg. Technol. (INCET) (2020) 1–7, https://doi. org/10.1109/INCET49848.2020.9154130.
R.J.P. Princy, S. Parthasarathy, P.S. Hency Jose, A. Raj Lakshminarayanan, S. Jeganathan, Prediction of Cardiac Disease using Supervised Machine Learning Algorithms, in: 2020 4th International Conference on Intelligent Computing and Control Systems (ICICCS), 2020, pp. 570–575, https://doi.org/10.1109/ICICCS48265.2020.9121169.
P. Deepika, S. Sasikala. Enhanced Model for Prediction and Classification of Cardiovascular Disease using Decision Tree with Particle Swarm Optimization, 2020 4th International Conference on Electronics, Communication and Aerospace Technology (ICECA), 2020, pp. 1068-1072, doi: 10.1109/ ICECA49313.2020.9297398.
Haq A U, Li J P, Memon M H, Nazir S and Sun R 2018 A hybrid intelligent system framework for the prediction of heart disease using machine learning algorithms; Mob. Inf. Syst. 2018: 21–22
Xiao Q, Luo J and Dai J 2019 Computational prediction of human disease- associated circRNAs based on manifold regularization learning framework; IEEE J. Biomed. Health. 23 2661–2669
Yang X, Lu R, Shao J, Tang X and Yang H 2019 An efficient and privacy-preserving disease risk prediction scheme for E-healthcare; IEEE Internet Things. 6 3284–3297
Va´squez-Morales G R, Martı´nez-Monterrubio S M, MorenoGer P and Recio-Garcı´a J A 2019 Explainable prediction of chronic renal disease in the colombian population using neural networks and case-based reasoning; IEEE Access. 7 152900–152910
Minhas S, Khanum A, Riaz F, Khan S A and Alvi A 2018 Predicting progression from mild cognitive impairment to Alzheimer’s disease using autoregressive modelling of longitudinal and multimodal biomarkers; IEEE J. Biomed. Health. (2020) 818–825.
Escudero J, Ifeachor E, Zajicek J P, Green C, Shearer J and Pearson S 2013 Machine learning-based method for personalized and cost-effective detection of Alzheimer’s disease; IEEE T Bio-Med Eng. 60 164–168.
Haq A Q, Li J P, Memon M H, Khan J, Malik A, Ahmad T, Ali A, Nazir S, Ahad I and Shahid M 2019 Feature selection based on L1-norm support vector machine and effective recognition system for Parkinson’s disease using voice recordings; IEEE Access 7: 37718–37734.
Sierra-Sosa D, Garcia-Zapirain M B, Castillo C, Oleagordia I, Nun˜o-Solinis R, Urtaran-Laresgoiti M, Elmaghraby A 2019 Scalable healthcare assessment for diabetic patients using deep learning on multiple GPUs; IEEE T. Ind. Inform. 15: 5682–5689.
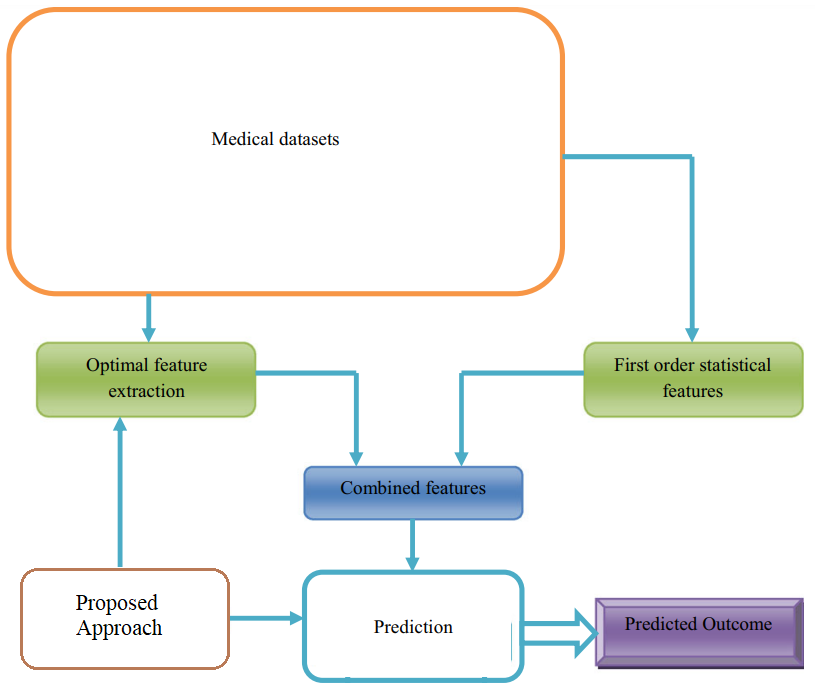
Downloads
Published
How to Cite
Issue
Section
License

This work is licensed under a Creative Commons Attribution-ShareAlike 4.0 International License.
All papers should be submitted electronically. All submitted manuscripts must be original work that is not under submission at another journal or under consideration for publication in another form, such as a monograph or chapter of a book. Authors of submitted papers are obligated not to submit their paper for publication elsewhere until an editorial decision is rendered on their submission. Further, authors of accepted papers are prohibited from publishing the results in other publications that appear before the paper is published in the Journal unless they receive approval for doing so from the Editor-In-Chief.
IJISAE open access articles are licensed under a Creative Commons Attribution-ShareAlike 4.0 International License. This license lets the audience to give appropriate credit, provide a link to the license, and indicate if changes were made and if they remix, transform, or build upon the material, they must distribute contributions under the same license as the original.